Large-Scale Exome Sequencing Study Implicates Both Developmental and Functional Changes in the Neurobiology of Autism - PubMed
- ️Wed Jan 01 2020
. 2020 Feb 6;180(3):568-584.e23.
doi: 10.1016/j.cell.2019.12.036. Epub 2020 Jan 23.
Jack A Kosmicki 2 , Jiebiao Wang 3 , Michael S Breen 4 , Silvia De Rubeis 4 , Joon-Yong An 5 , Minshi Peng 3 , Ryan Collins 6 , Jakob Grove 7 , Lambertus Klei 8 , Christine Stevens 9 , Jennifer Reichert 10 , Maureen S Mulhern 10 , Mykyta Artomov 9 , Sherif Gerges 9 , Brooke Sheppard 11 , Xinyi Xu 10 , Aparna Bhaduri 12 , Utku Norman 13 , Harrison Brand 14 , Grace Schwartz 11 , Rachel Nguyen 15 , Elizabeth E Guerrero 16 , Caroline Dias 17 ; Autism Sequencing Consortium; iPSYCH-Broad Consortium; Catalina Betancur 18 , Edwin H Cook 19 , Louise Gallagher 20 , Michael Gill 20 , James S Sutcliffe 21 , Audrey Thurm 22 , Michael E Zwick 23 , Anders D Børglum 24 , Matthew W State 11 , A Ercument Cicek 25 , Michael E Talkowski 14 , David J Cutler 23 , Bernie Devlin 8 , Stephan J Sanders 26 , Kathryn Roeder 27 , Mark J Daly 28 , Joseph D Buxbaum 29
Collaborators, Affiliations
- PMID: 31981491
- PMCID: PMC7250485
- DOI: 10.1016/j.cell.2019.12.036
Large-Scale Exome Sequencing Study Implicates Both Developmental and Functional Changes in the Neurobiology of Autism
F Kyle Satterstrom et al. Cell. 2020.
Abstract
We present the largest exome sequencing study of autism spectrum disorder (ASD) to date (n = 35,584 total samples, 11,986 with ASD). Using an enhanced analytical framework to integrate de novo and case-control rare variation, we identify 102 risk genes at a false discovery rate of 0.1 or less. Of these genes, 49 show higher frequencies of disruptive de novo variants in individuals ascertained to have severe neurodevelopmental delay, whereas 53 show higher frequencies in individuals ascertained to have ASD; comparing ASD cases with mutations in these groups reveals phenotypic differences. Expressed early in brain development, most risk genes have roles in regulation of gene expression or neuronal communication (i.e., mutations effect neurodevelopmental and neurophysiological changes), and 13 fall within loci recurrently hit by copy number variants. In cells from the human cortex, expression of risk genes is enriched in excitatory and inhibitory neuronal lineages, consistent with multiple paths to an excitatory-inhibitory imbalance underlying ASD.
Keywords: autism spectrum disorder; cell type; cytoskeleton; excitatory neurons; excitatory-inhibitory balance; exome sequencing; genetics; inhibitory neurons; liability; neurodevelopment.
Copyright © 2020 Elsevier Inc. All rights reserved.
Conflict of interest statement
Declaration of Interests B.M.N. is a member of the scientific advisory board at Deep Genomics and consults for Biogen, Camp4 Therapeutics Corporation, Takeda Pharmaceutical, and Biogen. During the last 3 years, C.M. Freitag has been consultant to Desitin and Roche and receives royalties for books on ASD, ADHD, and MDD.
Figures
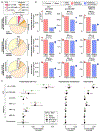
(A) The proportion of rare autosomal genetic variants split by predicted functional consequences, represented by color, is displayed for family-based (split into de novo and inherited variants) and case-control data. PTVs and missense variants are split into three tiers of predicted functional severity, represented by shade, based on the pLI and MPC metrics, respectively. (B) The relative difference in variant frequency (i.e., burden) between ASD cases and controls (top and bottom) or transmitted and untransmitted parental variants (center) is shown for the top two tiers of functional severity for PTVs (left and center) and the top tier of functional severity for missense variants (right). Next to the bar plot, the same data are shown divided by sex. (C) The relative difference in variant frequency shown in (B) is converted to a trait liability Z score, split by the same subsets used in (A). For context, a Z score of 2.18 would shift an individual from the population mean to the top 1.69% of the population (equivalent to an ASD threshold based on 1 in 68 children; Christensen et al., 2016). No significant difference in liability was observed between males and females for any analysis. Statistical tests: (B) and (C), binomial exact test (BET) for most contrasts; exceptions were “both” and “case-control,” for which Fisher’s method for combining BET p values for each sex and, for case-control, each population was used; p values corrected for 168 tests are shown.
