Automated network analysis identifies core pathways in glioblastoma - PubMed
- ️Fri Jan 01 2010
Automated network analysis identifies core pathways in glioblastoma
Ethan Cerami et al. PLoS One. 2010.
Abstract
Background: Glioblastoma multiforme (GBM) is the most common and aggressive type of brain tumor in humans and the first cancer with comprehensive genomic profiles mapped by The Cancer Genome Atlas (TCGA) project. A central challenge in large-scale genome projects, such as the TCGA GBM project, is the ability to distinguish cancer-causing "driver" mutations from passively selected "passenger" mutations.
Principal findings: In contrast to a purely frequency based approach to identifying driver mutations in cancer, we propose an automated network-based approach for identifying candidate oncogenic processes and driver genes. The approach is based on the hypothesis that cellular networks contain functional modules, and that tumors target specific modules critical to their growth. Key elements in the approach include combined analysis of sequence mutations and DNA copy number alterations; use of a unified molecular interaction network consisting of both protein-protein interactions and signaling pathways; and identification and statistical assessment of network modules, i.e. cohesive groups of genes of interest with a higher density of interactions within groups than between groups.
Conclusions: We confirm and extend the observation that GBM alterations tend to occur within specific functional modules, in spite of considerable patient-to-patient variation, and that two of the largest modules involve signaling via p53, Rb, PI3K and receptor protein kinases. We also identify new candidate drivers in GBM, including AGAP2/CENTG1, a putative oncogene and an activator of the PI3K pathway; and, three additional significantly altered modules, including one involved in microtubule organization. To facilitate the application of our network-based approach to additional cancer types, we make the method freely available as part of a software tool called NetBox.
Conflict of interest statement
Competing Interests: The authors have declared that no competing interests exist.
Figures
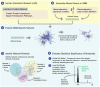
We began by creating a literature curated Human Interaction Network (HIN) derived from protein-protein interactions and signaling pathways (A), and assembling genomic alterations in GBM (B). We then extracted a GBM-specific network of altered genes (C), which was then partitioned into network modules (D). We assessed the level of connectivity seen within the GBM network by using (E1) a global null model to compare the size of the largest component in the observed network v. networks arising from randomly selected gene sets; and (E2) a local null model to compare network modularity of the observed network to locally rewired networks.
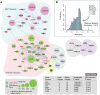
(A) Modules are densely connected sets of altered genes that may reflect oncogenic processes. A total of 10 modules were identified, the largest of which are shown. Linker genes, indicated in red, are not altered in GBM, but are statistically enriched for connections to GBM-altered genes. (B) The observed modularity of the GBM network (0.519) is compared with 1000 randomly rewired networks (average 0.296, standard deviation 0.058). This results in a z-score, or scaled modularity score, of 3.84.
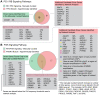
The original pathway analysis of TCGA glioblastoma datasets was derived by mapping observed gene alterations onto a manually curated GBM-specific network, based on the glioblastoma literature. This non-algorithmic analysis identified driver alterations in the p53, RB and PI3K pathways. Our automated network analysis approach is in close agreement with these results (top: P53/Rb; bottom: PI3K). The one main exception is that network analysis does not identify NF1 as a participant in the PI3K module. Additional candidate driver genes identified by network analysis, including AGAP2, are identified and annotated on the right. Percentage values after each newly identified candidate driver indicate percent of cases with genetic alterations (sequence mutations, homozygous deletions, or multi-copy amplifications) across the 84 TCGA GBM cases analyzed.
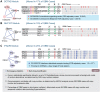
Each of the altered modules is implicated by homozygous deletions or multi-copy amplifications across the 84 analyzed GBM cases. Each module is annotated with Gene Ontology enrichment, chromosome location, statistical significance of copy number alteration against a background model of random aberrations, as determined by RAE copy number analysis; assessment of correlation between copy number and mRNA expression, and genomic signature across 84 GBM cases.
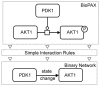
To integrate complex signaling pathway data into our Human Interaction Network (HIN), we have developed a set of rules for mapping subgraphs of biochemical networks to binary interactions. An example rule for mapping state changes, such as phosphorylation events, is shown.
