fastp: an ultra-fast all-in-one FASTQ preprocessor - PubMed
- ️Mon Jan 01 2018
fastp: an ultra-fast all-in-one FASTQ preprocessor
Shifu Chen et al. Bioinformatics. 2018.
Abstract
Motivation: Quality control and preprocessing of FASTQ files are essential to providing clean data for downstream analysis. Traditionally, a different tool is used for each operation, such as quality control, adapter trimming and quality filtering. These tools are often insufficiently fast as most are developed using high-level programming languages (e.g. Python and Java) and provide limited multi-threading support. Reading and loading data multiple times also renders preprocessing slow and I/O inefficient.
Results: We developed fastp as an ultra-fast FASTQ preprocessor with useful quality control and data-filtering features. It can perform quality control, adapter trimming, quality filtering, per-read quality pruning and many other operations with a single scan of the FASTQ data. This tool is developed in C++ and has multi-threading support. Based on our evaluation, fastp is 2-5 times faster than other FASTQ preprocessing tools such as Trimmomatic or Cutadapt despite performing far more operations than similar tools.
Availability and implementation: The open-source code and corresponding instructions are available at https://github.com/OpenGene/fastp.
Figures
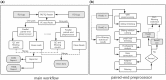
Workflow of fastp. (a) Main workflow of paired-end data processing, and (b) paired-end preprocessor of one read pair. In the main workflow, a pair of FASTQ files is loaded and packed, after which each read pair is processed individually in the paired-end preprocessor, described in (b)
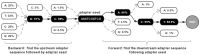
A demonstration of extending an adapter seed in both forward and backward directions. The found adapter is GCAAATCGATCGACT, with the first two bases (GC) as the upstream sequence, the central ten bases as the adapter seed, and the last three bases (ACT) as the downstream sequence
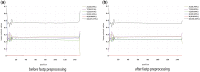
The base content ratio curves generated by fastp for one Illumina NextSeq FASTQ file. (a) Before fastp preprocessing, and (b) after fastp preprocessing. As depicted in (a), the G curve is abnormal and the G/C curves are separated. In (b), the G/C separation problem is eliminated
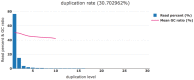
Duplication estimation. The read percentages and mean GC ratios of different duplication levels. The mean GC ratio curve is truncated since the reads with higher duplication level are too few to compute a stable mean value
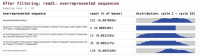
Overrepresented sequence analysis results. The right column shows the histogram of occurrence among all sequencing cycles
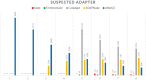
Result of adapter trimming performance evaluation. The X-axis is the number of allowed mismatches when searching for suspected adapter sequences, and the Y-axis is the count of suspected adapter sequences
Similar articles
-
Ultrafast one-pass FASTQ data preprocessing, quality control, and deduplication using fastp.
Chen S. Chen S. Imeta. 2023 May 8;2(2):e107. doi: 10.1002/imt2.107. eCollection 2023 May. Imeta. 2023. PMID: 38868435 Free PMC article.
-
FastqPuri: high-performance preprocessing of RNA-seq data.
Pérez-Rubio P, Lottaz C, Engelmann JC. Pérez-Rubio P, et al. BMC Bioinformatics. 2019 May 3;20(1):226. doi: 10.1186/s12859-019-2799-0. BMC Bioinformatics. 2019. PMID: 31053060 Free PMC article.
-
AfterQC: automatic filtering, trimming, error removing and quality control for fastq data.
Chen S, Huang T, Zhou Y, Han Y, Xu M, Gu J. Chen S, et al. BMC Bioinformatics. 2017 Mar 14;18(Suppl 3):80. doi: 10.1186/s12859-017-1469-3. BMC Bioinformatics. 2017. PMID: 28361673 Free PMC article.
-
fastQ_brew: module for analysis, preprocessing, and reformatting of FASTQ sequence data.
O'Halloran DM. O'Halloran DM. BMC Res Notes. 2017 Jul 12;10(1):275. doi: 10.1186/s13104-017-2616-7. BMC Res Notes. 2017. PMID: 28701181 Free PMC article.
-
MutScan: fast detection and visualization of target mutations by scanning FASTQ data.
Chen S, Huang T, Wen T, Li H, Xu M, Gu J. Chen S, et al. BMC Bioinformatics. 2018 Jan 22;19(1):16. doi: 10.1186/s12859-018-2024-6. BMC Bioinformatics. 2018. PMID: 29357822 Free PMC article.
Cited by
-
Microbial biomarker discovery in Parkinson's disease through a network-based approach.
Zhao Z, Chen J, Zhao D, Chen B, Wang Q, Li Y, Chen J, Bai C, Guo X, Hu N, Zhang B, Zhao R, Yuan J. Zhao Z, et al. NPJ Parkinsons Dis. 2024 Oct 26;10(1):203. doi: 10.1038/s41531-024-00802-2. NPJ Parkinsons Dis. 2024. PMID: 39461950 Free PMC article.
-
Zhou D, Yang K, Zhang Y, Liu C, He Y, Tan J, Ruan Z, Qiu R. Zhou D, et al. Front Microbiol. 2024 Oct 21;15:1461821. doi: 10.3389/fmicb.2024.1461821. eCollection 2024. Front Microbiol. 2024. PMID: 39498128 Free PMC article.
-
Li Y, Chen J, Jiang S, Yang Q, Yang L, Huang J, Shi J, Zhang Y, Lu Z, Zhou F. Li Y, et al. Biology (Basel). 2024 Oct 19;13(10):838. doi: 10.3390/biology13100838. Biology (Basel). 2024. PMID: 39452146 Free PMC article.
-
DeLTa-Seq: High-Throughput Targeted RNA-Seq of Rice Leaves Without RNA Purification.
Kashima M, Nomura Y, Nagano AJ. Kashima M, et al. Methods Mol Biol. 2025;2869:113-121. doi: 10.1007/978-1-0716-4204-7_12. Methods Mol Biol. 2025. PMID: 39499472
-
Dragon D, Jansen W, Dumont H, Wiggers L, Coupeau D, Saulmont M, Taminiau B, Muylkens B, Daube G. Dragon D, et al. Animals (Basel). 2024 Oct 21;14(20):3038. doi: 10.3390/ani14203038. Animals (Basel). 2024. PMID: 39457968 Free PMC article.
References
-
- Andrews S. (2010) A quality control tool for high throughput sequence data. http://www.bioinformatics.babraham.ac.uk/projects/fastqc/.
-
- Bianchi D.W., et al. (2015) Noninvasive prenatal testing and incidental detection of occult maternal malignancies. JAMA, 314, 162–169. - PubMed
-
- Brad Chapman R.K., et al. (2018) Validated, Scalable, Community Developed Variant Calling, RNA-Seq and Small RNA Analysis, https://github.com/chapmanb/bcbio-nextgen.
Publication types
MeSH terms
LinkOut - more resources
Full Text Sources
Other Literature Sources