Automatic learning of pre-miRNAs from different species - PubMed
- ️Fri Jan 01 2016
Automatic learning of pre-miRNAs from different species
Ivani de O N Lopes et al. BMC Bioinformatics. 2016.
Abstract
Background: Discovery of microRNAs (miRNAs) relies on predictive models for characteristic features from miRNA precursors (pre-miRNAs). The short length of miRNA genes and the lack of pronounced sequence features complicate this task. To accommodate the peculiarities of plant and animal miRNAs systems, tools for both systems have evolved differently. However, these tools are biased towards the species for which they were primarily developed and, consequently, their predictive performance on data sets from other species of the same kingdom might be lower. While these biases are intrinsic to the species, their characterization can lead to computational approaches capable of diminishing their negative effect on the accuracy of pre-miRNAs predictive models. We investigate in this study how 45 predictive models induced for data sets from 45 species, distributed in eight subphyla/classes, perform when applied to a species different from the species used in its induction.
Results: Our computational experiments show that the separability of pre-miRNAs and pseudo pre-miRNAs instances is species-dependent and no feature set performs well for all species, even within the same subphylum/class. Mitigating this species dependency, we show that an ensemble of classifiers reduced the classification errors for all 45 species. As the ensemble members were obtained using meaningful, and yet computationally viable feature sets, the ensembles also have a lower computational cost than individual classifiers that rely on energy stability parameters, which are of prohibitive computational cost in large scale applications.
Conclusion: In this study, the combination of multiple pre-miRNAs feature sets and multiple learning biases enhanced the predictive accuracy of pre-miRNAs classifiers of 45 species. This is certainly a promising approach to be incorporated in miRNA discovery tools towards more accurate and less species-dependent tools. The material to reproduce the results from this paper can be downloaded from http://dx.doi.org/10.5281/zenodo.49754 .
Figures
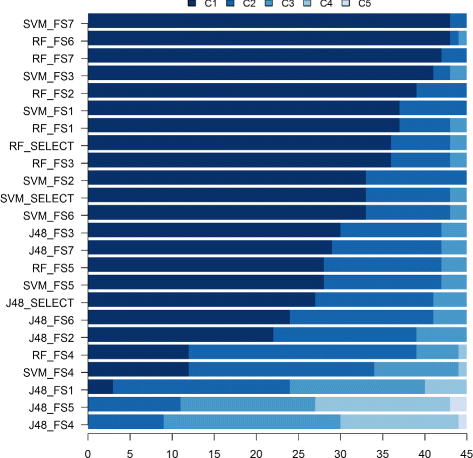
Frequencies of species for who each classification model achieved accuracies in the clusters C1-C5. MeanC1≥..≥MeanC5
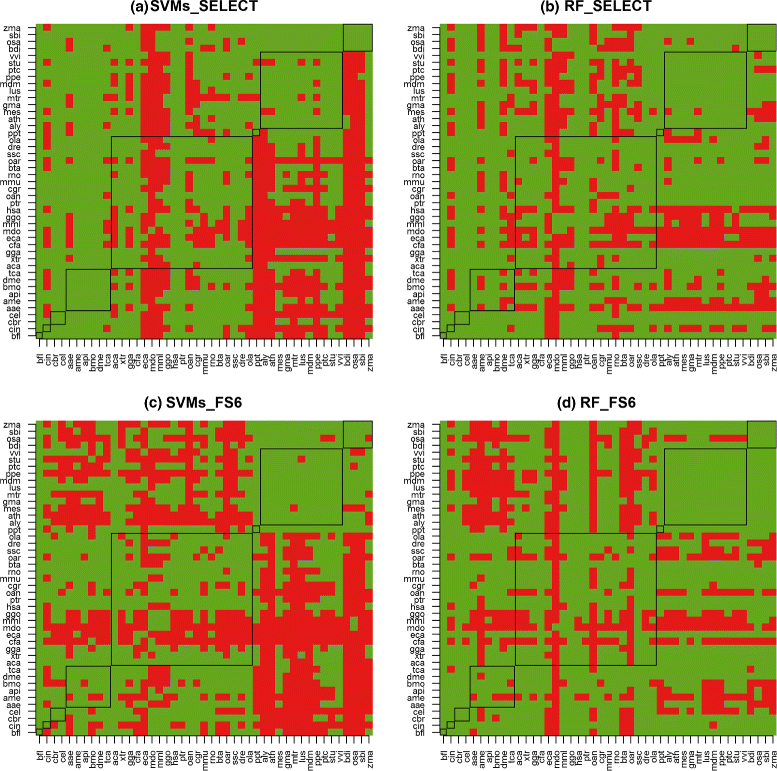
Accuracy cluster membership (columns) for cross-species pre-miRNAs classifiers. Green = c1; red =other; y-axis =model species; x-axis =test species; black frames encloses species from the same subphylum/class. Figures (a), (b), (c) and (d) show interactions between the learning algorithms SVMs and RF and the feature sets SELECT and FS6
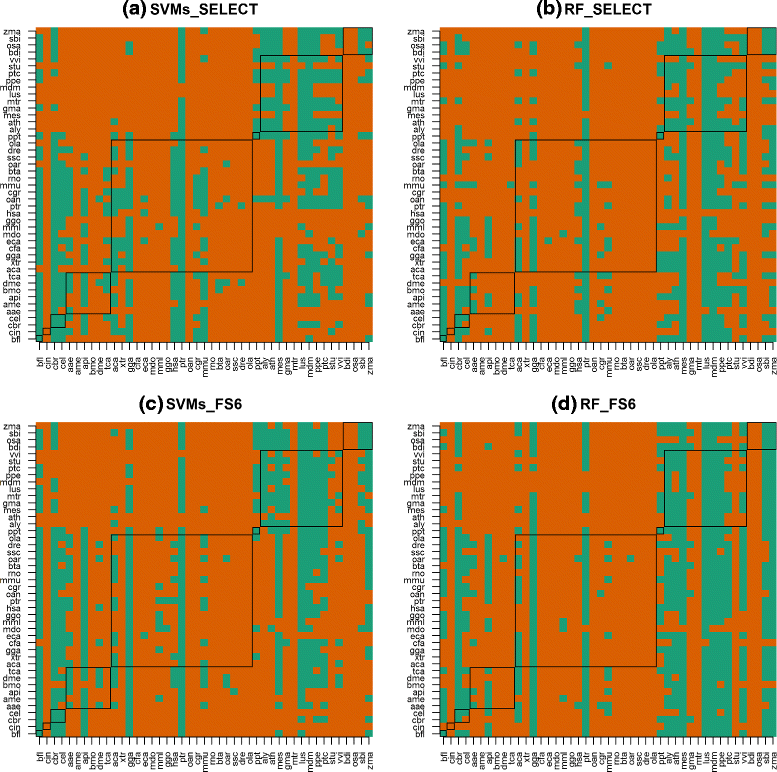
Accuracy cluster membership (rows) for cross-species pre-miRNAs classifiers. Green = c1; red =other; y-axis =model species; x-axis =test species; black frames encloses species from the same subphylum/class. Figures (a), (b), (c) and (d) show interactions between the learning algorithms SVMs and RF and the feature sets SELECT and FS6
