Mapping the ecological networks of microbial communities - PubMed
- ️Sun Jan 01 2017
Mapping the ecological networks of microbial communities
Yandong Xiao et al. Nat Commun. 2017.
Abstract
Mapping the ecological networks of microbial communities is a necessary step toward understanding their assembly rules and predicting their temporal behavior. However, existing methods require assuming a particular population dynamics model, which is not known a priori. Moreover, those methods require fitting longitudinal abundance data, which are often not informative enough for reliable inference. To overcome these limitations, here we develop a new method based on steady-state abundance data. Our method can infer the network topology and inter-taxa interaction types without assuming any particular population dynamics model. Additionally, when the population dynamics is assumed to follow the classic Generalized Lotka-Volterra model, our method can infer the inter-taxa interaction strengths and intrinsic growth rates. We systematically validate our method using simulated data, and then apply it to four experimental data sets. Our method represents a key step towards reliable modeling of complex, real-world microbial communities, such as the human gut microbiota.
Conflict of interest statement
The authors declare that they have no competing financial interests.
Figures
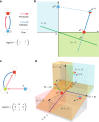
Inferring ecological interaction types for a small microbial community. The interaction types are coded as the sign-pattern of the Jacobian matrix. a For a microbial community of two taxa, its ecological network and the sign-pattern of the corresponding Jacobian matrix are shown here. b There are three possible steady-state samples (shown as colored pie charts), and two of them x{1,2},x1 share taxon 1. We calculate the green line that passes through the origin and is perpendicular to the vector (x1,2-x1) (shown as a blue line segment). This green line crosses the origin, and two other orthants (shown in light cyan and green), offering a set of possible sign-patterns: 0,0,(-,-) and (+,-), for which s1=(signJ11,sign(J12)) may belong to. Provided that J11<0, we conclude that s1=(-,+). c For a microbial community of three taxa, its ecological network and the sign-pattern of the corresponding Jacobian matrix are shown here. d There are seven possible steady-state samples, and we plot four of them that share taxon 1. Consider a line segment (x{1,3}-x{1}) (solid blue). We calculate the orange plane that passes through the origin and is perpendicular to this solid blue line. This orange plane crosses nine regions: the origin and the other eight regions (denoted in different color cubes, color lines), offering nine possible sign-patterns for s 1. We can consider another line segment that connects two steady-state samples sharing taxon 1, say, x{1,3} and x{1,2,3}, and repeat the above procedure. We do this for all the sample pairs (dashed blue lines), record the regions crossed by the corresponding orthogonal planes. Finally, the intersection of the regions crossed by all those orthogonal hyperplanes yields a minimum set of sign-patterns S^1={-,0,+,0,0,0,+,0,-} that s 1 may belong to. If we know that J11<0, then we can uniquely determine s1=(-,0,+)
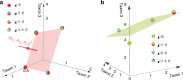
Consistency check of the GLV model and the observed steady-state samples. For a microbial community following exactly the GLV dynamics, all its steady-state samples sharing one common taxon will align onto a hyperplane in the state space. a Here we consider a microbial community of three taxa. There are four steady-state samples {x{1},x{1,2},x{1,3},x{1,2,3}} that share common taxon 1. Those four steady-state samples represent four points in the state space, and they align onto a plane (light red). The normal vector of this plane is parallel to the first row a 1 of the interaction matrix A in the GLV model. Given any one of non-zero entries in a 1, we can determine the exact values of all other entries. Otherwise, we can always express the inter-taxa interaction strengths a ij (j≠i) as a function of the intra-taxa interaction strength a ii. b Here we again consider a microbial community of three taxa. Taxon-1 and taxon-2 follow the GLV dynamics, but taxon-3 does not. Then those steady-state samples that share taxon-3 do not align onto a plane anymore. Here we show the best fitted plane (in green) by minimizing the distance between this plane and the four steady states, with the coefficient of determination R2=0.77
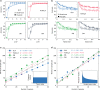
Validation of our method in inferring interaction types using simulated data. a,b Consider a small microbial community of N=8 taxa. We generate steady-state samples using four different population dynamics models: Generalized Lotka–Volterra (GLV), Holling Type II (Holling II), DeAngelis–Beddington (DB), and Crowley–Martin (CM). We compare the performance of the brute-force algorithm (with solution space ~38=6,561) and the heuristic algorithm (with solution space ~Ψ=5N=40). a In the noiseless case, we plot the inference accuracy (defined as the percentage of correctly inferred signs in the Jacobian matrix) as a function of sample size Ω. b In the presence of noise, we plot the inference accuracy as a function of the noise level η. Here the sample size is fixed: Ω=5N=40. The error bar represents standard deviation for 10 different realizations. c, d We calculate the minimal sample size Ω* required for the heuristic algorithm to achieve high accuracy (100% for GLV, 95% for Holling II, DB, and CM) at different system sizes. We consider two different taxa presence patterns: uniform and heterogenous (see insets). Here the simulated data is generated in the noiseless case and we chose Ψ=10N. a–d The underlying ecological network is generated from a directed random graph model with connectivity 0.4 (i.e., with probability 0.4 there will be a directed edge between any two taxa)
