Feature contingencies when reading letter strings - PubMed
Feature contingencies when reading letter strings
Daniel R Coates et al. Vision Res. 2019 Mar.
Abstract
Many models posit the use of distinctive spatial features to recognize letters of the alphabet, a fundamental component of reading. It has also been hypothesized that when letters are in close proximity, visual crowding may cause features to mislocalize between nearby letters, causing identification errors. Here, we took a data-driven approach to investigate these aspects of textual processing. Using data collected from subjects identifying each letter in thousands of lower-case letter trigrams presented in the peripheral visual field, we found characteristic error patterns in the results suggestive of the use of particular spatial features. Distinctive features were seldom entirely missed, and we found evidence for errors due to doubling, masking, and migration of features. Dependencies both amongst neighboring letters and in the responses revealed the contingent nature of processing letter strings, challenging the most basic models of reading that ignore either crowding or featural decomposition.
Keywords: Crowding; Feature migration; Letter recognition; Peripheral vision; Redundancy masking.
Copyright © 2019 Elsevier Ltd. All rights reserved.
Figures
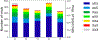
Summary of errors for each subject. In the figure legend, an “a” indicates a correct answer in the corresponding position (order: left, middle, right), while an ”X” indicates an error in that position. Incorrectly identifying the middle letter and correctly identifying the two outside letters was by far the most common error for all subjects, constituting nearly half of the error trials for each subject (or approximately a quarter of the total trials for each subject). Total number of trials are shown on the left axis, while the proportion of total trials are shown on the right axis. Each subject made an error in 42–55% of trials.
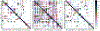
Aggregate confusion matrices for left, middle, and right letters, respectively. Cells are colored by occurrence from light/pastel to dark (see the color bar on the far right for values of occurrence). Letters presented are shown in rows while responses are shown in columns.
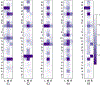
Proportion correct for each observer identifying each of the 26 letters presented in each position. Proportion is colored as indicated by scale bar, with darker colors indicating worse performance.
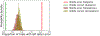
Illustration of Monte Carlo approach to statistical robustness determination of error contingencies (Section 3.1.4). The conditional likelihood of a subject making a left letter error is shown by the two vertical lines, conditioned on whether the middle letter was correctly identified (green vertical line) or erroneous (red vertical line). A left letter error was about 40% more likely when a middle letter error occurred, and simulations from the independence model (colored histograms) show that this difference was extremely unlikely to happen by chance.
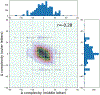
For trials with an error in the middle position and at least one outer letter, the summed change in complexity of the outer letters is plotted against the change in complexity of the middle letter. A positive number indicates that complexity is gained in the response. Each point represents a single trial. The correlation coefficient, given in the upper right corner, indicates a negative correlation—complexity gain/loss is balanced between letter positions.
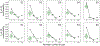
The average confusability of letters for each pair of middle and outer letters, plotted against the number of correct letters reported in the pair. Points and lines show the empirical data, while colored “violin plots” show the distributions resulting from 1000 simulations. Correct reports are negatively correlated with overall confusability, in both the data and the simulations.
Similar articles
-
The dependence of crowding on flanker complexity and target-flanker similarity.
Bernard JB, Chung ST. Bernard JB, et al. J Vis. 2011 Jul 5;11(8):10.1167/11.8.1 1. doi: 10.1167/11.8.1. J Vis. 2011. PMID: 21730225 Free PMC article.
-
Chung ST, Mansfield JS. Chung ST, et al. Vision Res. 2009 Nov;49(23):2782-9. doi: 10.1016/j.visres.2009.08.013. Epub 2009 Aug 18. Vision Res. 2009. PMID: 19695281 Free PMC article.
-
Learning to identify crowded letters: does it improve reading speed?
Chung ST. Chung ST. Vision Res. 2007 Nov;47(25):3150-9. doi: 10.1016/j.visres.2007.08.017. Epub 2007 Oct 24. Vision Res. 2007. PMID: 17928026 Free PMC article. Clinical Trial.
-
Visual crowding: a fundamental limit on conscious perception and object recognition.
Whitney D, Levi DM. Whitney D, et al. Trends Cogn Sci. 2011 Apr;15(4):160-8. doi: 10.1016/j.tics.2011.02.005. Epub 2011 Mar 21. Trends Cogn Sci. 2011. PMID: 21420894 Free PMC article. Review.
-
Crowding: a neuroanalytic approach.
Tyler CW, Likova LT. Tyler CW, et al. J Vis. 2007 Jul 30;7(2):16.1-9. doi: 10.1167/7.2.16. J Vis. 2007. PMID: 18217831 Review.
Cited by
-
The generality of the critical spacing for crowded optotypes: From Bouma to the 21st century.
Coates DR, Ludowici CJH, Chung STL. Coates DR, et al. J Vis. 2021 Oct 5;21(11):18. doi: 10.1167/jov.21.11.18. J Vis. 2021. PMID: 34694326 Free PMC article.
-
Yildirim FZ, Coates DR, Sayim B. Yildirim FZ, et al. Sci Rep. 2021 Feb 18;11(1):4095. doi: 10.1038/s41598-021-83325-7. Sci Rep. 2021. PMID: 33602975 Free PMC article.
-
Geometrically restricted image descriptors: A method to capture the appearance of shape.
Melnik N, Coates DR, Sayim B. Melnik N, et al. J Vis. 2021 Mar 1;21(3):14. doi: 10.1167/jov.21.3.14. J Vis. 2021. PMID: 33688921 Free PMC article.
-
Theiss JD, Bowen JD, Silver MA. Theiss JD, et al. Neural Comput. 2021 Dec 15;34(1):190-218. doi: 10.1162/neco_a_01447. Neural Comput. 2021. PMID: 34710898 Free PMC article.
-
Redundancy masking: The loss of repeated items in crowded peripheral vision.
Yildirim FZ, Coates DR, Sayim B. Yildirim FZ, et al. J Vis. 2020 Apr 9;20(4):14. doi: 10.1167/jov.20.4.14. J Vis. 2020. PMID: 32330230 Free PMC article.
References
-
- Attneave F, Arnoult MD (1956). The quantitative study of shape and pattern perception. Psychological bulletin, 53, 452. - PubMed
-
- Bouma H (1970). Interaction effects in parafoveal letter recognition. Nature, 226, 177–178. - PubMed
-
- Bouma H (1971). Visual recognition of isolated lower-case letters. Vision Research, 11, 459–474. - PubMed