SPA: Short peptide analyzer of intrinsic disorder status of short peptides - PubMed
SPA: Short peptide analyzer of intrinsic disorder status of short peptides
Bin Xue et al. Genes Cells. 2010 Jun.
Abstract
Disorder prediction for short peptides is important and difficult. All modern predictors have to be optimized on a preselected dataset prior to prediction. In the succeeding prediction process, the predictor works on a query sequence or its short segment. For implementing the prediction smoothly and obtaining sound prediction results, a specific length of the sequence or segment is usually required. The need of the preselected dataset in the optimization process and the length limitation in the prediction process restrict predictors' performance. To minimize the influence of these limitations, we developed a method for the prediction of intrinsic disorder in short peptides based on large dataset sampling and statistics. As evident from the data analysis, this method provides more reliable prediction of the intrinsic disorder status of short peptides.
Figures
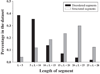
Length distribution of short disordered and short ordered segments from DSP and OSP.
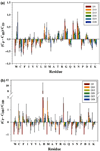
Relative composition profile of DSP segments vs FDD (a) and that of OSP segments vs fully ordered dataset (FOD) (b). On x-axis, amino acids are arranged in ascending disorder tendency. CP is the absolute composition of one amino acid in the query dataset; CID is the absolute composition of the same amino acid in FDD; COD is the same amino acid composition in FOD. Error bars are from 200 times of bootstrapping sampling. ‘D’ indicates subsets from DSP while ‘O’ is for subsets from OSP. D5 includes all the segments with segment length less than 5; D10 is for segments longer than or equal to 5 but <10; D15 corresponds to segments with 10 ≤ L < 15; D20 is 15 ≤ L < 20; D25 is 20 ≤ L < 25; D28 is 25 ≤ L ≤ 28. The same nomenclature is applied to subsets obtained from OSP.
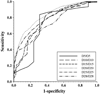
Receiver operating characteristic (ROC) curve of SPA in DSP/OSP datasets. These two datasets are furthermore grouped into subsets according to the length of segments in them. ‘D’ indicates subsets from DSP while ‘O’ is for subsets from OSP. D5 includes all the segments with segment length less than 5; D10 is for segments longer than or equal to five but <10; D15 corresponds to segments with 10 ≤ L < 15; D20 is 15 ≤ L < 20; D25 is 20 ≤ L < 25; D28 is 25 ≤ L ≤ 28. The same nomenclature is applied to subsets obtained from OSP. Each pair of subsets with the same range of length, originated from DSP and OSP, respectively, are put together to calculate the ROC curve for segments of that length.
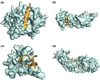
3D Structure of molecular recognition features (MoRFs) with their substrates. (a) (PDBid:2NM1) and (b) (PDBid:2AUC) are alpha-MoRFs. (a) is predicted to be structured while (b) is disordered. (c) (PDBid:1LXH) and (d) (PDNid: 1PJM) are coil-MoRFs with (c) structured and (d) disordered.
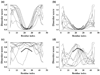
Application of SPA on two peptides P1 (PFVVSDIAFMGLFYD) and P2 (PLSHGSVVYPRSSLG). P1 is experimentally identified as ordered while P2 is disordered. All the slim curves are PONDR-VLXT predictions for the combined peptides by inserting the query peptides into disordered and ordered segments selected from fully disordered segments (FDS) and fully ordered segments (FOS). The large connected dots are predictions and error bars from SPA. (a) Predictions of 10 randomly selected combined peptides by embedding P1 on disordered protein segments from FDS. (b) Predictions of 10 randomly combined peptides by implanting P1 into ordered segments of FOS. (c) Predictions for peptides generated by inserting P2 into 10 segments used in (a). (d) Combining P2 onto 10 segments shown in (b).
Similar articles
-
Structural prediction of peptides binding to MHC class I molecules.
Bui HH, Schiewe AJ, von Grafenstein H, Haworth IS. Bui HH, et al. Proteins. 2006 Apr 1;63(1):43-52. doi: 10.1002/prot.20870. Proteins. 2006. PMID: 16447245
-
Zhao Z, Peng Z, Yang J. Zhao Z, et al. J Chem Inf Model. 2018 Jul 23;58(7):1459-1468. doi: 10.1021/acs.jcim.8b00019. Epub 2018 Jun 25. J Chem Inf Model. 2018. PMID: 29895149
-
Prediction of the protein structural class by specific peptide frequencies.
Costantini S, Facchiano AM. Costantini S, et al. Biochimie. 2009 Feb;91(2):226-9. doi: 10.1016/j.biochi.2008.09.005. Epub 2008 Oct 10. Biochimie. 2009. PMID: 18957316
-
Atomic displacement parameters in structural biology.
Carugo O. Carugo O. Amino Acids. 2018 Jul;50(7):775-786. doi: 10.1007/s00726-018-2574-y. Epub 2018 May 11. Amino Acids. 2018. PMID: 29752562 Review.
-
Accuracy of protein-level disorder predictions.
Katuwawala A, Oldfield CJ, Kurgan L. Katuwawala A, et al. Brief Bioinform. 2020 Sep 25;21(5):1509-1522. doi: 10.1093/bib/bbz100. Brief Bioinform. 2020. PMID: 31616935 Review.
Cited by
-
An Overview of Predictors for Intrinsically Disordered Proteins over 2010-2014.
Li J, Feng Y, Wang X, Li J, Liu W, Rong L, Bao J. Li J, et al. Int J Mol Sci. 2015 Sep 29;16(10):23446-62. doi: 10.3390/ijms161023446. Int J Mol Sci. 2015. PMID: 26426014 Free PMC article. Review.
-
Yang X, Guo Y, Luo J, Pu X, Li M. Yang X, et al. PLoS One. 2013 Dec 31;8(12):e84439. doi: 10.1371/journal.pone.0084439. eCollection 2013. PLoS One. 2013. PMID: 24391954 Free PMC article.
References
-
- Balla S, Thapar V, Verma S, Luong T, Faghri T, Huang CH, Rajasekaran S, del Campo JJ, Shinn JH, Mohler WA, Maciejewski MW, Gryk MR, Piccirillo B, Schiller SR, Schiller MR. Minimotif Miner: a tool for investigating protein function. Nat. Methods. 2006;3:175–177. - PubMed
-
- Burley SK, Petsko GA. Aromatic-aromatic interaction: a mechanism of protein structure stabilization. Science. 1985;229:23–28. - PubMed
-
- Dunker AK, Brown CJ, Lawson JD, Iakoucheva LM, Obradovic Z. Intrinsic disorder and protein function. Biochemistry. 2002a;41:6573–6582. - PubMed
Publication types
MeSH terms
Substances
Grants and funding
LinkOut - more resources
Full Text Sources
Research Materials