Predicting continuous local structure and the effect of its substitution for secondary structure in fragment-free protein structure prediction - PubMed
- ️Thu Jan 01 2009
Predicting continuous local structure and the effect of its substitution for secondary structure in fragment-free protein structure prediction
Eshel Faraggi et al. Structure. 2009.
Abstract
Local structures predicted from protein sequences are used extensively in every aspect of modeling and prediction of protein structure and function. For more than 50 years, they have been predicted at a low-resolution coarse-grained level (e.g., three-state secondary structure). Here, we combine a two-state classifier with real-value predictor to predict local structure in continuous representation by backbone torsion angles. The accuracy of the angles predicted by this approach is close to that derived from NMR chemical shifts. Their substitution for predicted secondary structure as restraints for ab initio structure prediction doubles the success rate. This result demonstrates the potential of predicted local structure for fragment-free tertiary-structure prediction. It further implies potentially significant benefits from using predicted real-valued torsion angles as a replacement for or supplement to the secondary-structure prediction tools used almost exclusively in many computational methods ranging from sequence alignment to function prediction.
Figures
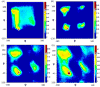
The Ramachandran plot of 10-fold cross-validated predictions of 2640 proteins by a previous real-value prediction method called Real-SPINE 3 (A), by combining a two-state classifier with real value prediction, SPINE X (B), post-refinement, SPINE XI (C), and native dihedral angles (D). The ψ axis is shifted for a clearer view of the population separation. No shift was done on the ϕ angles.
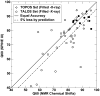
The accuracy of torsion angles obtained from NMR chemical shifts by programs TOPOS (11 proteins) and TALOS (37 proteins) is compared to that given by SPINEXI. Accuracy is measured by Q60°, the fraction of residues for which both ϕ and ψ angles are less than 60° away from their respective native values. SPINE XI makes equally or more accurate prediction for 20 proteins (42%). NMR-derived angles are only slightly more accurate than SPINE XI for an additional 10 proteins (less than 5% difference) (dashed line).
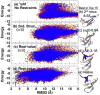
The effects of various restraints on conformational sampling of 1shf. The energy of all sampled conformations as a function of their RMSD values from the native structure. From top to bottom, conformations are sampled without any restraints (a), with predicted secondary-structure restraints (i.e. restraining around the ideal angles of predicted helical and/or strand residues) (b), with restraints around predicted real-value torsion angles of predicted helical and/or strand residues only (c), and with restraints around predicted torsion angles for all residues (d). The corresponding best structures in top 15 for (b) to (d) are shown in the right panel and the native structure is shown in (e).
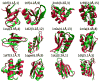
Comparison between predicted structure (best in top 15, in Green) and native structure (in Red) for 12 proteins as labeled, along with their ranks.
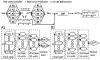
Network architecture for real-value prediction of backbone torsion angles (SPINE XI). Two sets of neural networks are constructed for predicting angle peaks (Peak I or Peak II) by a two-state classifier (A1–A5) and the deviation from the peak by a real-value predictor (B1–B5). Both predictions result from a consensus of five independent predictions. Each predictor (A1,..A5,B1,…B5) has two hidden layers. Angles predicted by neural networks are further refined by the conditional random field (CRF) model.
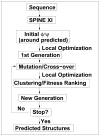
The flow chart for the method used for fragment-free protein-tertiary-structure prediction. Details for the first part of the method, obtaining SPINE XI, are given in Fig. 5.
Similar articles
-
Song J, Tan H, Wang M, Webb GI, Akutsu T. Song J, et al. PLoS One. 2012;7(2):e30361. doi: 10.1371/journal.pone.0030361. Epub 2012 Feb 2. PLoS One. 2012. PMID: 22319565 Free PMC article.
-
Shen Y, Bax A. Shen Y, et al. J Biomol NMR. 2013 Jul;56(3):227-41. doi: 10.1007/s10858-013-9741-y. Epub 2013 Jun 2. J Biomol NMR. 2013. PMID: 23728592 Free PMC article.
-
Helles G, Fonseca R. Helles G, et al. BMC Bioinformatics. 2009 Oct 16;10:338. doi: 10.1186/1471-2105-10-338. BMC Bioinformatics. 2009. PMID: 19835576 Free PMC article.
-
Computational methods for protein secondary structure prediction using multiple sequence alignments.
Heringa J. Heringa J. Curr Protein Pept Sci. 2000 Nov;1(3):273-301. doi: 10.2174/1389203003381324. Curr Protein Pept Sci. 2000. PMID: 12369910 Review.
-
Functional inferences from blind ab initio protein structure predictions.
Bonneau R, Tsai J, Ruczinski I, Baker D. Bonneau R, et al. J Struct Biol. 2001 May-Jun;134(2-3):186-90. doi: 10.1006/jsbi.2000.4370. J Struct Biol. 2001. PMID: 11551178 Review.
Cited by
-
Inadequate Reference Datasets Biased toward Short Non-epitopes Confound B-cell Epitope Prediction.
Rahman KhS, Chowdhury EU, Sachse K, Kaltenboeck B. Rahman KhS, et al. J Biol Chem. 2016 Jul 8;291(28):14585-99. doi: 10.1074/jbc.M116.729020. Epub 2016 May 9. J Biol Chem. 2016. PMID: 27189949 Free PMC article.
-
Lin H, Hargreaves KA, Li R, Reiter JL, Wang Y, Mort M, Cooper DN, Zhou Y, Zhang C, Eadon MT, Dolan ME, Ipe J, Skaar TC, Liu Y. Lin H, et al. Genome Biol. 2019 Nov 28;20(1):254. doi: 10.1186/s13059-019-1847-4. Genome Biol. 2019. PMID: 31779641 Free PMC article.
-
Entropy, Fluctuations, and Disordered Proteins.
Faraggi E, Dunker AK, Jernigan RL, Kloczkowski A. Faraggi E, et al. Entropy (Basel). 2019 Aug;21(8):764. doi: 10.3390/e21080764. Epub 2019 Aug 6. Entropy (Basel). 2019. PMID: 32336912 Free PMC article.
-
Deng X, Cheng J. Deng X, et al. BMC Bioinformatics. 2014 Jul 25;15(1):252. doi: 10.1186/1471-2105-15-252. BMC Bioinformatics. 2014. PMID: 25062980 Free PMC article.
-
López Y, Sharma A, Dehzangi A, Lal SP, Taherzadeh G, Sattar A, Tsunoda T. López Y, et al. BMC Genomics. 2018 Jan 19;19(Suppl 1):923. doi: 10.1186/s12864-017-4336-8. BMC Genomics. 2018. PMID: 29363424 Free PMC article.
References
-
- Baum LE, Petrie T. Statistical inference for probablistic functions of finite state Markov chains. Annals of Mathematical Statistics. 1966;37:1554–1563.
-
- Betancourt MR. Efficient Monte Carlo trial moves for polypeptide simulations. J Chem Phys. 2005;123:174905. - PubMed
Publication types
MeSH terms
Substances
Grants and funding
LinkOut - more resources
Full Text Sources