Selecting optimal partitioning schemes for phylogenomic datasets - PubMed
- ️Wed Jan 01 2014
Selecting optimal partitioning schemes for phylogenomic datasets
Robert Lanfear et al. BMC Evol Biol. 2014.
Abstract
Background: Partitioning involves estimating independent models of molecular evolution for different subsets of sites in a sequence alignment, and has been shown to improve phylogenetic inference. Current methods for estimating best-fit partitioning schemes, however, are only computationally feasible with datasets of fewer than 100 loci. This is a problem because datasets with thousands of loci are increasingly common in phylogenetics.
Methods: We develop two novel methods for estimating best-fit partitioning schemes on large phylogenomic datasets: strict and relaxed hierarchical clustering. These methods use information from the underlying data to cluster together similar subsets of sites in an alignment, and build on clustering approaches that have been proposed elsewhere.
Results: We compare the performance of our methods to each other, and to existing methods for selecting partitioning schemes. We demonstrate that while strict hierarchical clustering has the best computational efficiency on very large datasets, relaxed hierarchical clustering provides scalable efficiency and returns dramatically better partitioning schemes as assessed by common criteria such as AICc and BIC scores.
Conclusions: These two methods provide the best current approaches to inferring partitioning schemes for very large datasets. We provide free open-source implementations of the methods in the PartitionFinder software. We hope that the use of these methods will help to improve the inferences made from large phylogenomic datasets.
Figures
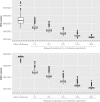
The strict clustering algorithm performs poorly, but the relaxed clustering algorithm performs almost as well as the greedy algorithm. All analyses were conducted on a phylogenomic dataset of birds (Table 1, Hackett_2008). Note that lower scores indicate a better fit of the model to the data. The dashed line in each plot shows the score of the best partitioning scheme found by the greedy algorithm. Each boxplot represent the distribution of scores for 1000 runs of the strict or relaxed clustering algorithms, where each run uses a different definition of the similarity of two subsets (see main text). The figure shows that the relaxed clustering algorithm’s performance approaches that of the greedy algorithm as P increases, and that analysing 10% of partitioning schemes results in information theoretic scores that are very close to that of the greedy algorithm.
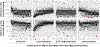
The performance of the strict clustering algorithm varies dramatically depending on the weighting schemes used to define subset similarity. The Y axis shows the difference in the AICc or BIC score compared to the best scheme found by the strict hierarchical clustering algorithm on the phylogenomic dataset from birds (Table 1). The X axes show the weights assigned to each of four parameter classes used to define subset similarity. Each panel shows 1000 data points, where each datapoint represents a single run of the strict hierarchical clustering algorithm under a particular weighting scheme. The set of four weights under which the best scheme was found by the strict hierarchical clustering algorithm are shown in red.
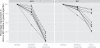
The relaxed clustering algorithm outperforms the strict clustering algorithm across the 10 datasets shown in Table1. All scores are standardised by the score increase achieved by the greedy algorithm (i.e. the score of the best partitioning scheme from the greedy algorithm minus the score of the starting scheme), so that performance can be compared across datasets. Thus, the greedy algorithm always scores 100%, and is shown only for reference. Each line connects the results from a single dataset, demonstrating that in all cases using both the AICc and the BIC, the greedy algorithm performed best, the relaxed clustering algorithm (with 10% of schemes analysed) performed second best, and the strict clustering algorithm performed the worst. All analyses use the RAxML version of PartitionFinder.
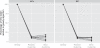
The strict and relaxed clustering algorithms are computationally much more efficient than the greedy algorithm. This figure shows the time taken by the relaxed and strict hierarchical clustering algorithms on the 10 datasets shown in Table 1, relative to the time taken by the greedy algorithm. All times are standardised by the time taken by the greedy algorithm, so that performance can be compared across datasets. Thus, the greedy algorithm always scores 100%, and is shown only for reference. Each line connects the results from a single dataset. The results show that the relaxed clustering algorithm (with 10% of schemes analysed) consistently takes about 10% of the time taken by the greedy algorithm, and that the strict hierarchical clustering algorithm takes between around 1% to 20% of the time taken by the greedy algorithm, depending on the dataset. All analyses use the RAxML version of PartitionFinder.
