TerraClimate, a high-resolution global dataset of monthly climate and climatic water balance from 1958-2015 - PubMed
- ️Mon Jan 01 2018
TerraClimate, a high-resolution global dataset of monthly climate and climatic water balance from 1958-2015
John T Abatzoglou et al. Sci Data. 2018.
Abstract
We present TerraClimate, a dataset of high-spatial resolution (1/24°, ~4-km) monthly climate and climatic water balance for global terrestrial surfaces from 1958-2015. TerraClimate uses climatically aided interpolation, combining high-spatial resolution climatological normals from the WorldClim dataset, with coarser resolution time varying (i.e., monthly) data from other sources to produce a monthly dataset of precipitation, maximum and minimum temperature, wind speed, vapor pressure, and solar radiation. TerraClimate additionally produces monthly surface water balance datasets using a water balance model that incorporates reference evapotranspiration, precipitation, temperature, and interpolated plant extractable soil water capacity. These data provide important inputs for ecological and hydrological studies at global scales that require high spatial resolution and time varying climate and climatic water balance data. We validated spatiotemporal aspects of TerraClimate using annual temperature, precipitation, and calculated reference evapotranspiration from station data, as well as annual runoff from streamflow gauges. TerraClimate datasets showed noted improvement in overall mean absolute error and increased spatial realism relative to coarser resolution gridded datasets.
Conflict of interest statement
The authors declare no competing financial interests.
Figures
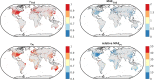
(a) Pearsons’ correlation coefficient (ρ) and (b) mean absolute error (MAE, units of °C) between GHCN stations and co-located pixels from TerraClimate for time series of annual mean temperature (TAS) from 1958–2015. (c,d) Show the correlation and relative mean absolute error (units of % of mean annual Pr) for calendar year accumulated precipitation (Pr). Statistics are reported for GHCN stations with at least 30 years of complete data.
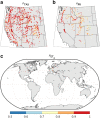
Pearsons’ correlation coefficient (ρ) with data from co-located pixels from TerraClimate and (a) annual mean temperature (TAS) from 1980–2015 from homogenized RAWS and SNOTEL stations, (b) annual mean precipitation (PR) from quality controlled SNOTEL stations, and (c) annual mean reference evapotranspiration (ET0) from FLUXNET stations from 1994–2014. Statistics are only reported for stations that had at least 10 years of complete data.
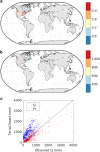
Validation statistics of TerraClimate estimated runoff from the WBM showing (a) Pearson’s correlation coefficient, and (b) mean absolute error (mm) for annual water-year (Oct-Sep) runoff (Q) for 587 streamflow from BGDC’s Climate Sensitive Stations Dataset covering at least 30 complete years from 1958–2015. (c) Shows a scatterplot of observed annual mean Q from streamflow stations versus TerraClimate estimated Q and water-year accumulated precipitation (P). The 1:1 line is shown for reference.
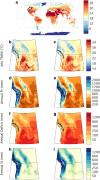
Top panel shows July 2015 monthly average maximum temperature (Tmax) from TerraClimate with the inset highlighting the region for (a–h). Comparison of (a,b) July 2015 Tmax, (c,d) 2015 calendar year accumulated precipitation, (e,f) 2015 calendar year climatic water deficit, and (g,h) 2015 runoff between the TerraClimate dataset (left) and CRU Ts4.0 dataset (right).
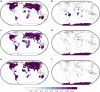
Proportion of monthly data with (left) at least 8 and (right) 0 stations from CRU Ts4.0 contributing to anomalies from 1958–2015. (a,b) Show coverage for mean temperature, used in TerraClimate maximum and minimum temperature, (c,d) show coverage for precipitation, and (e,f) show coverage for vapor pressure. TerraClimate uses anomalies from JRA-55 rather than assume climatological averages from CRU for voxels where 0 stations contribute.
Similar articles
-
Gaertner B. Gaertner B. Sci Total Environ. 2024 Feb 20;912:169211. doi: 10.1016/j.scitotenv.2023.169211. Epub 2023 Dec 12. Sci Total Environ. 2024. PMID: 38097071
-
Barbarossa V, Huijbregts MAJ, Beusen AHW, Beck HE, King H, Schipper AM. Barbarossa V, et al. Sci Data. 2018 Mar 27;5:180052. doi: 10.1038/sdata.2018.52. Sci Data. 2018. PMID: 29583139 Free PMC article.
-
High-resolution grids of daily air temperature for Peru - the new PISCOt v1.2 dataset.
