Predicting dynamic signaling network response under unseen perturbations - PubMed
Predicting dynamic signaling network response under unseen perturbations
Fan Zhu et al. Bioinformatics. 2014 Oct.
Abstract
Motivation: Predicting trajectories of signaling networks under complex perturbations is one of the most valuable, but challenging, tasks in systems biology. Signaling networks are involved in most of the biological pathways, and modeling their dynamics has wide applications including drug design and treatment outcome prediction.
Results: In this paper, we report a novel model for predicting the cell type-specific time course response of signaling proteins under unseen perturbations. This algorithm achieved the top performance in the 2013 8th Dialogue for Reverse Engineering Assessments and Methods (DREAM 8) subchallenge: time course prediction in breast cancer cell lines. We formulate the trajectory prediction problem into a standard regularization problem; the solution becomes solving this discrete ill-posed problem. This algorithm includes three steps: denoising, estimating regression coefficients and modeling trajectories under unseen perturbations. We further validated the accuracy of this method against simulation and experimental data. Furthermore, this method reduces computational time by magnitudes compared to state-of-the-art methods, allowing genome-wide modeling of signaling pathways and time course trajectories to be carried out in a practical time.
Availability and implementation: Source code is available at http://guanlab.ccmb.med.umich.edu/DREAM/code.html and as supplementary file online.
© The Author 2014. Published by Oxford University Press. All rights reserved. For Permissions, please e-mail: journals.permissions@oup.com.
Figures
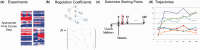
Workflow of time course prediction of the network trajectory under unseen perturbations. (a) Time course dynamic phosphorylation levels under several observed perturbations are collected. (b) Regression coefficient matrix representing the influence level (positive and negative) between proteins are calculated based on the observed data. (c) Starting phosphorylation levels of the proteins under unseen perturbation are estimated using observed data. (d) Regression coefficient matrix is adjusted according to the new perturbation and phosphorylation levels are predicted iteratively across a time course
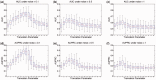
Accuracy of recovering the singaling networks. This figure shows AUC and AUPRC under different truncation parameters and noise levels, which indicate how well the regression coefficient matrix estimated by truncated SVD recovers the true influence matrix. The box plots are generated from 100 runs, with the density of non-zero elements in the signaling network to be 15%. A truncation parameter equals to N represents that the first N elements in diagonal matrix will be kept, while other elements are set to 0
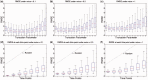
RMSE under different truncation parameters and noise levels. This figure indicates how truncation parameter influences the RMSE under different noise levels. Subfigures (a), (b) and (c) demonstrate the overall RMSE under different truncation parameters from 1 to 16 and different noise levels. Subfigures (d), (e) and (f) show the RMSE values at different time points under a truncation parameter of 8. The RMSE baseline of random prediction is also depicted. RMSE was evaluated under 100 repeated runs
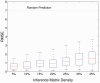
Influence of matrix densities. This figure illustrates the performance of the prediction method under different densities of the influence matrix. This result is evaluated under the low noise level (0.1) with a truncation parameter, which keeps eight largest elements in the diagonal matrix. The RMSE is evaluated from 100 runs
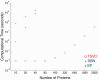
Computation time. This figure shows the computational time required to reconstruct the signaling network. The computational cost is evaluated under 100 repeated measurements. DBN and BP are only measured under 10, 20 and 40 proteins because of their exponential computational time required
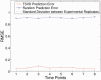
Predicting errors in experimental data. This figure illustrates the accuracy of this trajectory predicting method in recovering experimental data. The predicted time course trajectory (red line) has a much lower RMSE across all the time points compared with random prediction (blue line). Additionally, RMSE of the predicted trajectory (0.06) is comparable with the difference between replicates (0.015, black line), indicating robust performance of the algorithms
Similar articles
-
An algebra-based method for inferring gene regulatory networks.
