Signatures of ecological processes in microbial community time series - PubMed
- ️Mon Jan 01 2018
Signatures of ecological processes in microbial community time series
Karoline Faust et al. Microbiome. 2018.
Abstract
Background: Growth rates, interactions between community members, stochasticity, and immigration are important drivers of microbial community dynamics. In sequencing data analysis, such as network construction and community model parameterization, we make implicit assumptions about the nature of these drivers and thereby restrict model outcome. Despite apparent risk of methodological bias, the validity of the assumptions is rarely tested, as comprehensive procedures are lacking. Here, we propose a classification scheme to determine the processes that gave rise to the observed time series and to enable better model selection.
Results: We implemented a three-step classification scheme in R that first determines whether dependence between successive time steps (temporal structure) is present in the time series and then assesses with a recently developed neutrality test whether interactions between species are required for the dynamics. If the first and second tests confirm the presence of temporal structure and interactions, then parameters for interaction models are estimated. To quantify the importance of temporal structure, we compute the noise-type profile of the community, which ranges from black in case of strong dependency to white in the absence of any dependency. We applied this scheme to simulated time series generated with the Dirichlet-multinomial (DM) distribution, Hubbell's neutral model, the generalized Lotka-Volterra model and its discrete variant (the Ricker model), and a self-organized instability model, as well as to human stool microbiota time series. The noise-type profiles for all but DM data clearly indicated distinctive structures. The neutrality test correctly classified all but DM and neutral time series as non-neutral. The procedure reliably identified time series for which interaction inference was suitable. Both tests were required, as we demonstrated that all structured time series, including those generated with the neutral model, achieved a moderate to high goodness of fit to the Ricker model.
Conclusions: We present a fast and robust scheme to classify community structure and to assess the prevalence of interactions directly from microbial time series data. The procedure not only serves to determine ecological drivers of microbial dynamics, but also to guide selection of appropriate community models for prediction and follow-up analysis.
Keywords: Brown noise; Community dynamics; Community models; Neutrality test; Noise types; Pink noise; Time series analysis.
Conflict of interest statement
Ethics approval and consent to participate
Not applicable.
Consent for publication
Not applicable.
Competing interests
The authors declare that they have no competing interests.
Publisher’s Note
Springer Nature remains neutral with regard to jurisdictional claims in published maps and institutional affiliations.
Figures
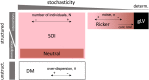
Overview of community models. The position of community models in this overview diagram is determined by two axes, which represent the importance given to structure and to noise, respectively. The first gradient orders models by the level of stochasticity, with neutral models at one extreme and the noise-free Ricker and generalized Lotka-Volterra model at the other. When increasing the strength of the noise or decreasing the number of individuals, deterministic models can move towards the stochastic end of the spectrum. The second axis orders models according to the role of structure, i.e., the strength of the dependency on previous time points. The Dirichlet-multinomial distribution and other probability distributions, which generate counts that do not depend on previous states, are at one end of the spectrum, whereas models with a high dependency on previous states, such as the generalized Lotka-Volterra, are at the other
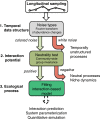
Classification of time series data. First, noise-type distributions are established. Darker noise colors indicate increasing temporal dependence between time points. White noise suggests random processes without temporal structure that can be caused for instance by technical bias such as insufficient sampling density or too large measurement noise, or reflect an intrinsic lack of time structure. Second, the presence of neutral dynamics are tested. A positive test result (p value below the significance level) suggests a potential for interactions in the microbial community and can be followed up for instance by fitting community models that assume interactions, by network analysis or by causal model approaches. For high levels of external noise or too large sampling intervals, the neutrality test may yield a false positive outcome. In neutral communities, variation can be suitably analyzed within stochastic frameworks
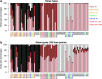
The noise-type profile distinguishes between temporally structured and unstructured community time series. a Noise types. b Noise types (100 time points). The bar plots depict for each community time series the percentage of species with white, pink, brown, or black noise. White noise indicates the absence of structure, all other noise types its presence. Labels for time series are colored according to the level of non-zero intrinsic noise (sigma) for Ricker, according to the death rate if larger than one for Hubbell, according to the interval if larger than one (with interval coloring taking precedence over sigma) and black otherwise time series for the full-length time series (a) as well as for shortened time series consisting of the first 100 time points (b)
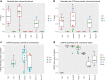
Neutrality test and LIMITS results. a The neutrality test distinguishes non-neutral from neutral time series. A long interval is a confounding factor. b The neutrality test also classifies short time series correctly. c The accuracy of the interaction matrix inferred with LIMITS is high for time series from the three deterministic models, but decreases with connectance. d The goodness of fit of time series to the Ricker model is high for all time series except for the unstructured DM data. The goodness of fit was quantified as the correlation between the original time series and the time series predicted with the Ricker model parameterized with LIMITS. Plots were made with ggplot2 [52]. The dashed lines in a and b indicate the value corresponding to a p value of 0.05. Values above represent significant p values, for which neutrality is rejected
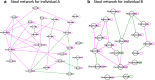
Inferred interactions in stool data sets. The inferred interaction matrices are represented as directed networks, where nodes are OTUs labeled with their genus or higher-level taxon name and directed edges represent non-zero entries in the inferred interaction matrix. Directed edges with positive signs are colored in green, those with negative in red. Orphan nodes are not shown. a In the network inferred for the stool time series of individual A, a negative hub is formed by a Faecalibacterium OTU. b In the network of individual B, the same Faecalibacterium OTU (OTU_165924) also forms a negative hub. Interaction matrices inferred with LIMITS were visualized with igraph [53]
Similar articles
-
Analytically tractable model for community ecology with many species.
