Impact of training sets on classification of high-throughput bacterial 16s rRNA gene surveys - PubMed
Impact of training sets on classification of high-throughput bacterial 16s rRNA gene surveys
Jeffrey J Werner et al. ISME J. 2012 Jan.
Abstract
Taxonomic classification of the thousands-millions of 16S rRNA gene sequences generated in microbiome studies is often achieved using a naïve Bayesian classifier (for example, the Ribosomal Database Project II (RDP) classifier), due to favorable trade-offs among automation, speed and accuracy. The resulting classification depends on the reference sequences and taxonomic hierarchy used to train the model; although the influence of primer sets and classification algorithms have been explored in detail, the influence of training set has not been characterized. We compared classification results obtained using three different publicly available databases as training sets, applied to five different bacterial 16S rRNA gene pyrosequencing data sets generated (from human body, mouse gut, python gut, soil and anaerobic digester samples). We observed numerous advantages to using the largest, most diverse training set available, that we constructed from the Greengenes (GG) bacterial/archaeal 16S rRNA gene sequence database and the latest GG taxonomy. Phylogenetic clusters of previously unclassified experimental sequences were identified with notable improvements (for example, 50% reduction in reads unclassified at the phylum level in mouse gut, soil and anaerobic digester samples), especially for phylotypes belonging to specific phyla (Tenericutes, Chloroflexi, Synergistetes and Candidate phyla TM6, TM7). Trimming the reference sequences to the primer region resulted in systematic improvements in classification depth, and greatest gains at higher confidence thresholds. Phylotypes unclassified at the genus level represented a greater proportion of the total community variation than classified operational taxonomic units in mouse gut and anaerobic digester samples, underscoring the need for greater diversity in existing reference databases.
Figures
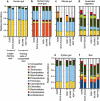
Relative abundance of the 10 major phyla identified by naïve Bayesian classification using five different training sets: three of approximately the same size: GG91.3, SILVA98.7, and RDP TS6, and two larger training sets: GG99 and the SILVA subset for Mothur. Relative abundances were averaged for samples of five different studies (note that human gut is shown apart from non-gut samples from the sample study): (a) human gut, (b) non-gut human body locations, (c) mouse gut, (d) anaerobic digester, (e) python gut and (f) soils.
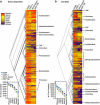
Summary of OTU classification depth using each of the three training sets for two of the four studies: (a) human body OTUs, and (b) soil OTUs (other three data sets shown in Supplementary Figure S6). OTUs are organized according to evolutionary history, as determined by the FastTree approximately-maximum-likelihood tree constructed in the default QIIME pipeline. Inset charts summarize the total number of OTUs classified at each taxonomic level (GG99=dark blue, GG91.3=light blue, SILVA=green, RDP TS6=orange).
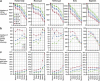
The effect of trimming the GG99 training set on classification depth, for each of the five data sets: (a) the total number of OTUs classified at each taxonomic level (Tr=trimmed; full=full length; color key indicates different percentage confidence thresholds applied to the naïve Bayesian model: 60%, 80% and 95%), (b) the total number of classified OTUs gained as a consequence of trimming the training set and (c) the percentage gain of total OTUs classified as a consequence of trimming the training set. Note that all values in (b) and (c) are positive, indicating that trimming always afforded a net gain in classification precision.
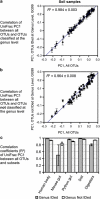
Ability of either classified or unclassified OTUs alone to recapture the variance of the whole data set. OTUs were classified using the GG99 training set. Each of the plots represent the phylogenetic variation among all soil samples, calculated using all OTUs, along the x axis (UniFrac principal coordinate 1; PC1), correlated to either OTUs that were classified to the genus level (a) or to unclassified OTUs at the genus level (b), on the y axis. If the variance in the OTU subsets (classified or unclassified) explains as much variance as the whole set, then a straight diagonal line is expected. The summary of R2 values for a similar analysis of each of the five sample types is shown for comparison (c). Error bars, and errors on R2 values, represent the s.d. of 10 rarefactions, 200 sequences each. Soil sample data are shown in (a) and (b); other four UniFrac data sets available in Supplementary Figure S7.