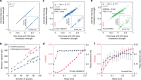
Validation of our method in inferring interaction strengths using simulated data. Here, we simulate steady-state samples using the GLV model with N taxa and intra-taxa interaction strength aii=-1 for each taxon. The underlying ecological network is generated from a directed random graph model with connectivity 0.4. The noise is added to steady-state samples as follows: xiI→xiI+ηu, where the random number u follows a uniform distribution U[-xiI,xiI], and η is the noise level. a, b Consider the ideal case: (1) noiseless η=0; and (2) we know exactly aii=-1. a We can perfectly infer a ij’s and r i’s. b The minimal sample size Ω* required to correctly infer the interaction strengths scales linearly with the system size N. Here we consider two different taxa presence pattern: uniform and heterogenous. c–f In the presence of noise, and during the we just assume that the intra-taxa interaction strengths âii follows a half-normal distribution. c Using the Lasso regularization induces high false-discovery rate (FDR) ~ 0.448 at η=0.1. d Using classical Lasso with cross validation, both NRMSE and FDR increase with increasing η. e For the same data set used in c, we use the knockoff filter to control the FDR below a certain level q=0.2. f With increasing noise level η, FDR can still be successfully controlled below q=0.2 by applying the knockoff filter. In subfigures a, c–f, we have N=50. The error bar represents standard deviation for 10 different realizations
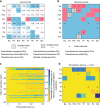
Inferring interaction types of a synthetic soil microbial community. The steady-state samples were experimentally collected from a synthetic soil microbial community of eight bacterial species. Those steady-state samples involve 101 different species combinations: all eight solos, 28 duos, 56 trios, all eight septets, and 1 octet. a From the eight solos (monoculture experiments) and 28 duos (pair-wise co-culture experiments), one can calculate the relative yield R ij, quantifying the promotion (positive) or inhibition (negative) impact of species j on species i. The values shown in the relative yield matrix R=(Rij) quantify the strengths of promotion and inhibition effects. The sign-pattern of this matrix serves as the ground truth of that of the Jacobian matrix associated with the unknown population dynamics of this microbial community. b Without considering the 8 solos and 28 duos, we analyze the other steady-state samples. We use the brute-force method to infer the ecological interaction types, i.e., the sign-pattern of the Jacobian matrix. Blue (or red) means inhibition (or promotion) effect of species j on species i, respectively. 10 signs (labeled by ‘×’) are falsely inferred, four signs (gray) are undetermined by the analyzed steady-state samples. c, d The robustness of the inference results in the presence of artificially added noise: xiI→xiI+ηu, where the random number u follows a uniform distribution U[-xiI,xiI], and η is the noise level. At each noise level, we run 50 different realizations. Yellow (or blue) means many (or few) inferred J ij keep the same sign among 50 different realizations. c. Many of the inferred J ij keep their signs in the presence of noise up to noise level η=0.3. d At η=0.04, we plot the percentage of unchanged signs for inferred Jacobian matrix in 50 different realizations. The ‘×’ labels correspond to the 10 falsely inferred signs shown in b. Five of the 10 falsely inferred interactions change their signs frequently even when the perturbation is very small, implying that the falsely inferred signs in b could be due to measurement noise in the experiments
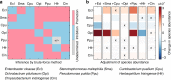
Inferring interaction types in a synthetic community of maize roots with seven bacterial species. The data set consists of seven sextets and one septet. a Without considering the one septet, we analyze the seven sextets (steady-state samples involving six of the seven species). We use the brute-force method to infer the ecological interaction types, i.e., the sign-pattern of the Jacobian matrix. Blue (or red) means inhibition (or promotion) effect of species j on species i, respectively. One sign (gray) is undetermined from the seven sextets. b The changes of species abundance before and after, respectively, adding one species into a sextet. Blue (or red) corresponds to the decrease (or increase) of one species’ abundance after introducing a new species into sextets, respectively. Each column corresponds to a sextet (a six-baterial community), the name of the newly introduced species is marked in the top of each column. ‘×’ indicates the false prediction. There are in total 12 false predictions
Similar articles
-
Identifying Keystone Species in the Microbial Community Based on Cross- Sectional Data.
