Improved disorder prediction by combination of orthogonal approaches - PubMed
- ️Invalid Date
Improved disorder prediction by combination of orthogonal approaches
Avner Schlessinger et al. PLoS One. 2009.
Abstract
Disordered proteins are highly abundant in regulatory processes such as transcription and cell-signaling. Different methods have been developed to predict protein disorder often focusing on different types of disordered regions. Here, we present MD, a novel META-Disorder prediction method that molds various sources of information predominantly obtained from orthogonal prediction methods, to significantly improve in performance over its constituents. In sustained cross-validation, MD not only outperforms its origins, but it also compares favorably to other state-of-the-art prediction methods in a variety of tests that we applied.
Availability: http://www.rostlab.org/services/md/
Conflict of interest statement
Competing Interests: The authors have declared that no competing interests exist.
Figures
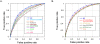
(A) The final method MD (blue filled diamonds), which uses neural networks to combine the output of other methods with sequence profiles and other sequence features, is significantly more accurate than the methods that it uses as input such as NORSnet (dark gray) and DISOPRED2 (dark green) as well as other popular predictors such as IUPred (purple), RONN (light green), VSL2B (pink) and VSL2 (light gray). Other VSL2 models resulted in AUCs ranging the values obtained by VSL2B (sequence based) and VSL2 (sequence+secondary structure+profiles). Note that the VSL methods were trained on DisProt. Since we tested that method on essentially the same data set without cross-validation, our results are likely to over-estimate the performance of the VSL methods. Using additional sequence features also improved over using only the output from other methods and profiles (light blue open diamonds). (B) We compared methods that would result from simply averaging over the output of original prediction methods (triangles). Most averages were better than the best original method (here Ucon, orange circle). Our final neural network-based method, MD, significantly outperformed others throughout almost the entire ROC-curve.
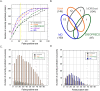
Data set: 205 DisProt proteins with at least one long (>30 residues) disordered region. (A) Our final method MD identified more true positives than the other methods at most of the false positive rates. (B) The results for false positive rates ≤0.25 (yellow bar) are presented in the Venn diagram. The numbers in parentheses correspond to the y-axis values of the points in the yellow column in graph (A). (C+D) This is the same data as for (A) except that we only considered the subset of proteins correctly predicted exclusively by the method shown, i.e., proteins with long disordered regions that no other method captured. Due to low counts, we smoothed values by running averages over three percentage points. In (C) the panels represent the proteins that are unique if MD is not included in the overlap calculation, whereas in (D) the panels represent the proteins that are unique when MD is included. The number of unique predictions is substantially smaller when including MD suggesting that MD not only yielded a good average but also captured all types of disorder.
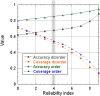
The normalized output of MD was converted into a reliability index that reflects the prediction strength. Different performance measures (Eqn. 1 and 2) were calculated and averaged over the six sets using the default cutoff defining positive prediction. Stronger predictions (higher reliability indices) were, on average, more accurate, e.g. if a user looked only at residues predicted at RI≥4, then she or he would expect to find about 52% of all disordered residues at that level, and over 68% of the residues identified at that level would be correct (marked by gray column). Note that one limitation of using DisProt is that the per-residue assignment of long unstructured regions can be inaccurate as some experimental techniques characterizing disorder may only capture global properties of the protein resulting mislabeling of the whole domain or protein as disordered.
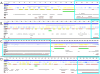
Predicting disorder and other sequence features using the MD server through the PredictProtein web-interface for protein sequence analysis (Methods) , . (A) NORSnet and Ucon predict some signal for the presence of disordered region in the C-terminal domain of T-cell surface glycoprotein CD3 gamma chain (DP00508) , while MD correctly predicts the whole domain to be disordered. (B) Similar results were obtained for the C-terminal domain of E. Coli Alkylmercury Lyase (DP00575) . (C) The signaling molecule Nogo-B (DP00524) contains disordered N-terminal, which was captured by MD. PROFsec and NORSnet predictions suggest that this region is long disordered loop. (D) The C-terminal domain of the ribosomal protein L5 (DP00579) is disordered. While PROFsec predicted this region to be helical (red rectangles), Ucon identified it as disordered, probably due to small number of internal contacts. MD agreed with Ucon output and correctly predicted this region to be disordered.
