Inadequate Reference Datasets Biased toward Short Non-epitopes Confound B-cell Epitope Prediction - PubMed
- ️Fri Jan 01 2016
Inadequate Reference Datasets Biased toward Short Non-epitopes Confound B-cell Epitope Prediction
Kh Shamsur Rahman et al. J Biol Chem. 2016.
Abstract
X-ray crystallography has shown that an antibody paratope typically binds 15-22 amino acids (aa) of an epitope, of which 2-5 randomly distributed amino acids contribute most of the binding energy. In contrast, researchers typically choose for B-cell epitope mapping short peptide antigens in antibody binding assays. Furthermore, short 6-11-aa epitopes, and in particular non-epitopes, are over-represented in published B-cell epitope datasets that are commonly used for development of B-cell epitope prediction approaches from protein antigen sequences. We hypothesized that such suboptimal length peptides result in weak antibody binding and cause false-negative results. We tested the influence of peptide antigen length on antibody binding by analyzing data on more than 900 peptides used for B-cell epitope mapping of immunodominant proteins of Chlamydia spp. We demonstrate that short 7-12-aa peptides of B-cell epitopes bind antibodies poorly; thus, epitope mapping with short peptide antigens falsely classifies many B-cell epitopes as non-epitopes. We also show in published datasets of confirmed epitopes and non-epitopes a direct correlation between length of peptide antigens and antibody binding. Elimination of short, ≤11-aa epitope/non-epitope sequences improved datasets for evaluation of in silico B-cell epitope prediction. Achieving up to 86% accuracy, protein disorder tendency is the best indicator of B-cell epitope regions for chlamydial and published datasets. For B-cell epitope prediction, the most effective approach is plotting disorder of protein sequences with the IUPred-L scale, followed by antibody reactivity testing of 16-30-aa peptides from peak regions. This strategy overcomes the well known inaccuracy of in silico B-cell epitope prediction from primary protein sequences.
Keywords: antibody; antigen; bioinformatics; epitope mapping; immunogenicity; protein motif; protein-protein interaction.
© 2016 by The American Society for Biochemistry and Molecular Biology, Inc.
Figures
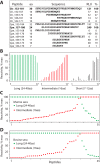
Peptide reactivity increases with length. A, elongation of peptides around the center of two epitopes increases the ELISA signal; RLU, relative light units/s, mean of six repeats; %, percent signal of maximally reactive peptide; Ctr, C. trachomatis; numbers indicate peptide position on the OmpA protein; Cpe; Chlamydia pecorum. B, relative signal from 17 epitopes in dependence on peptide antigen length. Peptides for 17 epitopes were extended toward the C and N terminus by 12–20 residues around the epitope center (long 24–40-aa peptides), 8 residues (intermediate 16-aa peptides), or 3–6 residues (short 7–12-aa peptides) and tested with the respective epitope-positive pooled hyperimmune mouse sera. Peptide reactivities are represented by vertical lines in the same order for long, intermediate, and short peptides. C, relative reactivity with murine antisera of corresponding long and intermediate peptides of 55 epitopes. D, relative reactivity with bovine antisera of corresponding long and intermediate peptides of 45 epitopes. Mapping of epitopes and peptide antigens used for Fig. 1 are described in the
supplemental Appendix.
