Delayed feedback model of axonal length sensing - PubMed
- ️Thu Jan 01 2015
Delayed feedback model of axonal length sensing
Bhargav R Karamched et al. Biophys J. 2015.
Abstract
A fundamental question in cell biology is how the sizes of cells and organelles are regulated at various stages of development. Size homeostasis is particularly challenging for neurons, whose axons can extend from hundreds of microns to meters (in humans). Recently, a molecular-motor-based mechanism for axonal length sensing has been proposed, in which axonal length is encoded by the frequency of an oscillating retrograde signal. In this article, we develop a mathematical model of this length-sensing mechanism in which advection-diffusion equations for bidirectional motor transport are coupled to a chemical signaling network. We show that chemical oscillations emerge due to delayed negative feedback via a Hopf bifurcation, resulting in a frequency that is a monotonically decreasing function of axonal length. Knockdown of either kinesin or dynein causes an increase in the oscillation frequency, suggesting that the length-sensing mechanism would produce longer axons, which is consistent with experimental findings. One major prediction of the model is that fluctuations in the transport of molecular motors lead to a reduction in the reliability of the frequency-encoding mechanism for long axons.
Copyright © 2015 Biophysical Society. Published by Elsevier Inc. All rights reserved.
Figures
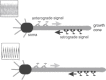
Schematic diagram of the bidirectional motor-transport mechanism for axonal length sensing hypothesized by Rishal et al. (27). A kinesin-based anterograde signal activates a dynein-based retrograde signal that itself represses the anterograde signal via negative feedback. The frequency of the resulting oscillatory retrograde signal decreases with axonal growth.
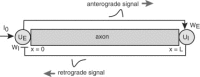
Schematic diagram of the feedback model. See text for details.
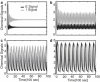
Chemical signal oscillations in the delayed-feedback model given by Eq. 1 for various values of the delay (in units of 100 s): τ = 0.2 (a); τ = 0.29 (b); τ = 0.75 (c); and τ = 1.5 (d). Other parameter values are n = 4, I0 = 6, WE = WI = 5.5 such that τc ≈ 0.25.
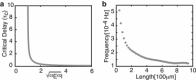
(a) Plot of critical delay τc as a function of the effective coupling parameter αEαI. Both are in units of 100 s. (b) Frequency of periodic solutions plotted against axonal delay. Parameter values are as in Fig. 3.
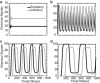
Chemical signal oscillations in the advection-diffusion model described by Eq. 7 for various axonal lengths: L = 100 μm (a); L = 500 μm (b); L = 1000 μm (c); and L = 2000 μm (d). Other parameter values are I0 = 10, wE = wI = 9, γ = 1, κE = κI = 1, v = 1 μm s−1, and D = 0.1 μm2 s−1.
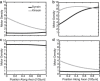
Spatial profiles for kinesin and dynein motors at different times during one cycle of period T = 2π/ω after transients have disappeared. (a) t = 0. (b) t = T/4. (c) t = T/2. (d) t = 3T/4. The initial condition for the kinesin motors is a hyperbolic secant function, whereas the initial condition for the dynein motors is zero. Here, L = 100 μm and other parameters are as in Fig. 5.
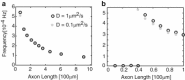
Variation in frequency as a function of length for different diffusivities in the advection-diffusion model. Other parameters are the same as in Fig. 5. (a) Plot showing that the frequency is insensitive to D except at small axonal lengths. (b) Plot showing that the main effect of diffusivity is to modify the critical length at which a Hopf bifurcation occurs. (A zero frequency indicates that the system is operating below the Hopf bifurcation point, so there are no oscillations.)
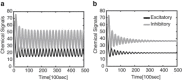
Chemical signals in the advection-diffusion model for D = 0.1 μm2 s−1 (a) and D = 100 μm2 s−1 (b). Here, L = 500 μm and other parameter values are as in Fig. 5.
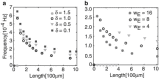
Variation in frequency as a function of length for decreasing flux δ ≡ I0 − wI (a) (representing knockdown of kinesin) and decreasing excitatory coupling, wE (b) (representing knockdown of dynein). Other parameters are the same as Fig. 5.
