Search strategies of Wikipedia readers - PubMed
- ️Sun Jan 01 2017
Search strategies of Wikipedia readers
Giovanna Chiara Rodi et al. PLoS One. 2017.
Abstract
The quest for information is one of the most common activity of human beings. Despite the the impressive progress of search engines, not to miss the needed piece of information could be still very tough, as well as to acquire specific competences and knowledge by shaping and following the proper learning paths. Indeed, the need to find sensible paths in information networks is one of the biggest challenges of our societies and, to effectively address it, it is important to investigate the strategies adopted by human users to cope with the cognitive bottleneck of finding their way in a growing sea of information. Here we focus on the case of Wikipedia and investigate a recently released dataset about users' click on the English Wikipedia, namely the English Wikipedia Clickstream. We perform a semantically charged analysis to uncover the general patterns followed by information seekers in the multi-dimensional space of Wikipedia topics/categories. We discover the existence of well defined strategies in which users tend to start from very general, i.e., semantically broad, pages and progressively narrow down the scope of their navigation, while keeping a growing semantic coherence. This is unlike strategies associated to tasks with predefined search goals, namely the case of the Wikispeedia game. In this case users first move from the 'particular' to the 'universal' before focusing down again to the required target. The clear picture offered here represents a very important stepping stone towards a better design of information networks and recommendation strategies, as well as the construction of radically new learning paths.
Conflict of interest statement
The authors have declared that no competing interests exist.
Figures
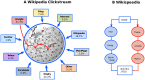
In (A) we illustrate the English Wikipedia Clickstream dataset. The 9 different external sources plus the MainPage are illustrated with the fraction of flux outgoing from them. The paths we considered in our analysis start from one of the 9 sources to randomly walking over the Wikipedia articles accordingly to the transition counts provided by the dataset. (B) Two examples of paths followed by players of the Wikispeedia game, whose task was that of navigating on a reduced version of Wikipedia from a given starting page to a given target one (from House to Electric_Field in the example).
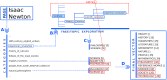
For the Isaac Newton page one first considers the list of parents categories (panel A). For each category, one identifies the most-representative-topics (panel B), selecting the ones from which the depth of the category in the categories tree is minimal. For each page, we consider the whole list of most-representative-topics and corresponding depths (panel C). For instance the category copernican_revolution has the smallest depth (equal to 3) in the tree of the topic SCIENCE. The vector representation of the coordinates of the main topics is now obtained by weighting each topic with the inverse of the minimal depth computed above (panel D). For instance the topic SCIENCE appears in the topical vector with weight 1/2.
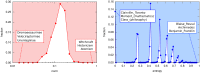
The distributions are computed over the set of all pages for which a vector representation was derived. For both norm and entropy, in the boxes some exemplar pages are reported to illustrate the meaning of extreme values.
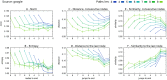
The 107 paths simulated with google as source were split by lengths. For each fixed length l, we computed the averages of the following quantities over all the nodes(pairs) at k steps(jumps) to the end: (A) the average norm ∥wkl∥¯, (B) the entropy S(wkl)¯, (C) the distance and (E) the similarity between all the pairs of nodes consecutively visited along each path, respectively d(wkl,wk-1l)¯ and sim(wkl,wk-1l)¯, (D) the distance and (F) the similarity between every node visited and the ending node along each path, i.e. d(wkl,w0l)¯ and sim(wkl,w0l)¯. The error bars display the standard errors of the means. Each color refers to a path length, from 3 (blue) to 9 (light green).
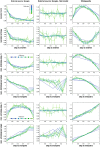
In this panel we report the same data of Fig 4 (left column) after rescaling. The walks lengths are normalized to 1. The corresponding averages for step of the different measures (A)-(F) are rescaled with the mean value of the same measures evaluated over the whole set of nodes belonging to paths with the same length. The averages used to rescale the data are displayed in Fig D in S1 File. In the central and right columns similarly processed data are reported which refer respectively to a semantically uncorrelated model based on the google paths and to the Wikispeedia paths. Each color refers to a path length, from 3 (blue) to 9 (light green). The standard error of the means are reported.
