MCMC estimation of Markov models for ion channels - PubMed
- ️Sat Jan 01 2011
MCMC estimation of Markov models for ion channels
Ivo Siekmann et al. Biophys J. 2011.
Abstract
Ion channels are characterized by inherently stochastic behavior which can be represented by continuous-time Markov models (CTMM). Although methods for collecting data from single ion channels are available, translating a time series of open and closed channels to a CTMM remains a challenge. Bayesian statistics combined with Markov chain Monte Carlo (MCMC) sampling provide means for estimating the rate constants of a CTMM directly from single channel data. In this article, different approaches for the MCMC sampling of Markov models are combined. This method, new to our knowledge, detects overparameterizations and gives more accurate results than existing MCMC methods. It shows similar performance as QuB-MIL, which indicates that it also compares well with maximum likelihood estimators. Data collected from an inositol trisphosphate receptor is used to demonstrate how the best model for a given data set can be found in practice.
Copyright © 2011 Biophysical Society. Published by Elsevier Inc. All rights reserved.
Figures
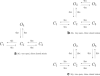
Examples for Markov models.
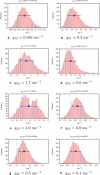
Histograms for the algorithm MHG after 50,000 iterations and a burn-in time of 10,000 iterations. MHG was run on a test data set consisting of 40,000 data points generated from model M2 (see Table 1, M2 columns). (Vertical dotted lines) True values of the rate constants. (Asterisks) Means of the histograms and (Arrows) standard deviations.
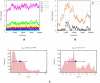
Model M2 is fitted to test data of 40,000 data points generated from the simpler model M1 using the MH sampler. The convergence plots (a and b) show that the rates connecting to the extra state O5 wander around whereas the others tend to the correct values (compare this to Table 1, M1 columns). Histograms for q45 and q54 are shown in panel c. The wide-spread multimodal posterior distributions for both rate constants clearly indicate that the state O5 is not supported by the data.
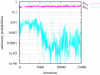
Model M3 is fitted to test data (40,000 data points) generated from the simpler model M1 using the MH algorithm. The stationary probability of the additional open state O5 quickly tends to zero. This suggests that the sampler detects when a model is too complex for representing a given data set and reacts by switching off transitions to the additional state.
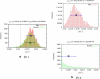
Rosales' Gibbs sampler and the MH algorithm are compared for a test data set of 100,000 data points. As representative examples, we show histograms for components ρ11 and ρ15 of the matrix exponential Aτ (plotted in red) of model M2 (see Fig. 1) with results from Rosales' Gibbs sampler (plotted in green). (Vertical dotted line) Exact value of the matrix component. Both methods give very similar results for the diagonal of Aτ, as can be seen in panel a, for example. Mean and standard deviations for MH algorithm (purple) and Rosales' Gibbs sampler (green) are similar. The histograms for the off-diagonal elements found by Rosales' method are distributed over a wide range and are therefore much less accurate than the estimates found by MH; compare the two fits for ρ15 in panel b.
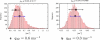
Selected histograms for a MHG run (50,000 iterations) and results of QUB-MIL for a test data set of 40,000 data points. (Dotted vertical line) The true value of a rate constant. (Asterisk) The maximum likelihood estimator found by QuB-MIL. (Upper arrows) Standard deviation found by QuB-MIL; (lower arrows) mean and standard deviations found by MHG. The estimates with relative errors are q^32MIL=0.522(−13.0%),q^32MHG=0.606(+1%) and q^45MIL=0.365(+21.5%),q^45MHG=0.359(+19.7%).
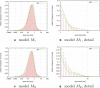
Open histogram for a data set of 1,400,000 data points which was collected from an IP3-type I receptor at a calcium concentration of 200 nmol/L (24). This is shown together with the superimposed open time distributions determined by fits to two different models, one with one open state (M1) and one with two open states (M2) (see Fig. 1, a and b). Although the histogram has only one distinguished peak indicating that one open state is sufficient, it shows that the open time histogram is better approximated for long open events by the model with two open states.
Similar articles
-
MCMC can detect nonidentifiable models.