Huerta A, Aybar C, Imfeld N, Correa K, Felipe-Obando O, Rau P, Drenkhan F, Lavado-Casimiro W. Huerta A, et al. Sci Data. 2023 Dec 1;10(1):847. doi: 10.1038/s41597-023-02777-w. Sci Data. 2023. PMID: 38040747 Free PMC article.
-
Diao Y, Pei T. Diao Y, et al. Ying Yong Sheng Tai Xue Bao. 2004 Dec;15(12):2369-76. Ying Yong Sheng Tai Xue Bao. 2004. PMID: 15825459 Review. Chinese.
-
Designing ecological climate change impact assessments to reflect key climatic drivers.
Sofaer HR, Barsugli JJ, Jarnevich CS, Abatzoglou JT, Talbert MK, Miller BW, Morisette JT. Sofaer HR, et al. Glob Chang Biol. 2017 Jul;23(7):2537-2553. doi: 10.1111/gcb.13653. Epub 2017 Mar 6. Glob Chang Biol. 2017. PMID: 28173628 Review.
Cited by
-
Widespread decline in winds delayed autumn foliar senescence over high latitudes.
Wu C, Wang J, Ciais P, Peñuelas J, Zhang X, Sonnentag O, Tian F, Wang X, Wang H, Liu R, Fu YH, Ge Q. Wu C, et al. Proc Natl Acad Sci U S A. 2021 Apr 20;118(16):e2015821118. doi: 10.1073/pnas.2015821118. Proc Natl Acad Sci U S A. 2021. PMID: 33846246 Free PMC article.
-
Observed humidity trends in dry regions contradict climate models.
Simpson IR, McKinnon KA, Kennedy D, Lawrence DM, Lehner F, Seager R. Simpson IR, et al. Proc Natl Acad Sci U S A. 2024 Jan 2;121(1):e2302480120. doi: 10.1073/pnas.2302480120. Epub 2023 Dec 26. Proc Natl Acad Sci U S A. 2024. PMID: 38147646 Free PMC article.
-
Zhang G, Roslan SNAB, Shafri HZM, Zhao Y, Wang C, Quan L. Zhang G, et al. Sci Rep. 2024 Jul 13;14(1):16212. doi: 10.1038/s41598-024-67109-3. Sci Rep. 2024. PMID: 39003342 Free PMC article.
-
Anthropogenic climate change has driven over 5 million km2 of drylands towards desertification.
Burrell AL, Evans JP, De Kauwe MG. Burrell AL, et al. Nat Commun. 2020 Jul 31;11(1):3853. doi: 10.1038/s41467-020-17710-7. Nat Commun. 2020. PMID: 32737311 Free PMC article.
-
Geng J, Yuan M, Xu S, Bai T, Xiao Y, Li X, Xu D. Geng J, et al. Int J Environ Res Public Health. 2022 Nov 25;19(23):15665. doi: 10.3390/ijerph192315665. Int J Environ Res Public Health. 2022. PMID: 36497740 Free PMC article.
References
Data Citations
-
- Harris I., Jones P. D., Osborn T. J., Lister D. H. 2017. Climatic Research Unit. http://dx.doi.org/10.5285/edf8febfdaad48abb2cbaf7d7e846a86 - DOI
-
- Abatzoglou J. T., Dobrowski S. Z., Parks S. A., Hegewisch K. C. 2017. Northwest Knowledge Network. http://doi.org/10.7923/G43J3B0R - DOI
References
-
- Harris I., Jones P. D., Osborn T. J. & Lister D. H. Updated high-resolution grids of monthly climatic observations—the CRU TS3.10 Dataset. Int. J. Climatol. 34, 623–642 (2014).
-
- Kobayashi S. et al. The JRA-55 reanalysis: General specifications and basic characteristics. J. Meteorol. Soc. Jpn. Ser. II 93, 5–48 (2015).
-
- Sheffield J., Goteti G. & Wood E. F. Development of a 50-year high-resolution global dataset of meteorological forcings for land surface modeling. J. Clim 19, 3088–3111 (2006).
-
- Abatzoglou J. T. Development of gridded surface meteorological data for ecological applications and modelling. Int. J. Climatol. 33, 121–131 (2013).
-
- Beck H. E. et al. MSWEP: 3-hourly 0.25 global gridded precipitation (1979-2015) by merging gauge, satellite, and reanalysis data. Hydrol. Earth Syst. Sci. 21, 589–615 (2017).
Publication types
LinkOut - more resources
Full Text Sources
Other Literature Sources