Prediction of drug-target interactions and drug repositioning via network-based inference - PubMed
Prediction of drug-target interactions and drug repositioning via network-based inference
Feixiong Cheng et al. PLoS Comput Biol. 2012.
Abstract
Drug-target interaction (DTI) is the basis of drug discovery and design. It is time consuming and costly to determine DTI experimentally. Hence, it is necessary to develop computational methods for the prediction of potential DTI. Based on complex network theory, three supervised inference methods were developed here to predict DTI and used for drug repositioning, namely drug-based similarity inference (DBSI), target-based similarity inference (TBSI) and network-based inference (NBI). Among them, NBI performed best on four benchmark data sets. Then a drug-target network was created with NBI based on 12,483 FDA-approved and experimental drug-target binary links, and some new DTIs were further predicted. In vitro assays confirmed that five old drugs, namely montelukast, diclofenac, simvastatin, ketoconazole, and itraconazole, showed polypharmacological features on estrogen receptors or dipeptidyl peptidase-IV with half maximal inhibitory or effective concentration ranged from 0.2 to 10 µM. Moreover, simvastatin and ketoconazole showed potent antiproliferative activities on human MDA-MB-231 breast cancer cell line in MTT assays. The results indicated that these methods could be powerful tools in prediction of DTIs and drug repositioning.
Conflict of interest statement
The authors have declared that no competing interests exist.
Figures
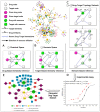
The entire workflow includes five steps: (i) collection of known drug-target interaction data and construction of bipartite drug-target graphs; (ii) calculation of drug-drug two dimensional structural similarity (SC), target-target genomic sequence similarity (Sg) and drug-target topology network similarity; (iii) application of new methods in prediction of new drugs for a given target (pink square) or new targets for a given drug (pink circle); (iv) validation of new drug-target interactions by experimental assays (D); (v) visualization of experimental results using drug-target-disease associations network analysis (E). In A–C, given drug node (pink circle) denotes the drug which we want to predict new target for, given target node (pink square) denotes the target which we want to predict new drug for, drug with resource (green circle) denotes that this drug have resource, target with resource (green square) denotes that this target have resource, the more resource a node possesses, the darker the color is, blue edges denote the drug-target interactions with known experimental evidence, black arrows denote the resource diffusion direction. In E, green circle: drug node, red square: on-target node, blue square: off-target node, yellow square: new off-target node, violet square: disease node.
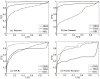
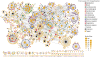
The DT network was generated using known FDA-approved small molecule DT interactions. The size of the drug node is the fraction of the number of targets that the drug linked in DrugBank. The size of the target node is the fraction of the number of drugs that the target linked in DrugBank. Color codes are given in the legend. Drug nodes (circles) are colored according to their Anatomical Therapeutic Chemical Classification. The graph was prepared by Cytoscape (
http://www.cytoscape.org/).
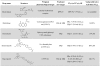
Data shown are the mean for at least triplicate measurements. aOriginal pharmacological target information was extracted from DrugBank (
http://www.DrugBank.ca/). b50% relatively effective concentration is the concentration of the tested chemical showing 50% of agonistic activity of the maximum activity of E2. REC50 provides the estrogenic activity relative to that of E2. cAntiproliferative activities were assayed on human MDA-MB-231 breast cancer cell line by MTT assays. IC50: half maximal inhibitory concentration, EC50: half maximal effective concentration, ER: Estrogen Receptors, DPP-IV: dipeptidyl peptidase-IV.
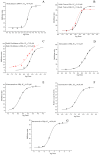
Dose-response curves for inhibitive activation: montelukast to DPP-IV (A), for transcriptional activation: tamoxifen (Tam) to ERα (black solid line) and ERβ (red dash line) (B), diclofenac to ERα (black solid line) and ERβ (red dash line) (C), simvastatin to ERβ (D), ketoconazole to ERβ (E), itraconazole to ERα (F), itraconazole to ERβ (G). In A–G, error bars were presented as the mean SD (standard deviation) of three duplicate determinations.
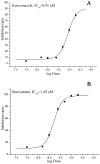
Error bars are presented as the SD (standard deviation) of three duplicate determinations.
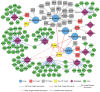
Grey arrows denote the old drug-target interactions, grey edges denote the old target-disease associations and blue edges denote the known disease-gene associations, which were extracted from DrugBank, Online Mendelian Inheritance in Man (OMIM) Morbid Map and literature reports (The further data were given in Table S7). Red arrows among approved drug nodes (cyan circle) and target nodes (yellow squares) denote the new discovered drug-target interactions in this study. Red dotted edges denote new target-disease associations discovered in this study. Cyan circle: drug node, red square: on-target (Primary targets annotated in DrugBank), grey square: off-target, yellow square: new off-target (new discovered target for a given drug validated in this study), violet square: disease node, green regular hexagon: gene. The graph was prepared by Cytoscape (
http://www.cytoscape.org/).
