CoRAL: predicting non-coding RNAs from small RNA-sequencing data - PubMed
CoRAL: predicting non-coding RNAs from small RNA-sequencing data
Yuk Yee Leung et al. Nucleic Acids Res. 2013 Aug.
Abstract
The surprising observation that virtually the entire human genome is transcribed means we know little about the function of many emerging classes of RNAs, except their astounding diversities. Traditional RNA function prediction methods rely on sequence or alignment information, which are limited in their abilities to classify the various collections of non-coding RNAs (ncRNAs). To address this, we developed Classification of RNAs by Analysis of Length (CoRAL), a machine learning-based approach for classification of RNA molecules. CoRAL uses biologically interpretable features including fragment length and cleavage specificity to distinguish between different ncRNA populations. We evaluated CoRAL using genome-wide small RNA sequencing data sets from four human tissue types and were able to classify six different types of RNAs with ∼80% cross-validation accuracy. Analysis by CoRAL revealed that microRNAs, small nucleolar and transposon-derived RNAs are highly discernible and consistent across all human tissue types assessed, whereas long intergenic ncRNAs, small cytoplasmic RNAs and small nuclear RNAs show less consistent patterns. The ability to reliably annotate loci across tissue types demonstrates the potential of CoRAL to characterize ncRNAs using small RNA sequencing data in less well-characterized organisms.
Figures
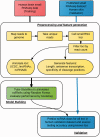
The analysis workflow for differentiating between six different classes of ncRNAs in smRNA-seq data sets.
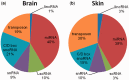
Percentage of small ncRNA loci identified by smRNA-seq for two human tissue types: (a) brain and (b) skin.
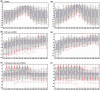
Feature spectrum plots for three of the ncRNA classes (as specified in the figure), in the (a–c) brain data and (d–f) the skin data. Each box corresponds to one length feature, and each grey line represents one locus. The red dots are outside of the 99th percentile of each distribution.
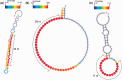
smRNA-seq reads plotted on the predicted RNA secondary structures using SAVoR (14) for (a) an miRNA, (b) a C/D box snoRNA and (c) a transposon-derived RNA. The miRNA and C/D box snoRNA structures are as reported by RFAM, and the transposon-derived RNA structure is as predicted by RNAfold.
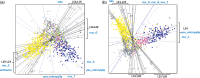
MDS based projections of the data for (a) brain and (b) skin. The three most discriminative classes are miRNA (yellow), C/D box snoRNA (blue) and transposon-derived RNAs (grey).
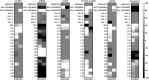
Selected features in each of the two data sets (as specified) for the six-class classifier: antisense expression (antisense), 5′ and 3′ smRNA positional entropy (pos_entropy5p and pos_entropy3p), nucleotide preference (nuc_A, nucC, nuc_G and nuc_T), MFE value and the smRNA length features from 14 to 30 nt (L14–L30). The sign of the value indicates whether the feature was larger (positive) or smaller (negative) within that class, on average, than the other classes (by difference of means).
Similar articles
-
Ryvkin P, Leung YY, Ungar LH, Gregory BD, Wang LS. Ryvkin P, et al. Methods. 2014 May 1;67(1):28-35. doi: 10.1016/j.ymeth.2013.10.002. Epub 2013 Oct 18. Methods. 2014. PMID: 24145223 Free PMC article.
-
Identification and classification of small RNAs in transcriptome sequence data.
Langenberger D, Bermudez-Santana CI, Stadler PF, Hoffmann S. Langenberger D, et al. Pac Symp Biocomput. 2010:80-7. doi: 10.1142/9789814295291_0010. Pac Symp Biocomput. 2010. PMID: 19908360
-
Asim MN, Ibrahim MA, Imran Malik M, Dengel A, Ahmed S. Asim MN, et al. Int J Mol Sci. 2021 Aug 13;22(16):8719. doi: 10.3390/ijms22168719. Int J Mol Sci. 2021. PMID: 34445436 Free PMC article. Review.
-
Hybridization-based reconstruction of small non-coding RNA transcripts from deep sequencing data.
Ragan C, Mowry BJ, Bauer DC. Ragan C, et al. Nucleic Acids Res. 2012 Sep;40(16):7633-43. doi: 10.1093/nar/gks505. Epub 2012 Jun 16. Nucleic Acids Res. 2012. PMID: 22705792 Free PMC article.
-
An introduction to small non-coding RNAs: miRNA and snoRNA.
Holley CL, Topkara VK. Holley CL, et al. Cardiovasc Drugs Ther. 2011 Apr;25(2):151-9. doi: 10.1007/s10557-011-6290-z. Cardiovasc Drugs Ther. 2011. PMID: 21573765 Review.
Cited by
-
NoFold: RNA structure clustering without folding or alignment.
Middleton SA, Kim J. Middleton SA, et al. RNA. 2014 Nov;20(11):1671-83. doi: 10.1261/rna.041913.113. Epub 2014 Sep 18. RNA. 2014. PMID: 25234928 Free PMC article.
-
Ryvkin P, Leung YY, Ungar LH, Gregory BD, Wang LS. Ryvkin P, et al. Methods. 2014 May 1;67(1):28-35. doi: 10.1016/j.ymeth.2013.10.002. Epub 2013 Oct 18. Methods. 2014. PMID: 24145223 Free PMC article.
-
A Review on Recent Computational Methods for Predicting Noncoding RNAs.
Zhang Y, Huang H, Zhang D, Qiu J, Yang J, Wang K, Zhu L, Fan J, Yang J. Zhang Y, et al. Biomed Res Int. 2017;2017:9139504. doi: 10.1155/2017/9139504. Epub 2017 May 3. Biomed Res Int. 2017. PMID: 28553651 Free PMC article. Review.
-
In Silico Identification of RNA Modifications from High-Throughput Sequencing Data Using HAMR.
Kuksa PP, Leung YY, Vandivier LE, Anderson Z, Gregory BD, Wang LS. Kuksa PP, et al. Methods Mol Biol. 2017;1562:211-229. doi: 10.1007/978-1-4939-6807-7_14. Methods Mol Biol. 2017. PMID: 28349463 Free PMC article.
-
Integrating Epigenomics into the Understanding of Biomedical Insight.
Han Y, He X. Han Y, et al. Bioinform Biol Insights. 2016 Dec 4;10:267-289. doi: 10.4137/BBI.S38427. eCollection 2016. Bioinform Biol Insights. 2016. PMID: 27980397 Free PMC article. Review.
References
-
- Eddy SR. Non-coding RNA genes and the modern RNA world. Nat. Rev. Genet. 2001;2:919–929. - PubMed
-
- Todd G, Karbstein K. RNA takes center stage. Biopolymers. 2007;87:275–278. - PubMed
-
- Khalil AM, Guttman M, Huarte M, Garber M, Raj A, Rivea Morales D, Thomas K, Presser A, Bernstein BE, Van Oudenaarden A, et al. Many human large intergenic noncoding RNAs associate with chromatin-modifying complexes and affect gene expression. Proc. Natl Acad. Sci. U.S.A. 2009;106:11667–11672. - PMC - PubMed
-
- Black DL, Chabot B, Steitz JA. U2 as well as U1 small nuclear ribonucleoproteins are involved in premessenger RNA splicing. Cell. 1985;42:737–750. - PubMed
Publication types
MeSH terms
Substances
Grants and funding
LinkOut - more resources
Full Text Sources
Other Literature Sources