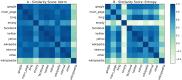
For the two observables norm (left panel) and entropy (right panel), we report the matrix of similarities score between all the sources and Wikispeedia. The score is defined by Eq (6). For each pair of sources, the unrescaled averages values of the observable are considered (as in Fig 4). Then, for each path length between 4 and 9, the Spearman correlation coefficient is computed between the averaged values of the observable. The final score is the obtained after averaging over all the lengths.
Similar articles
-
Seeking health information online: does Wikipedia matter?
Laurent MR, Vickers TJ. Laurent MR, et al. J Am Med Inform Assoc. 2009 Jul-Aug;16(4):471-9. doi: 10.1197/jamia.M3059. Epub 2009 Apr 23. J Am Med Inform Assoc. 2009. PMID: 19390105 Free PMC article.
-
Sanchiz M, Amadieu F, Fu WT, Chevalier A. Sanchiz M, et al. Appl Ergon. 2019 Feb;75:201-213. doi: 10.1016/j.apergo.2018.10.010. Epub 2018 Nov 8. Appl Ergon. 2019. PMID: 30509528
-
How the structure of Wikipedia articles influences user navigation.
Lamprecht D, Lerman K, Helic D, Strohmaier M. Lamprecht D, et al. New Rev Hypermedia Multimed. 2017 Jan 2;23(1):29-50. doi: 10.1080/13614568.2016.1179798. Epub 2016 May 12. New Rev Hypermedia Multimed. 2017. PMID: 28670171 Free PMC article.
-
Joshi MP, Bhangoo RS, Kumar K. Joshi MP, et al. Technol Health Care. 2011;19(6):391-400. doi: 10.3233/THC-2011-0643. Technol Health Care. 2011. PMID: 22129940 Review.
-
Analyzing Medical Image Search Behavior: Semantics and Prediction of Query Results.
De-Arteaga M, Eggel I, Kahn CE Jr, Müller H. De-Arteaga M, et al. J Digit Imaging. 2015 Oct;28(5):537-46. doi: 10.1007/s10278-015-9792-6. J Digit Imaging. 2015. PMID: 25810317 Free PMC article. Review.
Cited by
-
Architectural styles of curiosity in global Wikipedia mobile app readership.
Zhou D, Patankar S, Lydon-Staley DM, Zurn P, Gerlach M, Bassett DS. Zhou D, et al. Sci Adv. 2024 Oct 25;10(43):eadn3268. doi: 10.1126/sciadv.adn3268. Epub 2024 Oct 25. Sci Adv. 2024. PMID: 39454011 Free PMC article.
-
Knowledge categorization affects popularity and quality of Wikipedia articles.
Lerner J, Lomi A. Lerner J, et al. PLoS One. 2018 Jan 2;13(1):e0190674. doi: 10.1371/journal.pone.0190674. eCollection 2018. PLoS One. 2018. PMID: 29293627 Free PMC article.
References
-
- Levitin DJ. The Organized Mind: Thinking Straight in the Age of Information Overload. Dutton; 2014.
-
- Foerde K, Knowlton BJ, Poldrack RA. Modulation of competing memory systems by distraction. Proceedings of the National Academy of Sciences. 2006;103(31):11778–11783. Available from: http://www.pnas.org/content/103/31/11778.abstract 10.1073/pnas.0602659103 - DOI - PMC - PubMed
-
- Schweizer TA, Kan K, Hung Y, Tam F, Naglie G, Graham S. Brain activity during driving with distraction: an immersive fMRI study. Frontiers in Human Neuroscience. 2013;7(53). Available from: http://www.frontiersin.org/human_neuroscience/10.3389/fnhum.2013.00053/a... 10.3389/fnhum.2013.00053 - DOI - DOI - PMC - PubMed
MeSH terms
Grants and funding
The authors acknowledge support from the KREYON project funded by the John Templeton Foundation under contract n. 51663. The sponsors had no role in study design, data collection and analysis, decision to publish, or preparation of the manuscript.
LinkOut - more resources
Full Text Sources
Other Literature Sources