Randomness and preserved patterns in cancer network - PubMed
- ️Wed Jan 01 2014
Randomness and preserved patterns in cancer network
Aparna Rai et al. Sci Rep. 2014.
Abstract
Breast cancer has been reported to account for the maximum cases among all female cancers till date. In order to gain a deeper insight into the complexities of the disease, we analyze the breast cancer network and its normal counterpart at the proteomic level. While the short range correlations in the eigenvalues exhibiting universality provide an evidence towards the importance of random connections in the underlying networks, the long range correlations along with the localization properties reveal insightful structural patterns involving functionally important proteins. The analysis provides a benchmark for designing drugs which can target a subgraph instead of individual proteins.
Conflict of interest statement
The authors declare no competing financial interests.
Figures
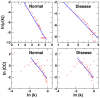
Left panel of the normal network show that the degree distribution follows power law and the degree clustering coefficient correlation shows are negatively correlated. The right panel gives us the same results for the disease networks.
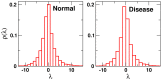
The plots depict triangular distribution for both the networks with a high degeneracy at zero.
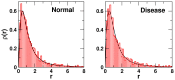
The ratio of eigenvalues spacing follow GOE statistics for both the networks. The bars represent data points and solid line represents Eq.4 with parameters of GOE statistics.
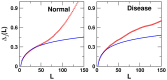
Circles denote data points for the normal and the disease networks whereas the solid line is the Δ3 statistics for the GOE.
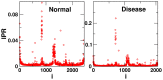
They clearly reflect three regions (i) degenerate part in the middle, (ii) a large non-degenerate part which follow GOE statistics of RMT and non-degenerate part at both the end and near to the zero eigenvalues which deviate from RMT.
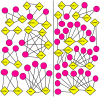
(Left panel) The local structure of all TCNs in the disease network. (Right panel) The local structure for the same proteins in the normal network. Yellow represents TCNs and pink represent their first neighbor.
Similar articles
-
Increased entropy of signal transduction in the cancer metastasis phenotype.
Teschendorff AE, Severini S. Teschendorff AE, et al. BMC Syst Biol. 2010 Jul 30;4:104. doi: 10.1186/1752-0509-4-104. BMC Syst Biol. 2010. PMID: 20673354 Free PMC article.
-
Two-layer modular analysis of gene and protein networks in breast cancer.
Srivastava A, Kumar S, Ramaswamy R. Srivastava A, et al. BMC Syst Biol. 2014 Jul 5;8:81. doi: 10.1186/1752-0509-8-81. BMC Syst Biol. 2014. PMID: 24997799 Free PMC article.
-
Jo K, Santos-Buitrago B, Kim M, Rhee S, Talcott C, Kim S. Jo K, et al. Methods. 2020 Jul 1;179:89-100. doi: 10.1016/j.ymeth.2020.05.008. Epub 2020 May 20. Methods. 2020. PMID: 32445696
-
Chiquet J, Rigaill G, Sundqvist M. Chiquet J, et al. Methods Mol Biol. 2019;1883:143-160. doi: 10.1007/978-1-4939-8882-2_6. Methods Mol Biol. 2019. PMID: 30547399
-
Network controllability solutions for computational drug repurposing using genetic algorithms.
Popescu VB, Kanhaiya K, Năstac DI, Czeizler E, Petre I. Popescu VB, et al. Sci Rep. 2022 Jan 26;12(1):1437. doi: 10.1038/s41598-022-05335-3. Sci Rep. 2022. PMID: 35082323 Free PMC article.
Cited by
-
Understanding cancer complexome using networks, spectral graph theory and multilayer framework.
Rai A, Pradhan P, Nagraj J, Lohitesh K, Chowdhury R, Jalan S. Rai A, et al. Sci Rep. 2017 Feb 3;7:41676. doi: 10.1038/srep41676. Sci Rep. 2017. PMID: 28155908 Free PMC article.
-
Gladilin E. Gladilin E. PLoS One. 2017 Jan 31;12(1):e0170953. doi: 10.1371/journal.pone.0170953. eCollection 2017. PLoS One. 2017. PMID: 28141819 Free PMC article.
-
The Human Body as a Super Network: Digital Methods to Analyze the Propagation of Aging.
Whitwell HJ, Bacalini MG, Blyuss O, Chen S, Garagnani P, Gordleeva SY, Jalan S, Ivanchenko M, Kanakov O, Kustikova V, Mariño IP, Meyerov I, Ullner E, Franceschi C, Zaikin A. Whitwell HJ, et al. Front Aging Neurosci. 2020 May 25;12:136. doi: 10.3389/fnagi.2020.00136. eCollection 2020. Front Aging Neurosci. 2020. PMID: 32523526 Free PMC article.
-
Network spectra for drug-target identification in complex diseases: new guns against old foes.
Rai A, Shinde P, Jalan S. Rai A, et al. Appl Netw Sci. 2018;3(1):51. doi: 10.1007/s41109-018-0107-y. Epub 2018 Dec 17. Appl Netw Sci. 2018. PMID: 30596144 Free PMC article. Review.
-
Random Matrix Analysis for Gene Interaction Networks in Cancer Cells.
Kikkawa A. Kikkawa A. Sci Rep. 2018 Jul 13;8(1):10607. doi: 10.1038/s41598-018-28954-1. Sci Rep. 2018. PMID: 30006574 Free PMC article.
References
-
- Venter J. C. et al. The Sequence of the Human Genome. Science 16, 1304–1351 (2001). - PubMed
-
- Ferlay J., Parkin D. M. & Fouchera E. S. Estimates of cancer incidence and mortality in Europe in 2008. EJC. 46, 765–781 (2010). - PubMed
-
- Kiberstis P. & Roberts L. A Race Still Unfinished. Science 343, 1451–1470 (2014). - PubMed
-
- Hanahan D. & Weinberg R. A. The Hallmarks of Cancer. Cell 100, 57–70 (2000). - PubMed
-
- Petricoin E. F. et al. Mapping Molecular Networks Using Proteomics: A Vision for Patient-Tailored Combination Therapy. J. Clin. Oncol. 23, 3614–3621 (2005). - PubMed
Publication types
MeSH terms
LinkOut - more resources
Full Text Sources
Other Literature Sources
Medical