Hybridization-based reconstruction of small non-coding RNA transcripts from deep sequencing data - PubMed
- ️Invalid Date
Hybridization-based reconstruction of small non-coding RNA transcripts from deep sequencing data
Chikako Ragan et al. Nucleic Acids Res. 2012 Sep.
Abstract
Recent advances in RNA sequencing technology (RNA-Seq) enables comprehensive profiling of RNAs by producing millions of short sequence reads from size-fractionated RNA libraries. Although conventional tools for detecting and distinguishing non-coding RNAs (ncRNAs) from reference-genome data can be applied to sequence data, ncRNA detection can be improved by harnessing the full information content provided by this new technology. Here we present NorahDesk, the first unbiased and universally applicable method for small ncRNAs detection from RNA-Seq data. NorahDesk utilizes the coverage-distribution of small RNA sequence data as well as thermodynamic assessments of secondary structure to reliably predict and annotate ncRNA classes. Using publicly available mouse sequence data from brain, skeletal muscle, testis and ovary, we evaluated our method with an emphasis on the performance for microRNAs (miRNAs) and piwi-interacting small RNA (piRNA). We compared our method with Dario and mirDeep2 and found that NorahDesk produces longer transcripts with higher read coverage. This feature makes it the first method particularly suitable for the prediction of both known and novel piRNAs.
Figures
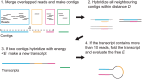
Steps to reconstruct small ncRNA transcripts.
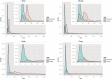
Size distribution of contigs and predicted transcripts. The shift in the size distribution of contigs (blue) and predicted transcripts (red) in brain, muscle, testis and ovary. The x-axis shows the size in number of nucleotides and the y-axis shows the corresponding density as the smoothed and normalized contig- and transcript count, respectively.
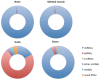
Distribution of ncRNA types in different tissues. The figures show the fraction of reads overlap with known and novel classes of RNAs in brain, skeletal muscles, testis and ovary.
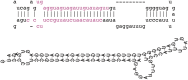
Known versus predicted structure of mmu-let-7f1. The top figure shows the known structure of mmu-let-7f1 from miRBase and the bottom shows the predicted structure of reconstructed transcript. The mature miRNA-duplex is shown in purple.
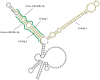
Example of long miRNA transcript. Predicted miRNA transcript from chr17:17967156-17967398 (+strand) overlaps with miR-99b precursor and contains additional one un-annotated contig.
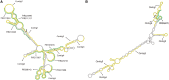
Examples of predicted piRNA transcripts. (A) Predicted piRNAs transcripts from chr10:18517077-18517536 (-strand); 4 out of 7 contigs overlap with known piRNAs and all contigs have 5′ uridine. (B) Predicted piRNAs transcripts from chr6:128121896-128122316 (-strand); only one out of 6 contigs overlap with a known piRNA and 4 out of 6 contigs have 5′ uridine.
Similar articles
-
Shi J, Ko EA, Sanders KM, Chen Q, Zhou T. Shi J, et al. Genomics Proteomics Bioinformatics. 2018 Apr;16(2):144-151. doi: 10.1016/j.gpb.2018.04.004. Epub 2018 May 3. Genomics Proteomics Bioinformatics. 2018. PMID: 29730207 Free PMC article.
-
Giurato G, De Filippo MR, Rinaldi A, Hashim A, Nassa G, Ravo M, Rizzo F, Tarallo R, Weisz A. Giurato G, et al. BMC Bioinformatics. 2013 Dec 13;14:362. doi: 10.1186/1471-2105-14-362. BMC Bioinformatics. 2013. PMID: 24330401 Free PMC article.
-
Identification and classification of small RNAs in transcriptome sequence data.
Langenberger D, Bermudez-Santana CI, Stadler PF, Hoffmann S. Langenberger D, et al. Pac Symp Biocomput. 2010:80-7. doi: 10.1142/9789814295291_0010. Pac Symp Biocomput. 2010. PMID: 19908360
-
Resources for Small Regulatory RNAs.
Bell GW, Lewitter F. Bell GW, et al. Curr Protoc Mol Biol. 2014 Jul 1;107:19.8.1-19.8.14. doi: 10.1002/0471142727.mb1908s107. Curr Protoc Mol Biol. 2014. PMID: 24984849 Review.
-
Biases in small RNA deep sequencing data.
Raabe CA, Tang TH, Brosius J, Rozhdestvensky TS. Raabe CA, et al. Nucleic Acids Res. 2014 Feb;42(3):1414-26. doi: 10.1093/nar/gkt1021. Epub 2013 Nov 5. Nucleic Acids Res. 2014. PMID: 24198247 Free PMC article. Review.
Cited by
-
Wu J, Liu Q, Wang X, Zheng J, Wang T, You M, Sheng Sun Z, Shi Q. Wu J, et al. RNA Biol. 2013 Jul;10(7):1087-92. doi: 10.4161/rna.25193. Epub 2013 May 29. RNA Biol. 2013. PMID: 23778453 Free PMC article.
-
Alkbh1 and Tzfp repress a non-repeat piRNA cluster in pachytene spermatocytes.
Nordstrand LM, Furu K, Paulsen J, Rognes T, Klungland A. Nordstrand LM, et al. Nucleic Acids Res. 2012 Nov;40(21):10950-63. doi: 10.1093/nar/gks839. Epub 2012 Sep 10. Nucleic Acids Res. 2012. PMID: 22965116 Free PMC article.
-
DANSR: A Tool for the Detection of Annotated and Novel Small RNAs.
Zhang J, Eteleeb AM, Rozycki EB, Inkman MJ, Ly A, Scharf RE, Jayachandran K, Krasnick BA, Mazur T, White NM, Fields RC, Maher CA. Zhang J, et al. Noncoding RNA. 2022 Jan 13;8(1):9. doi: 10.3390/ncrna8010009. Noncoding RNA. 2022. PMID: 35076605 Free PMC article.
-
Automatic learning of pre-miRNAs from different species.
O N Lopes Id, Schliep A, de L F de Carvalho AP. O N Lopes Id, et al. BMC Bioinformatics. 2016 May 28;17(1):224. doi: 10.1186/s12859-016-1036-3. BMC Bioinformatics. 2016. PMID: 27233515 Free PMC article.
-
Li Y, Li J, Fang C, Shi L, Tan J, Xiong Y, Bin Fan, Li C. Li Y, et al. Sci Rep. 2016 May 27;6:26852. doi: 10.1038/srep26852. Sci Rep. 2016. PMID: 27229484 Free PMC article.
References
-
- Mattick JS, Taft RJ, Faulkner GJ. A global view of genomic information–moving beyond the gene and the master regulator. Trends Genet. 2010;26:21–28. - PubMed
-
- Friedländer MR, Chen W, Adamidi C, Maaskola J, Einspanier R, Knespel S, Rajewsky N. Discovering microRNAs from deep sequencing data using miRDeep. Nat. Biotechnol. 2008;26:407–415. - PubMed