A Bayesian framework for de novo mutation calling in parents-offspring trios - PubMed
- ️Thu Jan 01 2015
A Bayesian framework for de novo mutation calling in parents-offspring trios
Qiang Wei et al. Bioinformatics. 2015.
Abstract
Motivation: Spontaneous (de novo) mutations play an important role in the disease etiology of a range of complex diseases. Identifying de novo mutations (DNMs) in sporadic cases provides an effective strategy to find genes or genomic regions implicated in the genetics of disease. High-throughput next-generation sequencing enables genome- or exome-wide detection of DNMs by sequencing parents-proband trios. It is challenging to sift true mutations through massive amount of noise due to sequencing error and alignment artifacts. One of the critical limitations of existing methods is that for all genomic regions the same pre-specified mutation rate is assumed, which has a significant impact on the DNM calling accuracy.
Results: In this study, we developed and implemented a novel Bayesian framework for DNM calling in trios (TrioDeNovo), which overcomes these limitations by disentangling prior mutation rates from evaluation of the likelihood of the data so that flexible priors can be adjusted post-hoc at different genomic sites. Through extensively simulations and application to real data we showed that this new method has improved sensitivity and specificity over existing methods, and provides a flexible framework to further improve the efficiency by incorporating proper priors. The accuracy is further improved using effective filtering based on sequence alignment characteristics.
Availability and implementation: The C++ source code implementing TrioDeNovo is freely available at https://medschool.vanderbilt.edu/cgg.
Contact: bingshan.li@vanderbilt.edu
Supplementary information: Supplementary data are available at Bioinformatics online.
© The Author 2014. Published by Oxford University Press. All rights reserved. For Permissions, please e-mail: journals.permissions@oup.com.
Figures
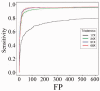
ROC curves of de novo SNV mutations called by TrioDeNovo in simulated datasets with different coverage. Sensitivity and false positive rates were calculated for sequencing coverage of 17× (black), 34× (green), 51× (blue) and 68× (red) with flat prior odds for all candidates (color version of this figure is available at Bioinformatics online.)
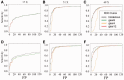
Comparison of ROC curves of de novo SNV mutations called by TrioDeNovo and DeNovoGear in the simulated datasets with coverage of 17X (A, D), 51X (B, E) and 68X (C, F). (A–C) The ROC curves calculated based on data simulated with the same mutation rate, and (D-F) the corresponding ROC curves with different prior mutation rates. Black lines represent TrioDeNovo calls with appropriate prior odds. Green, orange and red lines represent DeNovoGear calls with specified mutation rates of 10−8 (default), 10−4 and 10−12, respectively (color version of this figure is available at Bioinformatics online.)
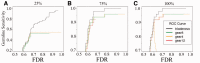
ROC curves of de novo germline SNV mutations called by TrioDeNovo and DeNovoGear in the 1000GP CEU trio data with different coverage. ROC curves were calculated on datasets with 25% (A), 75% (B) and 100% (C) of the original whole genome data. Black lines represent TrioDeNovo calls with flat prior odds. Green, orange and red lines represent DeNovoGear calls with specified mutation rates of 10−8 (default), 10−4 and 10−12, respectively (color version of this figure is available at Bioinformatics online.)
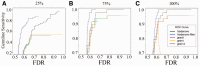
ROC curves of de novo germline SNV mutations called by TrioDeNovo and DeNovoGear in the 1000G CEU trio filtered using DNMfilter. Blue lines represent the ROC curves of the TrioDeNovo calls after applying DNMFilter and other lines are the same as those in Figure 3 (color version of this figure is available at Bioinformatics online.)
Similar articles
-
Li J, Jiang Y, Wang T, Chen H, Xie Q, Shao Q, Ran X, Xia K, Sun ZS, Wu J. Li J, et al. J Med Genet. 2015 Apr;52(4):275-81. doi: 10.1136/jmedgenet-2014-102656. Epub 2015 Jan 16. J Med Genet. 2015. PMID: 25596308
-
TotalReCaller: improved accuracy and performance via integrated alignment and base-calling.
Menges F, Narzisi G, Mishra B. Menges F, et al. Bioinformatics. 2011 Sep 1;27(17):2330-7. doi: 10.1093/bioinformatics/btr393. Epub 2011 Jun 30. Bioinformatics. 2011. PMID: 21724593
-
Yang H, Wei Q, Zhong X, Yang H, Li B. Yang H, et al. Bioinformatics. 2017 Feb 15;33(4):483-490. doi: 10.1093/bioinformatics/btw662. Bioinformatics. 2017. PMID: 27797769 Free PMC article.
-
Jin ZB, Li Z, Liu Z, Jiang Y, Cai XB, Wu J. Jin ZB, et al. Biol Rev Camb Philos Soc. 2018 May;93(2):1014-1031. doi: 10.1111/brv.12383. Epub 2017 Nov 20. Biol Rev Camb Philos Soc. 2018. PMID: 29154454 Review.
-
Review of alignment and SNP calling algorithms for next-generation sequencing data.
Mielczarek M, Szyda J. Mielczarek M, et al. J Appl Genet. 2016 Feb;57(1):71-9. doi: 10.1007/s13353-015-0292-7. Epub 2015 Jun 9. J Appl Genet. 2016. PMID: 26055432 Review.
Cited by
-
Lian Q, Chen Y, Chang F, Fu Y, Qi J. Lian Q, et al. Genomics Proteomics Bioinformatics. 2022 Jun;20(3):524-535. doi: 10.1016/j.gpb.2019.11.014. Epub 2021 Mar 10. Genomics Proteomics Bioinformatics. 2022. PMID: 33711466 Free PMC article.
-
Haplotyping-Assisted Diploid Assembly and Variant Detection with Linked Reads.
Hu Y, Yang C, Zhang L, Zhou X. Hu Y, et al. Methods Mol Biol. 2023;2590:161-182. doi: 10.1007/978-1-0716-2819-5_11. Methods Mol Biol. 2023. PMID: 36335499 Review.
-
Contributions of de novo variants to systemic lupus erythematosus.
Almlöf JC, Nystedt S, Mechtidou A, Leonard D, Eloranta ML, Grosso G, Sjöwall C, Bengtsson AA, Jönsen A, Gunnarsson I, Svenungsson E, Rönnblom L, Sandling JK, Syvänen AC. Almlöf JC, et al. Eur J Hum Genet. 2021 Jan;29(1):184-193. doi: 10.1038/s41431-020-0698-5. Epub 2020 Jul 28. Eur J Hum Genet. 2021. PMID: 32724065 Free PMC article.
-
Abu-Khalaf M, Wang C, Zhang Z, Luo R, Chong W, Silver DP, Fellin F, Jaslow R, Lopez A, Cescon T, Jiang W, Myers R, Wei Q, Li B, Cristofanilli M, Yang H. Abu-Khalaf M, et al. Cancers (Basel). 2022 Jun 10;14(12):2872. doi: 10.3390/cancers14122872. Cancers (Basel). 2022. PMID: 35740538 Free PMC article.
-
De novo variants in exomes of congenital heart disease patients identify risk genes and pathways.
Sevim Bayrak C, Zhang P, Tristani-Firouzi M, Gelb BD, Itan Y. Sevim Bayrak C, et al. Genome Med. 2020 Jan 15;12(1):9. doi: 10.1186/s13073-019-0709-8. Genome Med. 2020. PMID: 31941532 Free PMC article.
References
Publication types
MeSH terms
Grants and funding
LinkOut - more resources
Full Text Sources
Other Literature Sources