Scaling laws predict global microbial diversity - PubMed
- ️Fri Jan 01 2016
Scaling laws predict global microbial diversity
Kenneth J Locey et al. Proc Natl Acad Sci U S A. 2016.
Abstract
Scaling laws underpin unifying theories of biodiversity and are among the most predictively powerful relationships in biology. However, scaling laws developed for plants and animals often go untested or fail to hold for microorganisms. As a result, it is unclear whether scaling laws of biodiversity will span evolutionarily distant domains of life that encompass all modes of metabolism and scales of abundance. Using a global-scale compilation of ∼35,000 sites and ∼5.6⋅10(6) species, including the largest ever inventory of high-throughput molecular data and one of the largest compilations of plant and animal community data, we show similar rates of scaling in commonness and rarity across microorganisms and macroscopic plants and animals. We document a universal dominance scaling law that holds across 30 orders of magnitude, an unprecedented expanse that predicts the abundance of dominant ocean bacteria. In combining this scaling law with the lognormal model of biodiversity, we predict that Earth is home to upward of 1 trillion (10(12)) microbial species. Microbial biodiversity seems greater than ever anticipated yet predictable from the smallest to the largest microbiome.
Keywords: biodiversity; macroecology; microbiology; microbiome; rare biosphere.
Conflict of interest statement
The authors declare no conflict of interest.
Figures

Microbial communities (blue dots) and communities of macroscopic plants and animals (red dots) are similar in the rates at which rarity, absolute dominance, and species evenness scale with the number of individuals or genes reads (N). However, for a given N, microbial communities have greater rarity, less evenness, and greater richness than those of macroorganisms. Coefficients and exponents of scaling equations are mean values from 10,000 bootstrapped multiple regressions, with each regression based on 500 microbial and 500 macrobial communities chosen by stratified random sampling. Each scatterplot represents a single random sample; hulls are 95% confidence intervals.
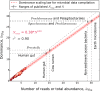
The dominance-abundance scaling law (dashed red line) predicts the abundance of the most abundant microbial taxa (Nmax) up to global scales. The pink hull is the 95% prediction interval for the regression based on 3,000 sites chosen by stratified random sampling (red heat map) from our microbial data compilation. Gray cross-hairs are ranges of published estimates of N and Nmax for large microbiomes, including Earth (6, 7, 31, 32) (Materials and Methods, Approximating Ranges of Nmax for Large Microbiomes). The light-gray dashed line is the 1:1 relationship. The scaling equation and r2 only pertain to the scatterplot data.
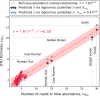
The microbial richness-abundance scaling relationship (dashed red line) supports values of S predicted from the lognormal model using the published ranges of N and Nmax (gray dots) as well as ranges of Nmax predicted from the dominance scaling law (blue dots). The pink hull is the 95% prediction interval for the regression based on 3,000 sites chosen by stratified random sampling (red scatterplot). The scaling equation and r2 value are based solely on the red scatterplot data. SEs around predicted S are too small to illustrate.
Comment in
-
The vast unknown microbial biosphere.
Pedrós-Alió C, Manrubia S. Pedrós-Alió C, et al. Proc Natl Acad Sci U S A. 2016 Jun 14;113(24):6585-7. doi: 10.1073/pnas.1606105113. Epub 2016 Jun 2. Proc Natl Acad Sci U S A. 2016. PMID: 27302946 Free PMC article. No abstract available.
-
Extrapolating abundance curves has no predictive power for estimating microbial biodiversity.
Willis A. Willis A. Proc Natl Acad Sci U S A. 2016 Aug 30;113(35):E5096. doi: 10.1073/pnas.1608281113. Epub 2016 Aug 10. Proc Natl Acad Sci U S A. 2016. PMID: 27512033 Free PMC article. No abstract available.
Similar articles
-
Depressing time: Waiting, melancholia, and the psychoanalytic practice of care.
Salisbury L, Baraitser L. Salisbury L, et al. In: Kirtsoglou E, Simpson B, editors. The Time of Anthropology: Studies of Contemporary Chronopolitics. Abingdon: Routledge; 2020. Chapter 5. In: Kirtsoglou E, Simpson B, editors. The Time of Anthropology: Studies of Contemporary Chronopolitics. Abingdon: Routledge; 2020. Chapter 5. PMID: 36137063 Free Books & Documents. Review.