Dickens B, Fisher CK, Mehta P. Dickens B, et al. Phys Rev E. 2016 Aug;94(2-1):022423. doi: 10.1103/PhysRevE.94.022423. Epub 2016 Aug 30. Phys Rev E. 2016. PMID: 27627348
-
Demographic analysis of Hubbell's neutral theory of biodiversity.
Allouche O, Kadmon R. Allouche O, et al. J Theor Biol. 2009 May 21;258(2):274-80. doi: 10.1016/j.jtbi.2008.12.024. Epub 2008 Dec 30. J Theor Biol. 2009. PMID: 19168081
-
A mathematical synthesis of niche and neutral theories in community ecology.
Haegeman B, Loreau M. Haegeman B, et al. J Theor Biol. 2011 Jan 21;269(1):150-65. doi: 10.1016/j.jtbi.2010.10.006. Epub 2010 Oct 12. J Theor Biol. 2011. PMID: 20946903
-
Inferring human microbial dynamics from temporal metagenomics data: Pitfalls and lessons.
Cao HT, Gibson TE, Bashan A, Liu YY. Cao HT, et al. Bioessays. 2017 Feb;39(2). doi: 10.1002/bies.201600188. Epub 2016 Dec 21. Bioessays. 2017. PMID: 28000336 Review.
-
Applying ecological models to communities of genetic elements: the case of neutral theory.
Linquist S, Cottenie K, Elliott TA, Saylor B, Kremer SC, Gregory TR. Linquist S, et al. Mol Ecol. 2015 Jul;24(13):3232-42. doi: 10.1111/mec.13219. Epub 2015 Jun 15. Mol Ecol. 2015. PMID: 25919906 Review.
Cited by
-
Microbiome time series data reveal predictable patterns of change.
Karwowska Z, Szczerbiak P, Kosciolek T. Karwowska Z, et al. Microbiol Spectr. 2024 Oct 3;12(10):e0410923. doi: 10.1128/spectrum.04109-23. Epub 2024 Aug 20. Microbiol Spectr. 2024. PMID: 39162505 Free PMC article.
-
Predicted Metabolic Function of the Gut Microbiota of Drosophila melanogaster.
Ankrah NYD, Barker BE, Song J, Wu C, McMullen JG 2nd, Douglas AE. Ankrah NYD, et al. mSystems. 2021 May 4;6(3):e01369-20. doi: 10.1128/mSystems.01369-20. mSystems. 2021. PMID: 33947801 Free PMC article.
-
Li C, Chng KR, Kwah JS, Av-Shalom TV, Tucker-Kellogg L, Nagarajan N. Li C, et al. Microbiome. 2019 Aug 22;7(1):118. doi: 10.1186/s40168-019-0729-z. Microbiome. 2019. PMID: 31439018 Free PMC article.
-
Microbial Growth under Limiting Conditions-Future Perspectives.
Gonzalez JM, Aranda B. Gonzalez JM, et al. Microorganisms. 2023 Jun 23;11(7):1641. doi: 10.3390/microorganisms11071641. Microorganisms. 2023. PMID: 37512814 Free PMC article. Review.
-
Schwan B, Abendroth C, Latorre-Pérez A, Porcar M, Vilanova C, Dornack C. Schwan B, et al. Front Microbiol. 2020 May 12;11:867. doi: 10.3389/fmicb.2020.00867. eCollection 2020. Front Microbiol. 2020. PMID: 32477297 Free PMC article.
References
Publication types
MeSH terms
LinkOut - more resources
Full Text Sources
Other Literature Sources