Cultivating healthy growth and nutrition through the gut microbiota - PubMed
- ️Thu Jan 01 2015
Cultivating healthy growth and nutrition through the gut microbiota
Sathish Subramanian et al. Cell. 2015.
Abstract
Microbiota assembly is perturbed in children with undernutrition, resulting in persistent microbiota immaturity that is not rescued by current nutritional interventions. Evidence is accumulating that this immaturity is causally related to the pathogenesis of undernutrition and its lingering sequelae. Preclinical models in which human gut communities are replicated in gnotobiotic mice have provided an opportunity to identify and predict the effects of different dietary ingredients on microbiota structure, expressed functions, and host biology. This capacity sets the stage for proof-of-concept tests designed to deliberately shape the developmental trajectory and configurations of microbiota in children representing different geographies, cultural traditions, and states of health. Developing these capabilities for microbial stewardship is timely given the global health burden of childhood undernutrition, the effects of changing eating practices brought about by globalization, and the realization that affordable nutritious foods need to be developed to enhance our capacity to cultivate healthier microbiota in populations at risk for poor nutrition.
Copyright © 2015 Elsevier Inc. All rights reserved.
Figures
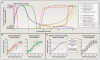
(A) Bacterial taxa that discriminate different stages of development were identified by a machine learning-based (Random Forests) regression of 16S rRNA data sets produced from monthly fecal samples collected from anthropometrically healthy infants and children living in an urban slum in Dhaka, Bangladesh during their first 2 years of postnatal life to their respective chronologic ages at the time of sample collection (Subramanian et. al, 2014). Shown are depictions of the typical distributions of these age-discriminatory taxa across the population. Taxa were selected based on their relative importance to the accuracy of the Random Forests model using a permutation-based “feature importance.” (B) The most discriminatory taxa, as defined by their feature importance, were used as inputs into a sparse 24 taxon model whose output (“microbiota age”) is a microbiota-based prediction of the chronologic age of a healthy child. The plot on the left of the panel shows microbiota age against chronologic age of healthy children used as a training set to fit the regression (each dot is a fecal sample from an individual child). The plot on the right of the panel shows application of the sparse model to a validation set composed of a different group of children living in the same location that were not used to train the model. Applying the model to a separate validation set controls for over-fitting of the model to the training set and ensures its wider usability. (C) Two metrics of microbiota maturation based on application of the model to two separate validation sets of singletons and a separate study of Bangladeshi twins/triplets. “Relative microbiota maturity” is the deviation, in months, from a smooth-spline fit of microbiota age values with respect to chronologic age, fitted using the validation data sets (see black dashed curve). The red dot represents a fecal sample collected from a focus child that is 11 months below the spline fit, indicating negative relative microbiota maturity (i.e., an immature microbiota). A MAZ is computed by dividing the difference between the focal child’s microbiota age and the median microbiota age of healthy controls in the same monthly chronologic age bin over the SD within the same age bin. The median and SD of each bin are computed using the validation data sets. The distribution of microbiota maturity and MAZ scores in birth-cohort studies have been studied using linear mixed models that take into account random variation specific to each serially sampled child and family while estimating the fixed variation attributable to a factor observed across different children (e.g., diarrheal episodes) (Subramanian et al., 2014). Note that using Random Forests to study microbiota maturation is advantageous because of its non-parametric assumptions and utility in the context of high dimensional data sets (large numbers of predictors). Nonetheless, it is one of several methods that can be useful. For example, the rank-order Spearman correlation metric has been applied to infant microbiome data sets to detect monotonic relationships between microbiome-encoded functions/bacterial taxa and postnatal age (Yatsunenko et. al, 2012).
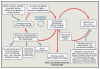
The discovery process depicted by the left circle illustrates how gnotobiotic animal models colonized with human donor microbiota and fed human diets can lead to a greater understanding of how diet-by-microbiota interactions are causally related to healthy growth and to phenotypes associated with undernutrition: e.g., immune system development, brain development, and host and microbial community metabolism. New surrogate- or mechanism-based biomarkers of nutritional state emanating from these gnotobiotic models can be validated using biospecimens collected from the donors used to construct these gnotobiotic models, as well as from other members of the study population. The discovery/development process depicted on the right illustrates how dietary and microbial “leads” can be tested in the context of humanized gnotobiotic animals to assess how they modulate biological processes already known, discovered, or postulated to be involved in healthy growth and/or the pathogenesis of undernutrition. The downward-pointing arrow in the middle of the figure points to next steps in clinical translation. See the main text for a discussion of the regulatory, ethical, societal, and commercial implications of these efforts. Abbreviation: IND, investigational new drug.