Similar articles
-
Lanfear R, Calcott B, Ho SY, Guindon S. Lanfear R, et al. Mol Biol Evol. 2012 Jun;29(6):1695-701. doi: 10.1093/molbev/mss020. Epub 2012 Jan 20. Mol Biol Evol. 2012. PMID: 22319168
-
Frandsen PB, Calcott B, Mayer C, Lanfear R. Frandsen PB, et al. BMC Evol Biol. 2015 Feb 10;15(1):13. doi: 10.1186/s12862-015-0283-7. BMC Evol Biol. 2015. PMID: 25887041 Free PMC article.
-
Lanfear R, Frandsen PB, Wright AM, Senfeld T, Calcott B. Lanfear R, et al. Mol Biol Evol. 2017 Mar 1;34(3):772-773. doi: 10.1093/molbev/msw260. Mol Biol Evol. 2017. PMID: 28013191
-
Phylogenomic inference of protein molecular function: advances and challenges.
Sjölander K. Sjölander K. Bioinformatics. 2004 Jan 22;20(2):170-9. doi: 10.1093/bioinformatics/bth021. Bioinformatics. 2004. PMID: 14734307 Review.
-
Distance-based phylogenetic inference from typing data: a unifying view.
Vaz C, Nascimento M, Carriço JA, Rocher T, Francisco AP. Vaz C, et al. Brief Bioinform. 2021 May 20;22(3):bbaa147. doi: 10.1093/bib/bbaa147. Brief Bioinform. 2021. PMID: 32734294 Review.
Cited by
-
Figueroa DF, Baco AR. Figueroa DF, et al. Genome Biol Evol. 2014 Dec 24;7(1):391-409. doi: 10.1093/gbe/evu286. Genome Biol Evol. 2014. PMID: 25539723 Free PMC article.
-
Straube N, Li C, Mertzen M, Yuan H, Moritz T. Straube N, et al. BMC Evol Biol. 2018 Oct 23;18(1):158. doi: 10.1186/s12862-018-1267-1. BMC Evol Biol. 2018. PMID: 30352561 Free PMC article.
-
Jones KE, Fér T, Schmickl RE, Dikow RB, Funk VA, Herrando-Moraira S, Johnston PR, Kilian N, Siniscalchi CM, Susanna A, Slovák M, Thapa R, Watson LE, Mandel JR. Jones KE, et al. Appl Plant Sci. 2019 Oct 25;7(10):e11295. doi: 10.1002/aps3.11295. eCollection 2019 Oct. Appl Plant Sci. 2019. PMID: 31667023 Free PMC article.
-
Species Tree Estimation and the Impact of Gene Loss Following Whole-Genome Duplication.
Xiong H, Wang D, Shao C, Yang X, Yang J, Ma T, Davis CC, Liu L, Xi Z. Xiong H, et al. Syst Biol. 2022 Oct 12;71(6):1348-1361. doi: 10.1093/sysbio/syac040. Syst Biol. 2022. PMID: 35689633 Free PMC article.
-
New Ascomycetes from the Mexican Tropical Montane Cloud Forest.
Raymundo T, Valenzuela R, Martínez-González CR, García-Jiménez J, Cobos-Villagrán A, Sánchez-Flores M, de la Fuente J, Martínez-Pineda M, Pérez-Valdespino A, Ramírez-Martínez JC, Luna-Vega I. Raymundo T, et al. J Fungi (Basel). 2023 Sep 15;9(9):933. doi: 10.3390/jof9090933. J Fungi (Basel). 2023. PMID: 37755041 Free PMC article.
References
Publication types
MeSH terms
LinkOut - more resources
Full Text Sources
Other Literature Sources