Similar articles
-
Chouari R, Le Paslier D, Daegelen P, Ginestet P, Weissenbach J, Sghir A. Chouari R, et al. Environ Microbiol. 2005 Aug;7(8):1104-15. doi: 10.1111/j.1462-2920.2005.00795.x. Environ Microbiol. 2005. PMID: 16011748
-
Yarza P, Yilmaz P, Pruesse E, Glöckner FO, Ludwig W, Schleifer KH, Whitman WB, Euzéby J, Amann R, Rosselló-Móra R. Yarza P, et al. Nat Rev Microbiol. 2014 Sep;12(9):635-45. doi: 10.1038/nrmicro3330. Nat Rev Microbiol. 2014. PMID: 25118885 Review.
-
F Escapa I, Huang Y, Chen T, Lin M, Kokaras A, Dewhirst FE, Lemon KP. F Escapa I, et al. Microbiome. 2020 May 15;8(1):65. doi: 10.1186/s40168-020-00841-w. Microbiome. 2020. PMID: 32414415 Free PMC article.
-
Yan YW, Zou B, Zhu T, Hozzein WN, Quan ZX. Yan YW, et al. PLoS One. 2017 Oct 10;12(10):e0186161. doi: 10.1371/journal.pone.0186161. eCollection 2017. PLoS One. 2017. PMID: 29016661 Free PMC article.
-
The dynamic history of prokaryotic phyla: discovery, diversity and division.
Pallen MJ. Pallen MJ. Int J Syst Evol Microbiol. 2024 Sep;74(9):006508. doi: 10.1099/ijsem.0.006508. Int J Syst Evol Microbiol. 2024. PMID: 39250184 Review.
Cited by
-
Tegtmeier D, Hurka S, Klüber P, Brinkrolf K, Heise P, Vilcinskas A. Tegtmeier D, et al. Front Microbiol. 2021 Mar 29;12:634503. doi: 10.3389/fmicb.2021.634503. eCollection 2021. Front Microbiol. 2021. PMID: 33854488 Free PMC article.
-
A pig model of the human gastrointestinal tract.
Zhang Q, Widmer G, Tzipori S. Zhang Q, et al. Gut Microbes. 2013 May-Jun;4(3):193-200. doi: 10.4161/gmic.23867. Epub 2013 Apr 2. Gut Microbes. 2013. PMID: 23549377 Free PMC article.
-
Truffle brûlés have an impact on the diversity of soil bacterial communities.
Mello A, Ding GC, Piceno YM, Napoli C, Tom LM, DeSantis TZ, Andersen GL, Smalla K, Bonfante P. Mello A, et al. PLoS One. 2013 Apr 30;8(4):e61945. doi: 10.1371/journal.pone.0061945. Print 2013. PLoS One. 2013. PMID: 23667413 Free PMC article.
-
Stabili L, Di Salvo M, Alifano P, Talà A. Stabili L, et al. Microb Ecol. 2022 Feb;83(2):271-283. doi: 10.1007/s00248-021-01731-w. Epub 2021 May 4. Microb Ecol. 2022. PMID: 33948706 Free PMC article.
-
Daisley BA, Reid G. Daisley BA, et al. mSystems. 2021 Apr 6;6(2):e00082-21. doi: 10.1128/mSystems.00082-21. mSystems. 2021. PMID: 33824193 Free PMC article.
References
-
- Altschul SF, Gish W, Miller W, Myers EW, Lipman DJ. Basic local alignment search tool. J Mol Biol. 1990;215:403–410. - PubMed
Publication types
MeSH terms
Substances
Grants and funding
LinkOut - more resources
Full Text Sources
Research Materials