How attention extracts objects from noise - PubMed
How attention extracts objects from noise
Michael S Pratte et al. J Neurophysiol. 2013 Sep.
Abstract
The visual system is remarkably proficient at extracting relevant object information from noisy, cluttered environments. Although attention is known to enhance sensory processing, the mechanisms by which attention extracts relevant information from noise are not well understood. According to the perceptual template model, attention may act to amplify responses to all visual input, or it may act as a noise filter, dampening responses to irrelevant visual noise. Amplification allows for improved performance in the absence of visual noise, whereas a noise-filtering mechanism can only improve performance if the target stimulus appears in noise. Here, we used fMRI to investigate how attention modulates cortical responses to objects at multiple levels of the visual pathway. Participants viewed images of faces, houses, chairs, and shoes, presented in various levels of visual noise. We used multivoxel pattern analysis to predict the viewed object category, for attended and unattended stimuli, from cortical activity patterns in individual visual areas. Early visual areas, V1 and V2, exhibited a benefit of attention only at high levels of visual noise, suggesting that attention operates via a noise-filtering mechanism at these early sites. By contrast, attention led to enhanced processing of noise-free images (i.e., amplification) only in higher visual areas, including area V4, fusiform face area, mid-Fusiform area, and the lateral occipital cortex. Together, these results suggest that attention improves people's ability to discriminate objects by de-noising visual input in early visual areas and amplifying this noise-reduced signal at higher stages of visual processing.
Keywords: decoding; equivalent noise; functional magnetic resonance imaging; noise reduction; primary visual cortex; selective attention.
Figures
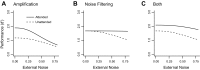
Predictions of the perceptual template model. Performance is plotted as a function of external noise for attended (solid curves) and unattended (dashed curves) conditions. Performance could be a behavioral measure or the accuracy of fMRI pattern classification. A: pure amplification is evidenced by an attentional benefit at low noise levels, but no effect at high noise. B: pure noise filtering is evidenced by an attentional benefit at high levels of noise, but no effect at low noise levels. C: if both amplification and noise-filtering effects are present, then attention is expected to increase performance accuracy across all noise levels.
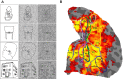
Experimental stimuli and cortical regions of interest. A: stimuli consisted of images of shoes, chairs, faces, and houses. The left column shows images with zero noise, the middle and right columns show the same images with 50% and 75% noise, respectively. The objects were still identifiable in the highest noise condition. B: a flattened representation of the right occipital lobe for 1 participant. The activity map shows significant BOLD activity in the visual localizer experiment. VO, ventral occipital cortex; LO, lateral occipital cortex; FFA, fusiform face area; mFus, mid-Fusiform area.
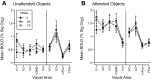
Mean BOLD activity for each noise condition, plotted by visual area separately for unattended (A) and attended (B) conditions. Error bars denote ±1 SE. Raw BOLD amplitudes were converted to percent signal change units by dividing the mean time course of each voxel by the mean signal for that voxel over the first 16 s of fixation that began each run.
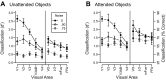
fMRI pattern classification accuracy plotted across visual areas for each noise level in the unattended (A) and attended (B) conditions. Error bars denote standard errors. Data are in d′ units (left axis); corresponding 4-choice accuracy is shown on the rightmost axis.
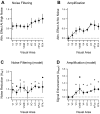
Measures of noise filtering and amplification for individual visual areas. A: attention effect (attended-unattended classification performance) for each visual area in the high-noise condition. B: attention effect in the no-noise condition. C: parameter estimates of noise filtering from perceptual template model (PTM) fits to each visual area. D: parameter estimates of amplification from PTM fits. Parameter estimates that significantly exceed 1.0 indicate a reliable multiplicative effect; asterisks and black circles denote significant (P < 0.05) and marginally significant (P < 0.1) effects, respectively. Error bars denote standard errors.
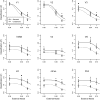
Classification accuracy in each visual area plotted as a function of external noise level for attended and unattended conditions. Points denote the data with standard errors; asterisks and black circles denote statistically significant (P < 0.05) and marginally significant (P < 0.1) attentional effects, respectively, for each area and noise level. Curves indicate PTM fits to the fMRI classification data, obtained by generating predictions for each participant and averaging over participants. Whereas the pattern of results in areas V1 and V2 are consistent with pure noise reduction, higher-level areas exhibit both noise reduction and amplification.