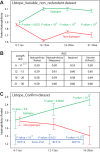
B-cell epitope prediction score and performance in dependence on peptide length. A, hydrophilicity scores of epitopes and non-epitopes are grouped by length in the Lbtope_Variable_non_redundant dataset (24). Hydrophilicity (Parker) (11) scores were obtained by using default settings in the ProtScale tools of the ExPASy server (29). Length-dependent hydrophilicity (±95% CI) of epitopes and non-epitopes is shown in green and red, respectively, and the p values for differences are shown in green. B, epitope length-dependent prediction performance (area under receiver operating characteristic curve) of different prediction scales in the Lbtope_Variable_non_redundant dataset. ***, p value <10−6 for comparison of any scale to any other scale of 6–11-aa epitopes versus longer epitopes. Hydrophobicity (Miyazawa, 30), a ProtScale (29) for hydrophobicity; Bepipred, a hidden Markov model combined with the Parker hydrophilicity scale (19); IUPred-L, an algorithm for protein disorder tendency (31). C, all 6–20-aa epitopes and non-epitopes of the Lbtope_Confirm dataset (24) grouped into 6–11-, 12–15-, and 16–20-aa peptides are compared with Swiss-Prot 12-, 14-, and 18-aa peptides of the Bcpreds BCP12, BCP14, and BCP18 datasets (20). The p value for hydrophilicity score differences between epitopes and non-epitopes is shown in green and between non-epitopes and Swiss-Prot random peptides in blue. All epitopes have higher hydrophilicity scores than Swiss-Prot random peptides (p value ≤ 10−5).
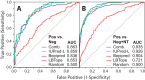
Comparison of ROC curves for prediction of 25-aa epitopes (Table 3). Plots of epitope-positive rate versus false-positive rate for the 18 chlamydial protein dataset are shown. A, prediction of epitopes from confirmed non-epitopes (25-aa epitopes/non-epitopes spaced 10 aa). The combined scale represents the arithmetic mean of two disorder scales, IUPred-L (31) and VSL2B (33), and one solvent accessibility scale, Accessible Surface Area, Spine-X (34). B, prediction of epitopes from the total remaining proteins (non-epitope plus non-tested regions). In both datasets (A and B), the combined scale and the single disorder (IUPred-L) scale performed best (highest sensitivity at given specificity or vice versa), significantly better than Bepipred or LBTope (one-tailed paired Student's t test, p value <10−4).
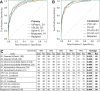
Combined scales provide only marginal improvement for B-cell epitope prediction. A, prediction by use of primary scales or B, combined scales. 2·D1 + S1, D1 score weighted 2×. Plots of true positive versus false-positive (ROC curve) are shown. C, prediction performance with 25-aa moving average scores of the Chl-18Prot dataset. At five specified sensitivities, B-cell epitope prediction specificities (Spec) and the corresponding accuracies (Acc) are shown.
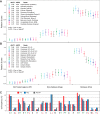
Comparative discriminatory power of protein property scales and machine learning algorithms, and dominant properties of B-cell epitope regions. Discrimination of proven epitopes, non-epitopes, and untested remaining total protein regions was evaluated in the Chl_18Prot dataset of 18 tested chlamydial protein. A, primary scales. Prediction scores (±95% CI) of protein property scales for epitope, non-epitope, and not tested datasets. B, combined scales and machine learning algorithms. Prediction scores (±95% CI) shown for combined scales derived from primary scales and for machine learning algorithms developed for B-cell epitope prediction. Combined scales are derived from primary scales (
supplemental Table S2). C, comparative amino acid frequencies of B-cell epitope and non-epitope regions.
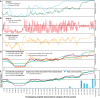
Optimal B-cell epitope prediction. A, disorder scores plotted against the C. pecorum IncA protein residues. Scores were obtained at default settings from IUPred-L (31) and VSL2B (33) web servers. B, default ASA_Spine-X solvent accessibility scores (34). C, hydrophilicity scores (inverted default Miyazawa hydrophobicity (29, 30)). D, standardized 25-aa moving average smoothed scores of scales shown in A–C. E, IUPred-L and combined scale scores compared with IncA peptide antigen reactivity with mouse sera. The combined scale is derived from the unweighted mean of standardized smoothed scores of scales shown in D.
Similar articles
-
Discovery of Human-Specific Immunodominant Chlamydia trachomatis B Cell Epitopes.
Rahman KS, Darville T, Russell AN, O'Connell CM, Wiesenfeld HC, Hillier SL, Chowdhury EU, Juan YC, Kaltenboeck B. Rahman KS, et al. mSphere. 2018 Aug 1;3(4):e00246-18. doi: 10.1128/mSphere.00246-18. mSphere. 2018. PMID: 30068558 Free PMC article.