Similar articles
-
Inferring Interactions between Novel Drugs and Novel Targets via Instance-Neighborhood-Based Models.
Shi JY, Li JX, Chen BL, Zhang Y. Shi JY, et al. Curr Protein Pept Sci. 2018;19(5):488-497. doi: 10.2174/1389203718666161108093907. Curr Protein Pept Sci. 2018. PMID: 27829347
-
Wu Z, Cheng F, Li J, Li W, Liu G, Tang Y. Wu Z, et al. Brief Bioinform. 2017 Mar 1;18(2):333-347. doi: 10.1093/bib/bbw012. Brief Bioinform. 2017. PMID: 26944082
-
Prediction of chemical-protein interactions network with weighted network-based inference method.
Cheng F, Zhou Y, Li W, Liu G, Tang Y. Cheng F, et al. PLoS One. 2012;7(7):e41064. doi: 10.1371/journal.pone.0041064. Epub 2012 Jul 16. PLoS One. 2012. PMID: 22815915 Free PMC article.
-
Network-Based Methods for Prediction of Drug-Target Interactions.
Wu Z, Li W, Liu G, Tang Y. Wu Z, et al. Front Pharmacol. 2018 Oct 9;9:1134. doi: 10.3389/fphar.2018.01134. eCollection 2018. Front Pharmacol. 2018. PMID: 30356768 Free PMC article. Review.
-
The Computational Models of Drug-target Interaction Prediction.
Ding Y, Tang J, Guo F. Ding Y, et al. Protein Pept Lett. 2020;27(5):348-358. doi: 10.2174/0929866526666190410124110. Protein Pept Lett. 2020. PMID: 30968771 Review.
Cited by
-
Identification association of drug-disease by using functional gene module for breast cancer.
Zhu L, Zhu F. Zhu L, et al. BMC Med Genomics. 2015;8 Suppl 2(Suppl 2):S3. doi: 10.1186/1755-8794-8-S2-S3. Epub 2015 May 29. BMC Med Genomics. 2015. PMID: 26045063 Free PMC article.
-
Towards FAIR protocols and workflows: the OpenPREDICT use case.
Celebi R, Rebelo Moreira J, Hassan AA, Ayyar S, Ridder L, Kuhn T, Dumontier M. Celebi R, et al. PeerJ Comput Sci. 2020 Sep 21;6:e281. doi: 10.7717/peerj-cs.281. eCollection 2020. PeerJ Comput Sci. 2020. PMID: 33816932 Free PMC article.
-
Chung SS, Pandini A, Annibale A, Coolen AC, Thomas NS, Fraternali F. Chung SS, et al. Sci Rep. 2015 Feb 23;5:8540. doi: 10.1038/srep08540. Sci Rep. 2015. PMID: 25703051 Free PMC article.
-
In silico drug repositioning based on integrated drug targets and canonical correlation analysis.
Chen H, Zhang Z, Zhang J. Chen H, et al. BMC Med Genomics. 2022 Mar 6;15(1):48. doi: 10.1186/s12920-022-01203-1. BMC Med Genomics. 2022. PMID: 35249529 Free PMC article.
-
Bipartite graphs in systems biology and medicine: a survey of methods and applications.
Pavlopoulos GA, Kontou PI, Pavlopoulou A, Bouyioukos C, Markou E, Bagos PG. Pavlopoulos GA, et al. Gigascience. 2018 Apr 1;7(4):1-31. doi: 10.1093/gigascience/giy014. Gigascience. 2018. PMID: 29648623 Free PMC article. Review.
References
-
- Hopkins AL. Network pharmacology: the next paradigm in drug discovery. Nat Chem Biol. 2008;4:682–690. - PubMed
-
- Roth BL, Sheffler DJ, Kroeze WK. Magic shotguns versus magic bullets: selectively non-selective drugs for mood disorders and schizophrenia. Nat Rev Drug Discov. 2004;3:353–359. - PubMed
-
- Kroeze WK, Kristiansen K, Roth BL. Molecular biology of serotonin receptors structure and function at the molecular level. Curr Top Med Chem. 2002;2:507–528. - PubMed
-
- Ashburn TT, Thor KB. Drug repositioning: identifying and developing new uses for existing drugs. Nat Rev Drug Discov. 2004;3:673–683. - PubMed
-
- Boguski MS, Mandl KD, Sukhatme VP. Drug discovery. Repurposing with a difference. Science. 2009;324:1394–1395. - PubMed
Publication types
MeSH terms
Substances
LinkOut - more resources
Full Text Sources
Other Literature Sources
Medical
Miscellaneous