Li M, Zhang J, Wu B, Zhou Z, Xu Y. Li M, et al. Curr Gene Ther. 2018;18(5):296-306. doi: 10.2174/1566523218666181008155734. Curr Gene Ther. 2018. PMID: 30306866
-
Universality of human microbial dynamics.
Bashan A, Gibson TE, Friedman J, Carey VJ, Weiss ST, Hohmann EL, Liu YY. Bashan A, et al. Nature. 2016 Jun 9;534(7606):259-62. doi: 10.1038/nature18301. Nature. 2016. PMID: 27279224 Free PMC article.
-
Suzuki K, Abe MS, Kumakura D, Nakaoka S, Fujiwara F, Miyamoto H, Nakaguma T, Okada M, Sakurai K, Shimizu S, Iwata H, Masuya H, Nihei N, Ichihashi Y. Suzuki K, et al. Int J Environ Res Public Health. 2022 Jan 22;19(3):1228. doi: 10.3390/ijerph19031228. Int J Environ Res Public Health. 2022. PMID: 35162258 Free PMC article.
-
Establishing Causality: Opportunities of Synthetic Communities for Plant Microbiome Research.
Vorholt JA, Vogel C, Carlström CI, Müller DB. Vorholt JA, et al. Cell Host Microbe. 2017 Aug 9;22(2):142-155. doi: 10.1016/j.chom.2017.07.004. Cell Host Microbe. 2017. PMID: 28799900 Review.
-
Dohlman AB, Shen X. Dohlman AB, et al. Exp Biol Med (Maywood). 2019 Apr;244(6):445-458. doi: 10.1177/1535370219836771. Epub 2019 Mar 16. Exp Biol Med (Maywood). 2019. PMID: 30880449 Free PMC article. Review.
Cited by
-
Martínez-Álvaro M, Auffret MD, Stewart RD, Dewhurst RJ, Duthie CA, Rooke JA, Wallace RJ, Shih B, Freeman TC, Watson M, Roehe R. Martínez-Álvaro M, et al. Front Microbiol. 2020 Apr 17;11:659. doi: 10.3389/fmicb.2020.00659. eCollection 2020. Front Microbiol. 2020. PMID: 32362882 Free PMC article.
-
Ma L, Huang X, Wang H, Yun Y, Cheng X, Liu D, Lu X, Qiu X. Ma L, et al. Microbiol Spectr. 2021 Oct 31;9(2):e0115221. doi: 10.1128/Spectrum.01152-21. Epub 2021 Sep 8. Microbiol Spectr. 2021. PMID: 34494852 Free PMC article.
-
Ren K, Mo Y, Xiao P, Rønn R, Xu Z, Xue Y, Chen H, Rivera WL, Rensing C, Yang J. Ren K, et al. ISME Commun. 2024 Feb 24;4(1):ycae026. doi: 10.1093/ismeco/ycae026. eCollection 2024 Jan. ISME Commun. 2024. PMID: 38559570 Free PMC article.
-
Phosphorus source driving the soil microbial interactions and improving sugarcane development.
Gumiere T, Rousseau AN, da Costa DP, Cassetari A, Cotta SR, Andreote FD, Gumiere SJ, Pavinato PS. Gumiere T, et al. Sci Rep. 2019 Mar 13;9(1):4400. doi: 10.1038/s41598-019-40910-1. Sci Rep. 2019. PMID: 30867512 Free PMC article.
-
Guex I, Mazza C, Dubey M, Batsch M, Li R, van der Meer JR. Guex I, et al. PLoS Comput Biol. 2023 Aug 21;19(8):e1011402. doi: 10.1371/journal.pcbi.1011402. eCollection 2023 Aug. PLoS Comput Biol. 2023. PMID: 37603551 Free PMC article.
References
-
- Nannipieri P, et al. Microbial diversity and soil functions. Eur. J. Soil Sci. 2003;54:655–670. doi: 10.1046/j.1351-0754.2003.0556.x. - DOI
Publication types
MeSH terms
LinkOut - more resources
Full Text Sources
Other Literature Sources