-
Rahman KS, Chowdhury EU, Poudel A, Ruettger A, Sachse K, Kaltenboeck B. Rahman KS, et al. Clin Vaccine Immunol. 2015 May;22(5):539-52. doi: 10.1128/CVI.00102-15. Epub 2015 Mar 11. Clin Vaccine Immunol. 2015. PMID: 25761461 Free PMC article.
-
Caoili SEC. Caoili SEC. Protein Pept Lett. 2021;28(8):953-962. doi: 10.2174/0929866528666210218215624. Protein Pept Lett. 2021. PMID: 33602065
-
B cell epitope mapping using synthetic peptides.
Carter JM, Loomis-Price L. Carter JM, et al. Curr Protoc Immunol. 2004 May;Chapter 9:Unit 9.4. doi: 10.1002/0471142735.im0904s60. Curr Protoc Immunol. 2004. PMID: 18432936 Review.
-
Sharon J, Rynkiewicz MJ, Lu Z, Yang CY. Sharon J, et al. Immunology. 2014 May;142(1):1-23. doi: 10.1111/imm.12213. Immunology. 2014. PMID: 24219801 Free PMC article. Review.
Cited by
-
Comprehending B-Cell Epitope Prediction to Develop Vaccines and Immunodiagnostics.
Caoili SEC. Caoili SEC. Front Immunol. 2022 Jul 7;13:908459. doi: 10.3389/fimmu.2022.908459. eCollection 2022. Front Immunol. 2022. PMID: 35874755 Free PMC article. No abstract available.
-
Bommana S, Walker E, Desclozeaux M, Timms P, Polkinghorne A. Bommana S, et al. PLoS One. 2017 Nov 30;12(11):e0188370. doi: 10.1371/journal.pone.0188370. eCollection 2017. PLoS One. 2017. PMID: 29190736 Free PMC article.
-
Batisti Biffignandi G, Vola A, Sassera D, Najafi-Fard S, Gomez Morales MA, Brunetti E, Teggi A, Goletti D, Petrone L, Tamarozzi F. Batisti Biffignandi G, et al. PLoS Negl Trop Dis. 2023 Apr 12;17(4):e0011210. doi: 10.1371/journal.pntd.0011210. eCollection 2023 Apr. PLoS Negl Trop Dis. 2023. PMID: 37043489 Free PMC article.
-
iBCE-EL: A New Ensemble Learning Framework for Improved Linear B-Cell Epitope Prediction.
Manavalan B, Govindaraj RG, Shin TH, Kim MO, Lee G. Manavalan B, et al. Front Immunol. 2018 Jul 27;9:1695. doi: 10.3389/fimmu.2018.01695. eCollection 2018. Front Immunol. 2018. PMID: 30100904 Free PMC article.
-
Discovery of Human-Specific Immunodominant Chlamydia trachomatis B Cell Epitopes.
Rahman KS, Darville T, Russell AN, O'Connell CM, Wiesenfeld HC, Hillier SL, Chowdhury EU, Juan YC, Kaltenboeck B. Rahman KS, et al. mSphere. 2018 Aug 1;3(4):e00246-18. doi: 10.1128/mSphere.00246-18. mSphere. 2018. PMID: 30068558 Free PMC article.
References
-
- Rubinstein N. D., Mayrose I., Halperin D., Yekutieli D., Gershoni J. M., and Pupko T. (2008) Computational characterization of B-cell epitopes. Mol. Immunol. 45, 3477–3489 - PubMed
-
- Ofran Y., Schlessinger A., and Rost B. (2008) Automated identification of complementarity determining regions (CDRs) reveals peculiar characteristics of CDRs and B-cell epitopes. J. Immunol. 181, 6230–6235 - PubMed
-
- Sun J., Xu T., Wang S., Li G., Wu D., and Cao Z. (2011) Does difference exist between epitope and non-epitope residues? Immunome Res. 201, 1–11
Publication types
MeSH terms
Substances
LinkOut - more resources
Full Text Sources
Other Literature Sources
Medical
Miscellaneous