Replicability and Prediction: Lessons and Challenges from GWAS - PubMed
Review
Replicability and Prediction: Lessons and Challenges from GWAS
Urko M Marigorta et al. Trends Genet. 2018 Jul.
Abstract
Since the publication of the Wellcome Trust Case Control Consortium (WTCCC) landmark study a decade ago, genome-wide association studies (GWAS) have led to the discovery of thousands of risk variants involved in disease etiology. This success story has two angles that are often overlooked. First, GWAS findings are highly replicable. This is an unprecedented phenomenon in complex trait genetics, and indeed in many areas of science, which in past decades have been plagued by false positives. At a time of increasing concerns about the lack of reproducibility, we examine the biological and methodological reasons that account for the replicability of GWAS and identify the challenges ahead. In contrast to the exemplary success of disease gene discovery, at present GWAS findings are not useful for predicting phenotypes. We close with an overview of the prospects for individualized prediction of disease risk and its foreseeable impact in clinical practice.
Keywords: GWAS; genetic architecture; genetic risk score; prediction; replicability.
Copyright © 2018 Elsevier Ltd. All rights reserved.
Figures
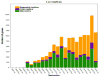
The graph reports the number of discoveries of new loci and re-discovery of previously discovered loci for 60 diseases included in the GWAS Catalog (quantitative traits were not considered; last accessed 17th March 2017). Data are classified according to the semester of publication (from 2005 to 2016). Given that all records included in the Catalog achieve at least P<10−5, all newly included SNP-disease pairs that had already been included because of being discovered by previous GWAS can be considered as replications of the first finding. We labelled these instances as re-discoveries, or replica. The re-discovery figure for a given semester corresponds to the total cumulative number since 2005, separated according to whether the re-discovery event constitutes the first evidence for replication of a given locus (‘first replica’) or if it had been previously replicated (‘repeated replica’). Given the diversity of arrays before the recent generalization of imputation in GWAS, SNPs are considered replicated either when the same SNP is re-discovered or another SNP in R2≥ 0.8 (using 1KG Europeans) within a +/− 500 kb window is reported. Only GWAS performed in Eurasian populations were evaluated.
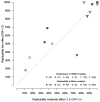
X-axis: Classified by disease, percentage of SNPs with a discovery OR between 1.2 and 1.5 that have been re-discovered at least once and included in the GWAS Catalog (filled circles) or that achieve nominal evidence of association (P<0.05) in a large meta-analysis published after the discovery GWAS (inverted triangles). Y-axis: for the same diseases, proportion of re-discovery for risk SNPs with discovery OR<1.2. Although the percentages vary by disease, the average re-discovery of low-effect variants is not significantly lower than that of variants with larger effect (P=0.93, Kolmogorov-Smirnov test) and overall re-discovery estimates are highly correlated (Spearman’s rho: 0.54, P=10−16).
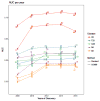
We calculated the area under the ROC curve (AUC) for five diseases using the samples from the WTCCC paper and SNPs discovered at different year ranges. X-axis: Discovery year of disease-associated variants included in the genetic risk score for each disease (based on year of inclusion in the GWAS Catalog). Y-axis: AUC using samples from the WTCCC case-control study. Performance of two predictors is shown, namely, a standard GRS (dashed lines) and a modified version of the GRS using the number of risk alleles times the weight for each SNP, as derived from the COMBI algorithm (solid lines). The number of SNPs used in each AUC calculation are indicated in the small numbers next to each point. Even if the number of risk SNPs has increased steadily, for both methodologies we observe a plateau in the predictive power of the genetic risk scores.
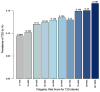
X-axis: 55,210 samples of European ancestry from the Kaiser GERA Cohort are classified according to deciles of PRS for type 2 diabetes (T2D). Y-axis: Percentage of patients in each category. The graph shows the impact of increasing deciles of a weighted genetic risk score based on 414 LD-pruned SNPs associated with T2D (P<10−3 in the 2014 trans-ethnic DIAGRAM GWAS). The PRS captures risk of disease according to genetic makeup of individuals, with a 2-fold enrichment of cases in the top vs. lowest decile.
Similar articles
-
Benefits and limitations of genome-wide association studies.
