Towards a unified model of event-related potentials as phases of stimulus-to-response processing - PubMed
Towards a unified model of event-related potentials as phases of stimulus-to-response processing
Brittany K Taylor et al. Neuropsychologia. 2019 Sep.
Abstract
This study demonstrates the utility of combining principles of connectionist theory with a sophisticated statistical approach, structural equation modeling (SEM), to better understand brain-behavior relationships in studies using event-related potentials (ERPs). The models show how sequential phases of neural processing measured by averaged ERP waveform components can successfully predict task behavior (response time; RT) while accounting for individual differences in maturation and sex. The models assume that all ERP measures are affected by individual differences in physical and mental state that inflate measurement error. ERP data were collected from 154 neurotypical children (7-13 years, M = 10.22, SD = 1.48; 74 males) performing a cued Go/No-Go task during two separate sessions. Using SEM, we show a latent variable path model with good fit (e.g., χ2(51) = 56.20, p = .25; RMSEA = .03; CFI = .99; SRMR = .06) yielding moderate-to-large predictive coefficients from N1 through the E-wave latent variables (N1 β = -.29 → P2 β = -.44 → N2 β = .28 → P3 β =.64→ E-wave), which in turn significantly predicted RT (β =.34, p = .02). Age significantly related to N1 and P3 latent variables as well as RT (β =.31, -.58, & -.40 respectively), and Sex significantly related to the E-wave latent variable and RT (β =.36 & 0.21 respectively). Additionally, the final model suggested that individual differences in emotional and physical state accounted for a significant proportion of variance in ERP measurements, and that individual states systematically varied across sessions (i.e., the variance was not just random noise). These findings suggest that modeling ERPs as a system of inter-related processes may be a more informative approach to examining brain-behavior relationships in neurotypical and clinical groups than traditional analysis techniques.
Keywords: Development; Event-related potentials (ERPs); Individual differences; Structural equation modeling (SEM).
Copyright © 2019 Elsevier Ltd. All rights reserved.
Figures
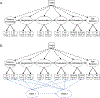
The two conceptual models being evaluated in this study. Model “A” depicts how both trait characteristics of the individual and a task behavior (e.g. response time) may relate to brain activity. State effects for manifest variable are assumed to be random and therefore are subsumed in the residual variances of the manifest measures. Model “B” assumes the same relationships between trait characteristics, task behavior, and brain activity as “A” but includes the assumption that nonrandom state effects are measurable for each assessment session. In both models, components from averaged ERPs of correct trials in the contingent negative variation (CNV) ERP paradigm and the averaged response time obtained in two separate sessions serve as the manifest variables (squares) used to create the latent variables (ovals). Solid arrows show relationships expected to be statistically significant and dashed arrows depict relationships that may or may not be statistically significant depending on nature of the cognitive task, the specific parameters of the task, and the characteristics of the participants.
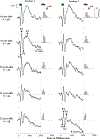
The grand averaged event-related potentials (ERPs) for session 1 and session 2 Go trials separated by age group. All ERP images were derived at site Cz. The frequency distribution of average response times for each session and age group is shown to the right of each ERP. Note: there were very few children that were 7- or 13-years-old, and those children’s data were grouped with the 8- and 12-year-olds’ data, respectively. Note: The data shown in the figure represent the data included in the models: all children’s response times, and only the averaged ERPs for children who had adequate data for that session are included in each figure. N’s for each age group indicate the total number of children in that age group.
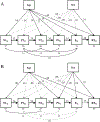
The two manifest path models of neural processing predicting a task behavior (e.g. response time) while controlling for variance attributed to two trait characteristics of the individuals (Age and Sex): A depicts outcomes for Session 1 Go trials; and B depicts outcomes for Session 2 Go trials. Gray dotted arrows indicate non-statistically significant relationships, i.e., p > .05. Residual variances are reported below each variable. All reported coefficients are standardized.
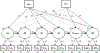
The latent variable model of neural processing predicting a task behavior (e.g. response time) while controlling for variance attributed to two trait characteristics of the individuals (Age and Sex). Gray dotted arrows indicate non-statistically significant relationships, i.e., p > .05. Residual variances are reported below each manifest variable, and disturbances are reported at the upper left of each latent variable. All reported coefficients are standardized. Note: S1 = session 1 measure, S2 = session 2 measure; ES1 = E-wave amplitude in session 1, ES2 = E-wave amplitude in session 2.