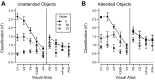
A pattern classifier was trained on the zero-noise unattended condition and used to classify object category in all other conditions. The results are similar to the above analyses, in which classifiers were separately trained and tested within each condition, suggesting that the nature of object-category information is qualitatively similar across attention and noise conditions, but that the strengths of these patterns are modified by attention and noise.
Similar articles
-
Neural mechanisms of object-based attention.
Cohen EH, Tong F. Cohen EH, et al. Cereb Cortex. 2015 Apr;25(4):1080-92. doi: 10.1093/cercor/bht303. Epub 2013 Nov 11. Cereb Cortex. 2015. PMID: 24217991 Free PMC article.
-
Neural basis of identity information extraction from noisy face images.
Hermann P, Bankó ÉM, Gál V, Vidnyánszky Z. Hermann P, et al. J Neurosci. 2015 May 6;35(18):7165-73. doi: 10.1523/JNEUROSCI.3572-14.2015. J Neurosci. 2015. PMID: 25948266 Free PMC article. Clinical Trial.
-
Rainer G, Augath M, Trinath T, Logothetis NK. Rainer G, et al. Curr Biol. 2001 Jun 5;11(11):846-54. doi: 10.1016/s0960-9822(01)00242-1. Curr Biol. 2001. PMID: 11516645
-
Vuilleumier P, Pourtois G. Vuilleumier P, et al. Neuropsychologia. 2007 Jan 7;45(1):174-94. doi: 10.1016/j.neuropsychologia.2006.06.003. Epub 2006 Jul 18. Neuropsychologia. 2007. PMID: 16854439 Review.
-
Representation of Naturalistic Image Structure in the Primate Visual Cortex.
Movshon JA, Simoncelli EP. Movshon JA, et al. Cold Spring Harb Symp Quant Biol. 2014;79:115-22. doi: 10.1101/sqb.2014.79.024844. Epub 2015 May 5. Cold Spring Harb Symp Quant Biol. 2014. PMID: 25943766 Free PMC article. Review.
Cited by
-
Janes AC, Farmer S, Peechatka AL, Frederick Bde B, Lukas SE. Janes AC, et al. Neuropsychopharmacology. 2015 Jun;40(7):1561-8. doi: 10.1038/npp.2015.9. Epub 2015 Jan 8. Neuropsychopharmacology. 2015. PMID: 25567427 Free PMC article.
-
Contour interpolation: A case study in Modularity of Mind.
Keane BP. Keane BP. Cognition. 2018 May;174:1-18. doi: 10.1016/j.cognition.2018.01.008. Epub 2018 Feb 3. Cognition. 2018. PMID: 29407601 Free PMC article.
-
Lu ZL, Dosher BA. Lu ZL, et al. J Vis. 2023 Feb 1;23(2):12. doi: 10.1167/jov.23.2.12. J Vis. 2023. PMID: 36826825 Free PMC article.
-
Ban H, Yamamoto H, Hanakawa T, Urayama S, Aso T, Fukuyama H, Ejima Y. Ban H, et al. J Neurosci. 2013 Oct 23;33(43):16992-7007. doi: 10.1523/JNEUROSCI.1455-12.2013. J Neurosci. 2013. PMID: 24155304 Free PMC article.
-
Wattanathorn J, Somboonporn W, Thukham-Mee W, Sungkamnee S. Wattanathorn J, et al. Foods. 2022 Sep 2;11(17):2678. doi: 10.3390/foods11172678. Foods. 2022. PMID: 36076862 Free PMC article.
References
-
- Beauchamp MS, Cox RW, DeYoe EA. Graded effects of spatial and featural attention on human area MT and associated motion processing areas. J Neurophysiol 78: 516–520, 1997 - PubMed
-
- Boser BE, Guyon IM, Vapnik VN. A training algorithm for optimal margin classifiers. In: Proceedings of the Fifth Annual Workshop on Computational Learning Theory, Pittsburgh, PA, 1992, p. 144–152
-
- Brainard DH. The psychophysics toolbox. Spatial Vision 10: 433–436, 1997 - PubMed
Publication types
MeSH terms
LinkOut - more resources
Full Text Sources
Other Literature Sources