Similar articles
-
Gu Y, Wang H, Qin Y, Zhang Y, Zhao W, Qi L, Zhang Y, Wang C, Guo Z. Gu Y, et al. Mol Biosyst. 2013 Mar;9(3):467-77. doi: 10.1039/c2mb25528f. Epub 2013 Jan 23. Mol Biosyst. 2013. PMID: 23344900
-
Zhang J, Zhang S, Wang Y, Zhang XS. Zhang J, et al. BMC Syst Biol. 2013;7 Suppl 2(Suppl 2):S4. doi: 10.1186/1752-0509-7-S2-S4. Epub 2013 Oct 14. BMC Syst Biol. 2013. PMID: 24565034 Free PMC article.
-
Mutual exclusivity analysis identifies oncogenic network modules.
Ciriello G, Cerami E, Sander C, Schultz N. Ciriello G, et al. Genome Res. 2012 Feb;22(2):398-406. doi: 10.1101/gr.125567.111. Epub 2011 Sep 9. Genome Res. 2012. PMID: 21908773 Free PMC article.
-
Current understanding of the role and targeting of tumor suppressor p53 in glioblastoma multiforme.
England B, Huang T, Karsy M. England B, et al. Tumour Biol. 2013 Aug;34(4):2063-74. doi: 10.1007/s13277-013-0871-3. Epub 2013 Jun 5. Tumour Biol. 2013. PMID: 23737287 Review.
-
Venkatesan S, Lamfers ML, Dirven CM, Leenstra S. Venkatesan S, et al. CNS Oncol. 2016;5(2):77-90. doi: 10.2217/cns-2015-0005. Epub 2016 Mar 17. CNS Oncol. 2016. PMID: 26986934 Free PMC article. Review.
Cited by
-
EARN: an ensemble machine learning algorithm to predict driver genes in metastatic breast cancer.
Mirsadeghi L, Haji Hosseini R, Banaei-Moghaddam AM, Kavousi K. Mirsadeghi L, et al. BMC Med Genomics. 2021 May 7;14(1):122. doi: 10.1186/s12920-021-00974-3. BMC Med Genomics. 2021. PMID: 33962648 Free PMC article.
-
Zhang L, Yuan Y, Lu KH, Zhang L. Zhang L, et al. BMC Bioinformatics. 2016 May 26;17(1):222. doi: 10.1186/s12859-016-1085-7. BMC Bioinformatics. 2016. PMID: 27230211 Free PMC article.
-
TRIM3, a tumor suppressor linked to regulation of p21(Waf1/Cip1.).
Liu Y, Raheja R, Yeh N, Ciznadija D, Pedraza AM, Ozawa T, Hukkelhoven E, Erdjument-Bromage H, Tempst P, Gauthier NP, Brennan C, Holland EC, Koff A. Liu Y, et al. Oncogene. 2014 Jan 16;33(3):308-15. doi: 10.1038/onc.2012.596. Epub 2013 Jan 14. Oncogene. 2014. PMID: 23318451 Free PMC article.
-
Identification of aberrant pathways and network activities from high-throughput data.
Wang J, Zhang Y, Marian C, Ressom HW. Wang J, et al. Brief Bioinform. 2012 Jul;13(4):406-19. doi: 10.1093/bib/bbs001. Epub 2012 Jan 27. Brief Bioinform. 2012. PMID: 22287794 Free PMC article. Review.
-
Studying a complex tumor: potential and pitfalls.
Zheng S, Chheda MG, Verhaak RG. Zheng S, et al. Cancer J. 2012 Jan-Feb;18(1):107-14. doi: 10.1097/PPO.0b013e3182431c57. Cancer J. 2012. PMID: 22290264 Free PMC article. Review.
References
-
- Furnari FB, Fenton T, Bachoo RM, Mukasa A, Stommel JM, et al. Malignant astrocytic glioma: genetics, biology, and paths to treatment. Genes Dev. 2007;21:2683–2710. - PubMed
Publication types
MeSH terms
Substances
Grants and funding
LinkOut - more resources
Full Text Sources
Other Literature Sources
Medical
Research Materials
Miscellaneous