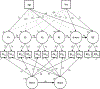
The latent variable model of neural processing predicting a task behavior (e.g. response time) while controlling for variance attributed to changes in individual states between the sessions as well variance due to two trait characteristics of the individuals (Age and Sex). Gray dotted arrows indicate non-statistically significant relationships, i.e., p > .05. Residual variances are reported below each manifest variable, and disturbances are reported at the upper left of each latent variable. All reported coefficients are standardized. Note: S1 = session 1 measure, S2 = session 2 measure, ES1 = E-wave amplitude in session 1, ES2 = E-wave amplitude in session 2.
Similar articles
-
Lin MH, Davies PL, Taylor BK, Prince MA, Gavin WJ. Lin MH, et al. Psychophysiology. 2022 Mar;59(3):e13972. doi: 10.1111/psyp.13972. Epub 2021 Nov 24. Psychophysiology. 2022. PMID: 34818441
-
Taylor BK, Gavin WJ, Grimm KJ, Passantino DE, Davies PL. Taylor BK, et al. Neuropsychologia. 2018 Jan 31;109:222-231. doi: 10.1016/j.neuropsychologia.2017.12.025. Epub 2017 Dec 15. Neuropsychologia. 2018. PMID: 29253492 Free PMC article.
-
Quinzi F, Perri RL, Berchicci M, Bianco V, Pitzalis S, Zeri F, Di Russo F. Quinzi F, et al. Biol Psychol. 2018 Oct;138:211-222. doi: 10.1016/j.biopsycho.2018.08.014. Epub 2018 Aug 18. Biol Psychol. 2018. PMID: 30130614
-
Internal and external control: a two-factor model of amplitude change of event-related potentials.
Kok A. Kok A. Acta Psychol (Amst). 1990 Aug;74(2-3):203-36. Acta Psychol (Amst). 1990. PMID: 2251929 Review.
-
Gajewski PD, Ferdinand NK, Kray J, Falkenstein M. Gajewski PD, et al. Neurosci Biobehav Rev. 2018 Sep;92:255-275. doi: 10.1016/j.neubiorev.2018.05.029. Epub 2018 Jun 6. Neurosci Biobehav Rev. 2018. PMID: 29885425 Review.
Cited by
-
Lin MH, Davies PL, Stephens J, Gavin WJ. Lin MH, et al. Dev Neuropsychol. 2020 Sep;45(6):341-366. doi: 10.1080/87565641.2020.1833208. Epub 2020 Oct 20. Dev Neuropsychol. 2020. PMID: 33078653 Free PMC article.
-
Automaticity of Early Sexual Attention: An Event-Related Potential Study.
Ziogas A, Habermeyer B, Kawohl W, Habermeyer E, Mokros A. Ziogas A, et al. Sex Abuse. 2022 Aug;34(5):507-536. doi: 10.1177/10790632211024241. Epub 2021 Jul 8. Sex Abuse. 2022. PMID: 34235992 Free PMC article.
-
Taylor BK, Heinrichs-Graham E, Eastman JA, Frenzel MR, Wang YP, Calhoun VD, Stephen JM, Wilson TW. Taylor BK, et al. Neuroimage. 2022 Jun;253:119094. doi: 10.1016/j.neuroimage.2022.119094. Epub 2022 Mar 16. Neuroimage. 2022. PMID: 35306160 Free PMC article.
-
Smigielski L, Kometer M, Scheidegger M, Stress C, Preller KH, Koenig T, Vollenweider FX. Smigielski L, et al. Hum Brain Mapp. 2020 Dec;41(17):4982-4996. doi: 10.1002/hbm.25174. Epub 2020 Aug 21. Hum Brain Mapp. 2020. PMID: 32820851 Free PMC article. Clinical Trial.
References
-
- American Electroencephalographic Society, 1994. Guidelines for standard electrode position nomenclature. Journal of Clinical Neurophysiology, 11, 111–113. - PubMed
-
- Bear MF, & Cooper LN (1990). Molecular mechanisms for synaptic modification in visual cortex: Interaction between theory and experiment In Gluck MA & Rumelhart DE (Eds.), Neuroscience and connectionist theory (pp. 65–94). Hillsdale, NJ, England: Lawrence Erlbaum Associates, Inc.
Publication types
MeSH terms
LinkOut - more resources
Full Text Sources
Medical
Miscellaneous