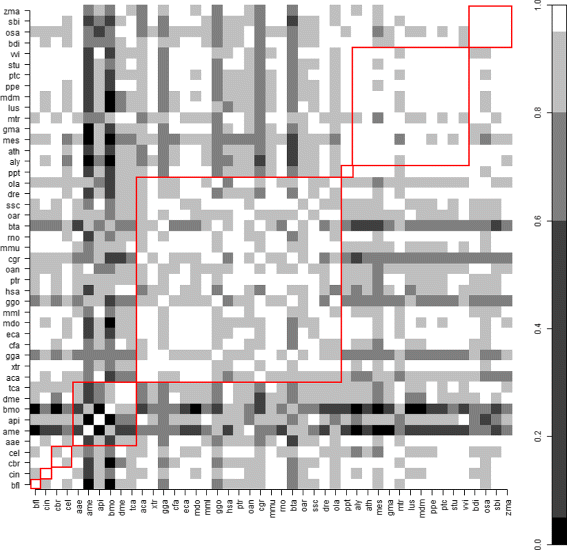
Pairwise Pearson correlation coefficient of RFI throughout species. Correlations breaks: 0, 0.05, 0.6, 0.8, 0.95 and 1
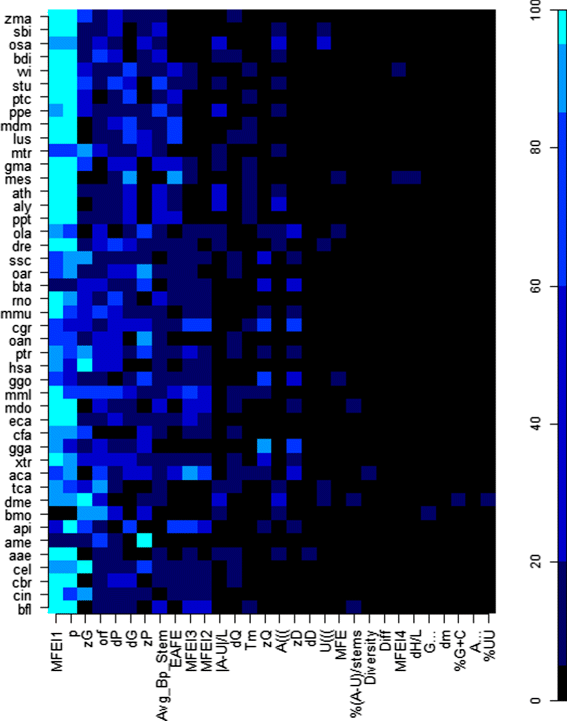
Thirty most relevant features for pre-miRNAs classification, according to RFI values, per species. RFI breaks: 0, 5, 20, 60, 85, 95 and 100
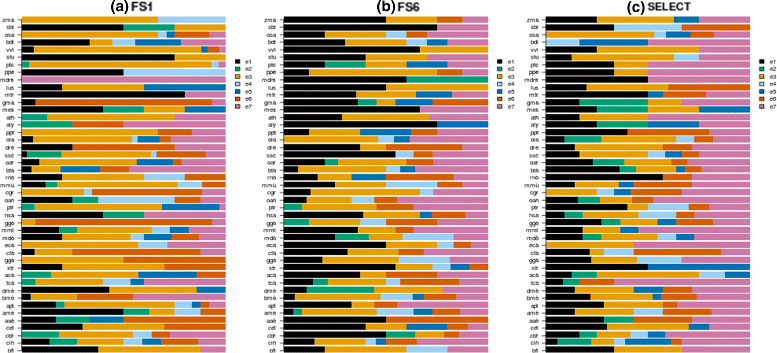
Distribution of classification errors per species. Exclusive errors by SVMs (e 1), RF (e 2), J48 (e 3), SVMs and RF (e 4), SVMs and J48 (e 5), RF and J48 (e 6) and SVMs and RF and J48 (e 7). Figures (a), (b) and (c) illustrate that, fixing the feature set, the number of instances simultaneously misclassified by two or three learning algorithms depends on species
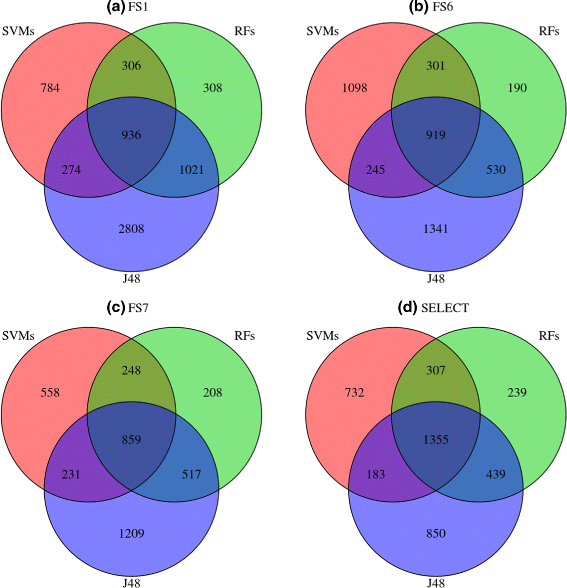
Venn diagram of the classification errors of the classification algorithms, by feature set. Results were obtained from the classification of 27,000 = 45 (test species) ×10 (repetitions) ×60 (30+,30-). Figures (a), (b), (c) and (d) illustrate that the number of instances simultaneously misclassified by two or three learning algorithms depends on the feature set
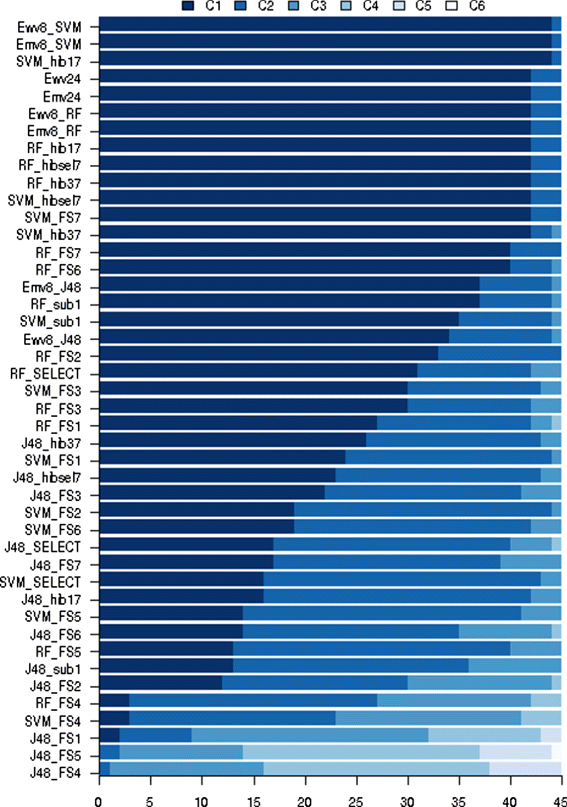
Distribution of the accuracies of 44 classifiers within the accuracy clusters. MeanC1>…>MeanC6
Similar articles
-
The discriminant power of RNA features for pre-miRNA recognition.
