Protein disorder prediction by condensed PSSM considering propensity for order or disorder - PubMed
- ️Sun Jan 01 2006
Comparative Study
Protein disorder prediction by condensed PSSM considering propensity for order or disorder
Chung-Tsai Su et al. BMC Bioinformatics. 2006.
Abstract
Background: More and more disordered regions have been discovered in protein sequences, and many of them are found to be functionally significant. Previous studies reveal that disordered regions of a protein can be predicted by its primary structure, the amino acid sequence. One observation that has been widely accepted is that ordered regions usually have compositional bias toward hydrophobic amino acids, and disordered regions are toward charged amino acids. Recent studies further show that employing evolutionary information such as position specific scoring matrices (PSSMs) improves the prediction accuracy of protein disorder. As more and more machine learning techniques have been introduced to protein disorder detection, extracting more useful features with biological insights attracts more attention.
Results: This paper first studies the effect of a condensed position specific scoring matrix with respect to physicochemical properties (PSSMP) on the prediction accuracy, where the PSSMP is derived by merging several amino acid columns of a PSSM belonging to a certain property into a single column. Next, we decompose each conventional physicochemical property of amino acids into two disjoint groups which have a propensity for order and disorder respectively, and show by experiments that some of the new properties perform better than their parent properties in predicting protein disorder. In order to get an effective and compact feature set on this problem, we propose a hybrid feature selection method that inherits the efficiency of uni-variant analysis and the effectiveness of the stepwise feature selection that explores combinations of multiple features. The experimental results show that the selected feature set improves the performance of a classifier built with Radial Basis Function Networks (RBFN) in comparison with the feature set constructed with PSSMs or PSSMPs that adopt simply the conventional physicochemical properties.
Conclusion: Distinguishing disordered regions from ordered regions in protein sequences facilitates the exploration of protein structures and functions. Results based on independent testing data reveal that the proposed predicting model DisPSSMP performs the best among several of the existing packages doing similar tasks, without either under-predicting or over-predicting the disordered regions. Furthermore, the selected properties are demonstrated to be useful in finding discriminating patterns for order/disorder classification.
Figures
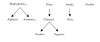
The relation of the selected properties after the first level of redundancy analysis.
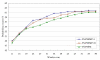
Comparison of using different feature sets on testing data R80.
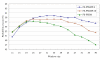
Comparison of using different feature sets on testing data U79 and P80.
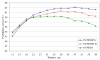
Comparison of using different feature sets on testing data R80, U79, and P80.
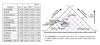
Comparing the performance of thirteen disorder prediction packages on testing data R80.
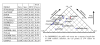
Comparing the performance of thirteen disorder prediction packages on testing data U79 and P80.
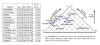
Comparing the performance of thirteen disorder prediction packages on testing data R80, U79, and P80.
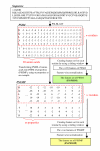
The procedure of preparing feature set for training and testing data.
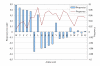
The propensity for order and the frequency of each amino acid in the training set.
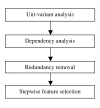
The flowchart of the hybrid feature selection mechanism.
Similar articles
-
Real value prediction of protein solvent accessibility using enhanced PSSM features.
Chang DT, Huang HY, Syu YT, Wu CP. Chang DT, et al. BMC Bioinformatics. 2008 Dec 12;9 Suppl 12(Suppl 12):S12. doi: 10.1186/1471-2105-9-S12-S12. BMC Bioinformatics. 2008. PMID: 19091011 Free PMC article.
-
PSSM-based prediction of DNA binding sites in proteins.
Ahmad S, Sarai A. Ahmad S, et al. BMC Bioinformatics. 2005 Feb 19;6:33. doi: 10.1186/1471-2105-6-33. BMC Bioinformatics. 2005. PMID: 15720719 Free PMC article.
-
iPDA: integrated protein disorder analyzer.
Su CT, Chen CY, Hsu CM. Su CT, et al. Nucleic Acids Res. 2007 Jul;35(Web Server issue):W465-72. doi: 10.1093/nar/gkm353. Epub 2007 Jun 6. Nucleic Acids Res. 2007. PMID: 17553839 Free PMC article.
-
Length-dependent prediction of protein intrinsic disorder.
Peng K, Radivojac P, Vucetic S, Dunker AK, Obradovic Z. Peng K, et al. BMC Bioinformatics. 2006 Apr 17;7:208. doi: 10.1186/1471-2105-7-208. BMC Bioinformatics. 2006. PMID: 16618368 Free PMC article.
-
Huang HL, Lin IC, Liou YF, Tsai CT, Hsu KT, Huang WL, Ho SJ, Ho SY. Huang HL, et al. BMC Bioinformatics. 2011 Feb 15;12 Suppl 1(Suppl 1):S47. doi: 10.1186/1471-2105-12-S1-S47. BMC Bioinformatics. 2011. PMID: 21342579 Free PMC article.
Cited by
-
The mysterious unfoldome: structureless, underappreciated, yet vital part of any given proteome.
Uversky VN. Uversky VN. J Biomed Biotechnol. 2010;2010:568068. doi: 10.1155/2010/568068. J Biomed Biotechnol. 2010. PMID: 20011072 Free PMC article. Review.
-
Library of disordered patterns in 3D protein structures.
Lobanov MY, Furletova EI, Bogatyreva NS, Roytberg MA, Galzitskaya OV. Lobanov MY, et al. PLoS Comput Biol. 2010 Oct 14;6(10):e1000958. doi: 10.1371/journal.pcbi.1000958. PLoS Comput Biol. 2010. PMID: 20976197 Free PMC article.
-
Understanding protein non-folding.
Uversky VN, Dunker AK. Uversky VN, et al. Biochim Biophys Acta. 2010 Jun;1804(6):1231-64. doi: 10.1016/j.bbapap.2010.01.017. Epub 2010 Feb 1. Biochim Biophys Acta. 2010. PMID: 20117254 Free PMC article. Review.
-
A comprehensive overview of computational protein disorder prediction methods.
Deng X, Eickholt J, Cheng J. Deng X, et al. Mol Biosyst. 2012 Jan;8(1):114-21. doi: 10.1039/c1mb05207a. Epub 2011 Aug 26. Mol Biosyst. 2012. PMID: 21874190 Free PMC article. Review.
-
Intrinsic disorder in protein interactions: insights from a comprehensive structural analysis.
Fong JH, Shoemaker BA, Garbuzynskiy SO, Lobanov MY, Galzitskaya OV, Panchenko AR. Fong JH, et al. PLoS Comput Biol. 2009 Mar;5(3):e1000316. doi: 10.1371/journal.pcbi.1000316. Epub 2009 Mar 13. PLoS Comput Biol. 2009. PMID: 19282967 Free PMC article.
References
-
- Dunker AK, Obradovic Z, Romero P, Kissinger C, Villafrance E. On the importance of being disordered. PDB Newsletter. 1997;81:3–5.
Publication types
MeSH terms
Substances
LinkOut - more resources
Full Text Sources
Research Materials