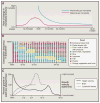
(A) Illustration of the rate of change occurring in gut microbiota structure of both mother and child. Note that infant variation curves are known from both longitudinal and cross-sectional study designs (Yatsunenko et al., 2012; Subramanian et al., 2014). In the case of mothers, the curve is interpolated based on studies of pregnant Finnish mothers prior to delivery (Koren et al., 2012) and Bangladeshi mothers following parturition (Subramanian et al., 2014). (B) The food consumption pattern shown is at a population level and does not depict the great deal of temporal variation observed in food consumption patterns within a given child. Depicting the fractional contribution of each food to the consumption patterns of children in Bangladesh underscores how dietary changes occur simultaneously (lowering of breast milk and increase in legumes and cow’s milk) and not in an orderly fashion (small fluctuations from month to month; re-entry and dropout of certain foods). It also underscores the challenge encountered in ascertaining how food and the microbiota interact to effect maturation of the community. (C) Major processes related to growth and how they vary in rate and magnitude over time. Curves are adapted from Bogin (1999). Note that the newborn brain represents 12% of body weight (a value 6 times greater than in adults). By the end of the first decade, the brain represents 6% of body weight and consumes twice the amount of glucose and 1.5 times the amount of oxygen as the adult brain. Approximately 30% of the glucose consumed by the infant brain is accounted for by aerobic glycolysis (versus 12% in adults) (Goyal et al., 2014). The dramatic changes in brain metabolism that occur over the first two decades of life coincide with the initial proliferation and then pruning of synapses to adult levels. Central questions that need to be addressed in this area include the biological effects of the gut microbial community on neurogenesis, synaptic connectivity, gliogenesis and glial-neuron interactions, neural circuit function and higher cognitive processes in the context of healthy growth versus undernutrition, and whether/how the gut-brain axis operates to influence/regulate other aspects of host physiology, metabolism, and immunity in the infant/child. Moreover, if persistent immaturity of the gut microbiota is causally related to undernutrition and its long-term sequelae, including neurodevelopmental abnormalities, does durable repair of this immaturity require that nutritional interventions be administered earlier before disease becomes fully manifest (and the microbial ecosystem is so perturbed that restoration becomes very difficult)? Do nutritional interventions need to be applied for more sustained periods of time? Do new types of therapeutic foods need to be developed, or is a microbial intervention also needed?
Similar articles
-
Ahmed T, Auble D, Berkley JA, Black R, Ahern PP, Hossain M, Hsieh A, Ireen S, Arabi M, Gordon JI. Ahmed T, et al. Ann N Y Acad Sci. 2014 Dec;1332(1):22-38. doi: 10.1111/nyas.12487. Epub 2014 Aug 12. Ann N Y Acad Sci. 2014. PMID: 25118072 Free PMC article. Review.
-
Of the bugs that shape us: maternal obesity, the gut microbiome, and long-term disease risk.
Gohir W, Ratcliffe EM, Sloboda DM. Gohir W, et al. Pediatr Res. 2015 Jan;77(1-2):196-204. doi: 10.1038/pr.2014.169. Epub 2014 Oct 14. Pediatr Res. 2015. PMID: 25314580 Review.
-
Prebiotic effects: metabolic and health benefits.
Roberfroid M, Gibson GR, Hoyles L, McCartney AL, Rastall R, Rowland I, Wolvers D, Watzl B, Szajewska H, Stahl B, Guarner F, Respondek F, Whelan K, Coxam V, Davicco MJ, Léotoing L, Wittrant Y, Delzenne NM, Cani PD, Neyrinck AM, Meheust A. Roberfroid M, et al. Br J Nutr. 2010 Aug;104 Suppl 2:S1-63. doi: 10.1017/S0007114510003363. Br J Nutr. 2010. PMID: 20920376 Review.
-
Persistent gut microbiota immaturity in malnourished Bangladeshi children.
Subramanian S, Huq S, Yatsunenko T, Haque R, Mahfuz M, Alam MA, Benezra A, DeStefano J, Meier MF, Muegge BD, Barratt MJ, VanArendonk LG, Zhang Q, Province MA, Petri WA Jr, Ahmed T, Gordon JI. Subramanian S, et al. Nature. 2014 Jun 19;510(7505):417-21. doi: 10.1038/nature13421. Epub 2014 Jun 4. Nature. 2014. PMID: 24896187 Free PMC article.
-
Putignani L, Del Chierico F, Petrucca A, Vernocchi P, Dallapiccola B. Putignani L, et al. Pediatr Res. 2014 Jul;76(1):2-10. doi: 10.1038/pr.2014.49. Epub 2014 Apr 14. Pediatr Res. 2014. PMID: 24732106 Review.
Cited by
-
Malmuthuge N, Liang G, Guan LL. Malmuthuge N, et al. Genome Biol. 2019 Aug 23;20(1):172. doi: 10.1186/s13059-019-1786-0. Genome Biol. 2019. PMID: 31443695 Free PMC article.
-
Kumar M, Ji B, Babaei P, Das P, Lappa D, Ramakrishnan G, Fox TE, Haque R, Petri WA, Bäckhed F, Nielsen J. Kumar M, et al. Metab Eng. 2018 Sep;49:128-142. doi: 10.1016/j.ymben.2018.07.018. Epub 2018 Jul 31. Metab Eng. 2018. PMID: 30075203 Free PMC article.
-
Iwase SC, Jaspan HB, Happel AU, Holmes SP, Abimiku A, Osawe S, Gray CM, Blackburn JM. Iwase SC, et al. Res Sq [Preprint]. 2023 Jul 3:rs.3.rs-3112263. doi: 10.21203/rs.3.rs-3112263/v1. Res Sq. 2023. PMID: 37461449 Free PMC article. Updated. Preprint.
-
Bozorgmehr T, Boutin RCT, Woodward SE, Donald K, Chow JM, Buck RH, Finlay BB. Bozorgmehr T, et al. J Immunol Res. 2023 Jul 29;2023:9603576. doi: 10.1155/2023/9603576. eCollection 2023. J Immunol Res. 2023. PMID: 37545544 Free PMC article.
-
Innate and adaptive lymphocytes sequentially shape the gut microbiota and lipid metabolism.
Mao K, Baptista AP, Tamoutounour S, Zhuang L, Bouladoux N, Martins AJ, Huang Y, Gerner MY, Belkaid Y, Germain RN. Mao K, et al. Nature. 2018 Feb 8;554(7691):255-259. doi: 10.1038/nature25437. Epub 2018 Jan 22. Nature. 2018. PMID: 29364878
References
Publication types
MeSH terms
Grants and funding
LinkOut - more resources
Full Text Sources
Other Literature Sources
Medical