Tam V, Patel N, Turcotte M, Bossé Y, Paré G, Meyre D. Tam V, et al. Nat Rev Genet. 2019 Aug;20(8):467-484. doi: 10.1038/s41576-019-0127-1. Nat Rev Genet. 2019. PMID: 31068683 Review.
-
Uimari O, Rahmioglu N, Nyholt DR, Vincent K, Missmer SA, Becker C, Morris AP, Montgomery GW, Zondervan KT. Uimari O, et al. Hum Reprod. 2017 Apr 1;32(4):780-793. doi: 10.1093/humrep/dex024. Hum Reprod. 2017. PMID: 28333195 Free PMC article.
-
Using genome-wide pathway analysis to unravel the etiology of complex diseases.
Elbers CC, van Eijk KR, Franke L, Mulder F, van der Schouw YT, Wijmenga C, Onland-Moret NC. Elbers CC, et al. Genet Epidemiol. 2009 Jul;33(5):419-31. doi: 10.1002/gepi.20395. Genet Epidemiol. 2009. PMID: 19235186
-
Molecular genetic studies of complex phenotypes.
Marian AJ. Marian AJ. Transl Res. 2012 Feb;159(2):64-79. doi: 10.1016/j.trsl.2011.08.001. Epub 2011 Aug 31. Transl Res. 2012. PMID: 22243791 Free PMC article. Review.
-
[No authors listed] [No authors listed] Nat Genet. 2010 Jul;42(7):551. doi: 10.1038/ng0710-551. Nat Genet. 2010. PMID: 20581872
Cited by
-
Weller CA, Tilk S, Rajpurohit S, Bergland AO. Weller CA, et al. G3 (Bethesda). 2021 Apr 15;11(4):jkab062. doi: 10.1093/g3journal/jkab062. G3 (Bethesda). 2021. PMID: 33677482 Free PMC article.
-
A Multibreed Genome-Wide Association Study for Cattle Leukocyte Telomere Length.
Igoshin AV, Yudin NS, Romashov GA, Larkin DM. Igoshin AV, et al. Genes (Basel). 2023 Aug 7;14(8):1596. doi: 10.3390/genes14081596. Genes (Basel). 2023. PMID: 37628647 Free PMC article.
-
Assessment of clinically actionable pharmacogenetic markers to stratify anti-seizure medications.
Guin D, Hasija Y, Kukreti R. Guin D, et al. Pharmacogenomics J. 2023 Nov;23(6):149-160. doi: 10.1038/s41397-023-00313-y. Epub 2023 Aug 26. Pharmacogenomics J. 2023. PMID: 37626111
-
Impact of Genetic Variations on Thromboembolic Risk in Saudis with Sickle Cell Disease.
Alshabeeb MA, Alwadaani D, Al Qahtani FH, Abohelaika S, Alzahrani M, Al Zayed A, Al Saeed HH, Al Ajmi H, Alsomaie B, Rashid M, Daly AK. Alshabeeb MA, et al. Genes (Basel). 2023 Oct 9;14(10):1919. doi: 10.3390/genes14101919. Genes (Basel). 2023. PMID: 37895268 Free PMC article.
-
GWAS Meta-analysis of Kidney Function Traits in Japanese Populations.
Hishida A, Nakatochi M, Sutoh Y, Nakano S, Momozawa Y, Narita A, Tanno K, Shimizu A, Hozawa A, Kinoshita K, Yamaji T, Goto A, Noda M, Sawada N, Ikezaki H, Nagayoshi M, Hara M, Suzuki S, Koyama T, Koriyama C, Katsuura-Kamano S, Kadota A, Kuriki K, Yamamoto M, Sasaki M, Iwasaki M, Matsuo K, Wakai K. Hishida A, et al. J Epidemiol. 2024 Nov 5;34(11):526-534. doi: 10.2188/jea.JE20230281. Epub 2024 Sep 30. J Epidemiol. 2024. PMID: 38583947 Free PMC article.
References
-
- Maher B. Personal genomes: The case of the missing heritability. Nature. 2008;456(7218):18–21. - PubMed
Publication types
MeSH terms
LinkOut - more resources
Full Text Sources
Other Literature Sources
Miscellaneous