Vera-Licona P, Jarrah A, Garcia-Puente LD, McGee J, Laubenbacher R. Vera-Licona P, et al. BMC Syst Biol. 2014 Mar 26;8:37. doi: 10.1186/1752-0509-8-37. BMC Syst Biol. 2014. PMID: 24669835 Free PMC article.
-
Towards a rigorous assessment of systems biology models: the DREAM3 challenges.
Prill RJ, Marbach D, Saez-Rodriguez J, Sorger PK, Alexopoulos LG, Xue X, Clarke ND, Altan-Bonnet G, Stolovitzky G. Prill RJ, et al. PLoS One. 2010 Feb 23;5(2):e9202. doi: 10.1371/journal.pone.0009202. PLoS One. 2010. PMID: 20186320 Free PMC article.
-
Modeling of Receptor Tyrosine Kinase Signaling: Computational and Experimental Protocols.
Fey D, Aksamitiene E, Kiyatkin A, Kholodenko BN. Fey D, et al. Methods Mol Biol. 2017;1636:417-453. doi: 10.1007/978-1-4939-7154-1_27. Methods Mol Biol. 2017. PMID: 28730495
-
Biological Network Inference and analysis using SEBINI and CABIN.
Taylor R, Singhal M. Taylor R, et al. Methods Mol Biol. 2009;541:551-76. doi: 10.1007/978-1-59745-243-4_24. Methods Mol Biol. 2009. PMID: 19381531 Review.
-
Assmann SM, Albert R. Assmann SM, et al. Methods Mol Biol. 2009;553:207-25. doi: 10.1007/978-1-60327-563-7_10. Methods Mol Biol. 2009. PMID: 19588107 Review.
Cited by
-
Zhu F, Shi L, Engel JD, Guan Y. Zhu F, et al. Bioinformatics. 2015 Aug 1;31(15):2537-44. doi: 10.1093/bioinformatics/btv186. Epub 2015 Apr 2. Bioinformatics. 2015. PMID: 25840044 Free PMC article.
-
Li HD, Omenn GS, Guan Y. Li HD, et al. Database (Oxford). 2015 May 7;2015:bav045. doi: 10.1093/database/bav045. Print 2015. Database (Oxford). 2015. PMID: 25953081 Free PMC article.
-
Li HD, Omenn GS, Guan Y. Li HD, et al. Brief Bioinform. 2016 Nov;17(6):1024-1031. doi: 10.1093/bib/bbv109. Epub 2016 Jan 6. Brief Bioinform. 2016. PMID: 26740460 Free PMC article.
-
Predicting Anticancer Drug Responses Using a Dual-Layer Integrated Cell Line-Drug Network Model.
Zhang N, Wang H, Fang Y, Wang J, Zheng X, Liu XS. Zhang N, et al. PLoS Comput Biol. 2015 Sep 29;11(9):e1004498. doi: 10.1371/journal.pcbi.1004498. eCollection 2015. PLoS Comput Biol. 2015. PMID: 26418249 Free PMC article.
-
Zhu F, Shi L, Li H, Eksi R, Engel JD, Guan Y. Zhu F, et al. Bioinformatics. 2014 Dec 1;30(23):3325-33. doi: 10.1093/bioinformatics/btu542. Epub 2014 Aug 12. Bioinformatics. 2014. PMID: 25115705 Free PMC article.
References
-
- Aebersold R, Mann M. Mass spectrometry-based proteomics. Nature. 2003;422:198–207. - PubMed
-
- Barrios-Rodiles M, et al. High-throughput mapping of a dynamic signaling network in mammalian cells. Science. 2005;307:1621–1625. - PubMed
-
- de Jong H, et al. Genetic network analyzer: qualitative simulation of genetic regulatory networks. Bioinformatics. 2003;19:336–344. - PubMed
-
- di Bernardo D, et al. Chemogenomic profiling on a genome-wide scale using reverse-engineered gene networks. Nat. Biotechnol. 2005;23:377–383. - PubMed
Publication types
MeSH terms
Substances
LinkOut - more resources
Full Text Sources
Other Literature Sources