-
Bryant A, Hiu S, Kunonga PT, Gajjar K, Craig D, Vale L, Winter-Roach BA, Elattar A, Naik R. Bryant A, et al. Cochrane Database Syst Rev. 2022 Sep 26;9(9):CD015048. doi: 10.1002/14651858.CD015048.pub2. Cochrane Database Syst Rev. 2022. PMID: 36161421 Free PMC article. Review.
-
Elwenspoek MM, Thom H, Sheppard AL, Keeney E, O'Donnell R, Jackson J, Roadevin C, Dawson S, Lane D, Stubbs J, Everitt H, Watson JC, Hay AD, Gillett P, Robins G, Jones HE, Mallett S, Whiting PF. Elwenspoek MM, et al. Health Technol Assess. 2022 Oct;26(44):1-310. doi: 10.3310/ZUCE8371. Health Technol Assess. 2022. PMID: 36321689 Free PMC article.
-
Enabling Systemic Identification and Functionality Profiling for Cdc42 Homeostatic Modulators.
Malasala S, Azimian F, Chen YH, Twiss JL, Boykin C, Akhtar SN, Lu Q. Malasala S, et al. bioRxiv [Preprint]. 2024 Jan 8:2024.01.05.574351. doi: 10.1101/2024.01.05.574351. bioRxiv. 2024. PMID: 38260445 Free PMC article. Updated. Preprint.
-
Triana L, Palacios Huatuco RM, Campilgio G, Liscano E. Triana L, et al. Aesthetic Plast Surg. 2024 Oct;48(20):4217-4227. doi: 10.1007/s00266-024-04260-2. Epub 2024 Aug 5. Aesthetic Plast Surg. 2024. PMID: 39103642 Review.
Cited by
-
Indoor microbiome and allergic diseases: From theoretical advances to prevention strategies.
Fu X, Ou Z, Sun Y. Fu X, et al. Eco Environ Health. 2022 Oct 17;1(3):133-146. doi: 10.1016/j.eehl.2022.09.002. eCollection 2022 Sep. Eco Environ Health. 2022. PMID: 38075599 Free PMC article. Review.
-
Microbiome definition re-visited: old concepts and new challenges.
Berg G, Rybakova D, Fischer D, Cernava T, Vergès MC, Charles T, Chen X, Cocolin L, Eversole K, Corral GH, Kazou M, Kinkel L, Lange L, Lima N, Loy A, Macklin JA, Maguin E, Mauchline T, McClure R, Mitter B, Ryan M, Sarand I, Smidt H, Schelkle B, Roume H, Kiran GS, Selvin J, Souza RSC, van Overbeek L, Singh BK, Wagner M, Walsh A, Sessitsch A, Schloter M. Berg G, et al. Microbiome. 2020 Jun 30;8(1):103. doi: 10.1186/s40168-020-00875-0. Microbiome. 2020. PMID: 32605663 Free PMC article.
-
Aligning the Measurement of Microbial Diversity with Macroecological Theory.
Stegen JC, Hurlbert AH, Bond-Lamberty B, Chen X, Anderson CG, Chu RK, Dini-Andreote F, Fansler SJ, Hess NJ, Tfaily M. Stegen JC, et al. Front Microbiol. 2016 Sep 23;7:1487. doi: 10.3389/fmicb.2016.01487. eCollection 2016. Front Microbiol. 2016. PMID: 27721808 Free PMC article.
-
Targeting Bacterial Genomes for Natural Product Discovery.
Kalkreuter E, Pan G, Cepeda AJ, Shen B. Kalkreuter E, et al. Trends Pharmacol Sci. 2020 Jan;41(1):13-26. doi: 10.1016/j.tips.2019.11.002. Epub 2019 Dec 7. Trends Pharmacol Sci. 2020. PMID: 31822352 Free PMC article. Review.
-
Emergence of Hierarchical Modularity in Evolving Networks Uncovered by Phylogenomic Analysis.
Caetano-Anollés G, Aziz MF, Mughal F, Gräter F, Koç I, Caetano-Anollés K, Caetano-Anollés D. Caetano-Anollés G, et al. Evol Bioinform Online. 2019 Sep 5;15:1176934319872980. doi: 10.1177/1176934319872980. eCollection 2019. Evol Bioinform Online. 2019. PMID: 31523127 Free PMC article. Review.
References
Publication types
MeSH terms
LinkOut - more resources
Full Text Sources
Other Literature Sources
Research Materials