Similar articles
-
PROFbval: predict flexible and rigid residues in proteins.
Schlessinger A, Yachdav G, Rost B. Schlessinger A, et al. Bioinformatics. 2006 Apr 1;22(7):891-3. doi: 10.1093/bioinformatics/btl032. Epub 2006 Feb 2. Bioinformatics. 2006. PMID: 16455751
-
Liu Y, Wang X, Liu B. Liu Y, et al. Brief Bioinform. 2019 Jan 18;20(1):330-346. doi: 10.1093/bib/bbx126. Brief Bioinform. 2019. PMID: 30657889 Review.
-
Deng X, Gumm J, Karki S, Eickholt J, Cheng J. Deng X, et al. Int J Mol Sci. 2015 Jul 7;16(7):15384-404. doi: 10.3390/ijms160715384. Int J Mol Sci. 2015. PMID: 26198229 Free PMC article.
-
Wang Z, Yang Q, Li T, Cong P. Wang Z, et al. PLoS One. 2015 Jun 19;10(6):e0128334. doi: 10.1371/journal.pone.0128334. eCollection 2015. PLoS One. 2015. PMID: 26090958 Free PMC article.
-
Bioinformatical approaches to characterize intrinsically disordered/unstructured proteins.
Dosztányi Z, Mészáros B, Simon I. Dosztányi Z, et al. Brief Bioinform. 2010 Mar;11(2):225-43. doi: 10.1093/bib/bbp061. Epub 2009 Dec 10. Brief Bioinform. 2010. PMID: 20007729 Review.
Cited by
-
Deep learning methods in protein structure prediction.
Torrisi M, Pollastri G, Le Q. Torrisi M, et al. Comput Struct Biotechnol J. 2020 Jan 22;18:1301-1310. doi: 10.1016/j.csbj.2019.12.011. eCollection 2020. Comput Struct Biotechnol J. 2020. PMID: 32612753 Free PMC article. Review.
-
Puton T, Kozlowski LP, Rother KM, Bujnicki JM. Puton T, et al. Nucleic Acids Res. 2013 Apr;41(7):4307-23. doi: 10.1093/nar/gkt101. Epub 2013 Feb 21. Nucleic Acids Res. 2013. PMID: 23435231 Free PMC article.
-
MetaDisorder: a meta-server for the prediction of intrinsic disorder in proteins.
Kozlowski LP, Bujnicki JM. Kozlowski LP, et al. BMC Bioinformatics. 2012 May 24;13:111. doi: 10.1186/1471-2105-13-111. BMC Bioinformatics. 2012. PMID: 22624656 Free PMC article.
-
A novel approach for predicting disordered regions in a protein sequence.
Li M, Cho SB, Ryu KH. Li M, et al. Osong Public Health Res Perspect. 2014 Aug;5(4):211-8. doi: 10.1016/j.phrp.2014.06.006. Epub 2014 Jul 1. Osong Public Health Res Perspect. 2014. PMID: 25379372 Free PMC article.
-
Predict impact of single amino acid change upon protein structure.
Schaefer C, Rost B. Schaefer C, et al. BMC Genomics. 2012 Jun 18;13 Suppl 4(Suppl 4):S4. doi: 10.1186/1471-2164-13-S4-S4. BMC Genomics. 2012. PMID: 22759652 Free PMC article.
References
-
- Dyson HJ, Wright PE. Intrinsically unstructured proteins and their functions. Nat Rev Mol Cell Biol. 2005;6:197–208. - PubMed
-
- Dunker AK, Obradovic Z. The protein trinity-linking function and disorder. Nature Biotechnology. 2001;19:805–806. - PubMed
-
- Uversky VN, Gillespie JR, Fink AL. Why are “natively unfolded” proteins unstructured under physiologic conditions? Proteins: Structure, Function, and Genetics. 2000;41:415–427. - PubMed
-
- Bracken C, Iakoucheva LM, Romero PR, Dunker AK. Combining prediction, computation and experiment for the characterization of protein disorder. Curr Opin Struct Biol. 2004;14:570–576. - PubMed
Publication types
MeSH terms
Substances
LinkOut - more resources
Full Text Sources