Siekmann I, Sneyd J, Crampin EJ. Siekmann I, et al. Biophys J. 2012 Dec 5;103(11):2275-86. doi: 10.1016/j.bpj.2012.10.024. Biophys J. 2012. PMID: 23283226 Free PMC article.
-
Bayesian approaches for mechanistic ion channel modeling.
Calderhead B, Epstein M, Sivilotti L, Girolami M. Calderhead B, et al. Methods Mol Biol. 2013;1021:247-72. doi: 10.1007/978-1-62703-450-0_13. Methods Mol Biol. 2013. PMID: 23715989
-
A kinetic model of the inositol trisphosphate receptor based on single-channel data.
Gin E, Falcke M, Wagner LE 2nd, Yule DI, Sneyd J. Gin E, et al. Biophys J. 2009 May 20;96(10):4053-62. doi: 10.1016/j.bpj.2008.12.3964. Biophys J. 2009. PMID: 19450477 Free PMC article.
-
MCMC for hidden Markov models incorporating aggregation of states and filtering.
Rosales RA. Rosales RA. Bull Math Biol. 2004 Sep;66(5):1173-99. doi: 10.1016/j.bulm.2003.12.001. Bull Math Biol. 2004. PMID: 15294422
-
Choi K. Choi K. Transl Clin Pharmacol. 2023 Jun;31(2):69-84. doi: 10.12793/tcp.2023.31.e9. Epub 2023 Jun 26. Transl Clin Pharmacol. 2023. PMID: 37440780 Free PMC article. Review.
Cited by
-
Jæger KH, Edwards AG, Giles WR, Tveito A. Jæger KH, et al. PLoS Comput Biol. 2021 Aug 12;17(8):e1009233. doi: 10.1371/journal.pcbi.1009233. eCollection 2021 Aug. PLoS Comput Biol. 2021. PMID: 34383746 Free PMC article.
-
Moffett AS, Cui G, Thomas PJ, Hunt WD, McCarty NA, Westafer RS, Eckford AW. Moffett AS, et al. Biophys Rep (N Y). 2022 Oct 19;2(4):100083. doi: 10.1016/j.bpr.2022.100083. eCollection 2022 Dec 14. Biophys Rep (N Y). 2022. PMID: 36425670 Free PMC article.
-
Potassium-selective block of barium permeation through single KcsA channels.
Piasta KN, Theobald DL, Miller C. Piasta KN, et al. J Gen Physiol. 2011 Oct;138(4):421-36. doi: 10.1085/jgp.201110684. Epub 2011 Sep 12. J Gen Physiol. 2011. PMID: 21911483 Free PMC article.
-
Gratz D, Winkle AJ, Weinberg SH, Hund TJ. Gratz D, et al. Cells. 2021 Jun 16;10(6):1516. doi: 10.3390/cells10061516. Cells. 2021. PMID: 34208565 Free PMC article.
-
Tveito A, Lines GT, Edwards AG, McCulloch A. Tveito A, et al. Math Biosci. 2016 Jul;277:126-35. doi: 10.1016/j.mbs.2016.04.011. Epub 2016 May 3. Math Biosci. 2016. PMID: 27154008 Free PMC article.
References
-
- Neher E., Sakmann B. Single-channel currents recorded from membrane of denervated frog muscle fibers. Nature. 1976;260:799–802. - PubMed
-
- Colquhoun D., Hawkes A.G. On the stochastic properties of single ion channels. Proc. R. Soc. Lond. B Biol. Sci. 1981;211:205–235. - PubMed
-
- Qin F., Auerbach A., Sachs F. Idealization of single-channel currents using the segmental K-means method. Biophys. J. 1996;70:MP432. - PubMed
-
- Colquhoun D., Hawkes A.G., Srodzinski K. Joint distributions of apparent open and shut times of single-ion channels and maximum likelihood fitting of mechanisms. Philos. Trans. R. Soc. Lond. A. 1996;354:2555–2590.
Publication types
MeSH terms
Substances
LinkOut - more resources
Full Text Sources
Other Literature Sources