Lopes Ide O, Schliep A, de Carvalho AC. Lopes Ide O, et al. BMC Bioinformatics. 2014 May 2;15:124. doi: 10.1186/1471-2105-15-124. BMC Bioinformatics. 2014. PMID: 24884650 Free PMC article.
-
Yao Y, Ma C, Deng H, Liu Q, Zhang J, Yi M. Yao Y, et al. Mol Biosyst. 2016 Oct 20;12(10):3124-31. doi: 10.1039/c6mb00295a. Epub 2016 Jul 29. Mol Biosyst. 2016. PMID: 27472470
-
Wang M, Song X, Han P, Li W, Jiang B. Wang M, et al. J Theor Biol. 2010 May 21;264(2):578-84. doi: 10.1016/j.jtbi.2010.02.037. Epub 2010 Mar 2. J Theor Biol. 2010. PMID: 20202471
-
Predicting novel microRNA: a comprehensive comparison of machine learning approaches.
Stegmayer G, Di Persia LE, Rubiolo M, Gerard M, Pividori M, Yones C, Bugnon LA, Rodriguez T, Raad J, Milone DH. Stegmayer G, et al. Brief Bioinform. 2019 Sep 27;20(5):1607-1620. doi: 10.1093/bib/bby037. Brief Bioinform. 2019. PMID: 29800232 Review.
-
Genome-wide discovery of pre-miRNAs: comparison of recent approaches based on machine learning.
Bugnon LA, Yones C, Milone DH, Stegmayer G. Bugnon LA, et al. Brief Bioinform. 2021 May 20;22(3):bbaa184. doi: 10.1093/bib/bbaa184. Brief Bioinform. 2021. PMID: 34020552 Review.
Cited by
-
Improved Pre-miRNAs Identification Through Mutual Information of Pre-miRNA Sequences and Structures.
Fu X, Zhu W, Cai L, Liao B, Peng L, Chen Y, Yang J. Fu X, et al. Front Genet. 2019 Feb 25;10:119. doi: 10.3389/fgene.2019.00119. eCollection 2019. Front Genet. 2019. PMID: 30858864 Free PMC article.
-
Tavara S, Schliep A. Tavara S, et al. PeerJ Comput Sci. 2021 Mar 9;7:e397. doi: 10.7717/peerj-cs.397. eCollection 2021. PeerJ Comput Sci. 2021. PMID: 33817043 Free PMC article.
-
MicroRNA categorization using sequence motifs and k-mers.
Yousef M, Khalifa W, Acar İE, Allmer J. Yousef M, et al. BMC Bioinformatics. 2017 Mar 14;18(1):170. doi: 10.1186/s12859-017-1584-1. BMC Bioinformatics. 2017. PMID: 28292266 Free PMC article.
-
Garg P, Jamal F, Srivastava P. Garg P, et al. IBRO Neurosci Rep. 2022 Oct 21;13:393-401. doi: 10.1016/j.ibneur.2022.10.005. eCollection 2022 Dec. IBRO Neurosci Rep. 2022. PMID: 36345471 Free PMC article.
References
MeSH terms
Substances
LinkOut - more resources
Full Text Sources
Other Literature Sources
Research Materials