MoRFpred, a computational tool for sequence-based prediction and characterization of short disorder-to-order transitioning binding regions in proteins - PubMed
- ️Sun Jan 01 2012
Comparative Study
MoRFpred, a computational tool for sequence-based prediction and characterization of short disorder-to-order transitioning binding regions in proteins
Fatemeh Miri Disfani et al. Bioinformatics. 2012.
Abstract
Motivation: Molecular recognition features (MoRFs) are short binding regions located within longer intrinsically disordered regions that bind to protein partners via disorder-to-order transitions. MoRFs are implicated in important processes including signaling and regulation. However, only a limited number of experimentally validated MoRFs is known, which motivates development of computational methods that predict MoRFs from protein chains.
Results: We introduce a new MoRF predictor, MoRFpred, which identifies all MoRF types (α, β, coil and complex). We develop a comprehensive dataset of annotated MoRFs to build and empirically compare our method. MoRFpred utilizes a novel design in which annotations generated by sequence alignment are fused with predictions generated by a Support Vector Machine (SVM), which uses a custom designed set of sequence-derived features. The features provide information about evolutionary profiles, selected physiochemical properties of amino acids, and predicted disorder, solvent accessibility and B-factors. Empirical evaluation on several datasets shows that MoRFpred outperforms related methods: α-MoRF-Pred that predicts α-MoRFs and ANCHOR which finds disordered regions that become ordered when bound to a globular partner. We show that our predicted (new) MoRF regions have non-random sequence similarity with native MoRFs. We use this observation along with the fact that predictions with higher probability are more accurate to identify putative MoRF regions. We also identify a few sequence-derived hallmarks of MoRFs. They are characterized by dips in the disorder predictions and higher hydrophobicity and stability when compared to adjacent (in the chain) residues.
Availability: http://biomine.ece.ualberta.ca/MoRFpred/; http://biomine.ece.ualberta.ca/MoRFpred/Supplement.pdf.
Figures
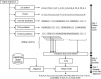
Architecture of the MoRFpred method
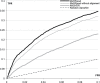
Comparison of ROCs for MoRFpred and ANCHOR on the test dataset. The ROC curves are provided for the FPR < 0.1
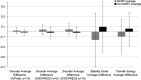
Analysis of the top-ranked features that serve as sequence-derived markers of MoRFs. The average values of the top five ranked features used by MoRFpred, which are shown on the x-axis, for the native MoRF residues (light gray bars) and native non-MoRF residues (dark gray bars) are compared. The corresponding standard deviations are shown using the error bars. The selected five features represent an average difference of a given quantity (predicted disorder, stability or transfer energy). Negative values mean that average in the inner window of size w was higher than the average in the flanking segments
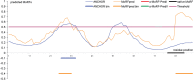
Prediction of MoRF residues for the Histone H2A protein by ANCHOR (blue lines), MoRFpred (orange lines), α-MoRF−PredI (thick red line) and α-MoRF-PredI I (thick green line) predictors. The x-axis shows positions in the protein sequence. Probability values are only available for ANCHOR and MoRFpred and are shown by thin blue and orange lines, respectively, at the top of the figure. The cutoff of 0.5 to convert probabilities into binary predictions for ANCHOR and MoRFpred is shown using a brown horizontal line. The native MoRF regions are annotated using black horizontal line. The binary predictions from ANCHOR, α-MoRF−PredI, α-MoRF-PredI I and MoRFpred are denoted using blue (at the −0.1 point on the y-axis), red (at the −0.2), green (at the −0.3) and orange (at the −0.4) horizontal lines. Lack of red and green lines means that α-MoRF−PredI and α-MoRF-PredII did not predict MoRFs
Similar articles
-
Sharma R, Bayarjargal M, Tsunoda T, Patil A, Sharma A. Sharma R, et al. J Theor Biol. 2018 Jan 21;437:9-16. doi: 10.1016/j.jtbi.2017.10.015. Epub 2017 Oct 16. J Theor Biol. 2018. PMID: 29042212
-
Computational identification of MoRFs in protein sequences.
Malhis N, Gsponer J. Malhis N, et al. Bioinformatics. 2015 Jun 1;31(11):1738-44. doi: 10.1093/bioinformatics/btv060. Epub 2015 Jan 30. Bioinformatics. 2015. PMID: 25637562 Free PMC article.
-
Predicting Functions of Disordered Proteins with MoRFpred.
Oldfield CJ, Uversky VN, Kurgan L. Oldfield CJ, et al. Methods Mol Biol. 2019;1851:337-352. doi: 10.1007/978-1-4939-8736-8_19. Methods Mol Biol. 2019. PMID: 30298407
-
Computational Prediction of MoRFs, Short Disorder-to-order Transitioning Protein Binding Regions.
Katuwawala A, Peng Z, Yang J, Kurgan L. Katuwawala A, et al. Comput Struct Biotechnol J. 2019 Mar 26;17:454-462. doi: 10.1016/j.csbj.2019.03.013. eCollection 2019. Comput Struct Biotechnol J. 2019. PMID: 31007871 Free PMC article. Review.
-
Li H, Pang Y, Liu B, Yu L. Li H, et al. Front Pharmacol. 2022 Mar 8;13:856417. doi: 10.3389/fphar.2022.856417. eCollection 2022. Front Pharmacol. 2022. PMID: 35350759 Free PMC article. Review.
Cited by
-
MoRFchibi SYSTEM: software tools for the identification of MoRFs in protein sequences.
Malhis N, Jacobson M, Gsponer J. Malhis N, et al. Nucleic Acids Res. 2016 Jul 8;44(W1):W488-93. doi: 10.1093/nar/gkw409. Epub 2016 May 12. Nucleic Acids Res. 2016. PMID: 27174932 Free PMC article.
-
Hwang Fu YH, Chandrasekar S, Lee JH, Shan SO. Hwang Fu YH, et al. J Cell Biol. 2019 Oct 7;218(10):3307-3319. doi: 10.1083/jcb.201901001. Epub 2019 Sep 19. J Cell Biol. 2019. PMID: 31537711 Free PMC article.
-
Ordered disorder of the astrocytic dystrophin-associated protein complex in the norm and pathology.
Na I, Redmon D, Kopa M, Qin Y, Xue B, Uversky VN. Na I, et al. PLoS One. 2013 Aug 27;8(8):e73476. doi: 10.1371/journal.pone.0073476. eCollection 2013. PLoS One. 2013. PMID: 24014171 Free PMC article.
-
Structure and function of yeast Atg20, a sorting nexin that facilitates autophagy induction.
Popelka H, Damasio A, Hinshaw JE, Klionsky DJ, Ragusa MJ. Popelka H, et al. Proc Natl Acad Sci U S A. 2017 Nov 21;114(47):E10112-E10121. doi: 10.1073/pnas.1708367114. Epub 2017 Nov 7. Proc Natl Acad Sci U S A. 2017. PMID: 29114050 Free PMC article.
-
Intrinsic Disorder in Plant Transcription Factor Systems: Functional Implications.
Salladini E, Jørgensen MLM, Theisen FF, Skriver K. Salladini E, et al. Int J Mol Sci. 2020 Dec 21;21(24):9755. doi: 10.3390/ijms21249755. Int J Mol Sci. 2020. PMID: 33371315 Free PMC article. Review.