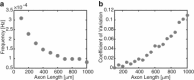
Effects of noise on the frequency-encoding mechanism for axonal length sensing in the computational model of Rishal et al. (27) with modified end conditions (see text for details). (a) Average frequency versus axonal length. (b) Coefficient of variation versus axonal length. The plots were obtained by running the simulations over 100 trials.
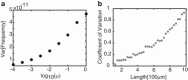
Effects of noise on the frequency-encoding mechanism for axonal length sensing in the stochastic advection-diffusion model. (a) Variance in oscillation frequency (determined by the peak of the power spectrum) versus noise strength μ. (b) Variance in frequency versus axonal length. The plots were obtained by solving the stochastic partial differential equations over 1000 trials with μ = 1.
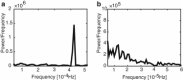
Power spectra of the retrograde chemical signal generated by the stochastic advection-diffusion model of Eq. 25 for (a) L = 100 μm and (b) L = 1000 μm. Other parameters are as in Fig. 5.
Similar articles
-
A frequency-dependent decoding mechanism for axonal length sensing.
Bressloff PC, Karamched BR. Bressloff PC, et al. Front Cell Neurosci. 2015 Jul 21;9:281. doi: 10.3389/fncel.2015.00281. eCollection 2015. Front Cell Neurosci. 2015. PMID: 26257607 Free PMC article.
-
Dolma K, Iacobucci GJ, Hong Zheng K, Shandilya J, Toska E, White JA 2nd, Spina E, Gunawardena S. Dolma K, et al. Hum Mol Genet. 2014 Mar 1;23(5):1121-33. doi: 10.1093/hmg/ddt505. Epub 2013 Oct 8. Hum Mol Genet. 2014. PMID: 24105467
-
Zhang Z, Wu S, Jonas JB, Zhang J, Liu K, Lu Q, Wang N. Zhang Z, et al. Acta Ophthalmol. 2016 May;94(3):266-75. doi: 10.1111/aos.12768. Epub 2015 Jul 14. Acta Ophthalmol. 2016. PMID: 26178710
-
Molecular motors and mechanisms of directional transport in neurons.
Hirokawa N, Takemura R. Hirokawa N, et al. Nat Rev Neurosci. 2005 Mar;6(3):201-14. doi: 10.1038/nrn1624. Nat Rev Neurosci. 2005. PMID: 15711600 Review.
Cited by
-
Stochastic switching of delayed feedback suppresses oscillations in genetic regulatory systems.
Karamched BR, Miles CE. Karamched BR, et al. J R Soc Interface. 2023 Jun;20(203):20230059. doi: 10.1098/rsif.2023.0059. Epub 2023 Jun 28. J R Soc Interface. 2023. PMID: 37376870 Free PMC article.
-
Delay-induced uncertainty for a paradigmatic glucose-insulin model.
Karamched B, Hripcsak G, Albers D, Ott W. Karamched B, et al. Chaos. 2021 Feb;31(2):023142. doi: 10.1063/5.0027682. Chaos. 2021. PMID: 33653035 Free PMC article.
-
A frequency-dependent decoding mechanism for axonal length sensing.
Bressloff PC, Karamched BR. Bressloff PC, et al. Front Cell Neurosci. 2015 Jul 21;9:281. doi: 10.3389/fncel.2015.00281. eCollection 2015. Front Cell Neurosci. 2015. PMID: 26257607 Free PMC article.
References
-
- Rafelski S.M., Marshall W.F. Building the cell: design principles of cellular architecture. Nat. Rev. Mol. Cell Biol. 2008;9:593–602. - PubMed
-
- Marshall W.F. Cellular length control systems. Annu. Rev. Cell Dev. Biol. 2004;20:677–693. - PubMed
-
- Katsura I. Determination of bacteriophage λ tail length by a protein ruler. Nature. 1987;327:73–75. - PubMed
-
- Lefebvre P.A., Rosenbaum J.L. Regulation of the synthesis and assembly of ciliary and flagellar proteins during regeneration. Annu. Rev. Cell Biol. 1986;2:517–546. - PubMed
-
- Stephens R.E. Quantal tektin synthesis and ciliary length in sea-urchin embryos. J. Cell Sci. 1989;92:403–413. - PubMed
Publication types
MeSH terms
Substances
LinkOut - more resources
Full Text Sources
Other Literature Sources