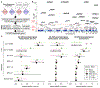
(A) WES data from 35,584 samples are entered into a Bayesian analysis framework (TADA) that incorporates pLI score for PTVs and MPC score for missense variants. (B) The model identifies 102 autosomal genes associated with ASD at a false discovery rate (FDR) threshold of 0.1 or less, which is shown on the y axis of this Manhattan plot, with each point representing a gene. Of these, 78 pass the threshold FDR of 0.05 or less, and 26 pass the threshold family-wise error rate (FWER) of 0.05 or less. (C) Repeating our ASD trait liability analysis (Figure 1C) for variants observed within the 102 ASD-associated genes only. Statistical tests: (B), TADA; (C), BET for most contrasts; exceptions were “both” and “case-control,” for which Fisher’s method for combining BET p values for each sex and, for case-control, each population was used; p values corrected for 168 tests are shown.
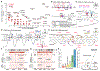
(A) Count of PTVs versus missensevariants(MPC ≥ 1) in cases for each ASD-associated gene (red points, selected genes labeled). These counts reflect the data used by TADA for association analysis: de novo and case-control data for PTVs; de novo only for missense. (B) Location of ASD de novo missense variants in DEAF1. The five ASD variants (marked in red) are in the SAND (Sp100, AIRE-1, NucP41/75, DEAF-1) DNA-binding domain (amino acids 193–273, spirals show α helices, arrows show β sheets, KDWK isthe DNA-binding motif) alongside 10 variants observed in NDD, several of which have been shown to reduce DNA binding, including Q264P and Q264R (Chen et al., 2017; Heyne et al., 2018; Vultovan Silfhout et al., 2014). (C) Location of ASD missensevariants in KCNQ3. All four ASD variants are located in the voltage sensor (fourth of six transmembrane domains), with three in the same residue (R230), including the gain-of-function R230C mutation observed in NDD (Heyne et al., 2018; Miceli et al, 2015). Five inherited variants observed in benign infantile seizures are shown in the pore loop (Landrum et al., 2014; Maljevic et al., 2016). (D) Location of ASD missense variants in SCN1A along side 17 de novo variants in NDD and epilepsy (Heyne et al., 2018). (E) Location of ASD missense variants in SLC6A1 along side 31 de novo variants in NDD and epilepsy (Heyne et al., 2018; Johannesen et al., 2018). (F) Subtelomeric 2q37 deletions are associated with facial dysmorphisms, brachydactyly, high BMI, NDD, and ASD (Leroy et al., 2013). Although three genes within the locus have a pLI score of 0.995 or higher, only HDLBP is associated with ASD. (G) Deletions atthe 11q13.2–q13.4 locus have been observed in NDD, ASD, and otodental dysplasia (Coe et al., 2014; Cooperet al., 2011). Five genes within the locus have a pLI score of 0.995 or higher, including two ASD genes: KMT5B and SHANK2. (H) Assessment of gene-based enrichment, via MAGMA, of 102 ASD genes against genome-wide significant common variants from six GWASs. (I) Gene-based enrichment of 102 ASD genes in multiple GWASs as a function of effective cohort size. The GWAS used for each disorder in (I) has a black outline. Statistical tests: (F) and (G), TADA; (H) and (I), MAGMA.
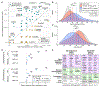
(A) Frequency of disruptive de novo variants (e.g., PTVs or missense variants with MPC ≥ 1) in ASD-ascertained and NDD-ascertained cohorts (Table S4) is shown for the 102 ASD-associated genes (selected genes labeled). Fifty genes with a higher frequency in ASD are designated ASD-predominant (ASDp), whereas the 49 genes more frequently mutated in NDD are designated as ASDNDD. Three genes marked with a star(UBR1, MAP1A, and NUP155) are included in the ASDP category on the basis of case-control data (Table S4), which are not shown here. Of the 26 FWER genes, 10 are ASDp and 16 are ASDNDD. Of the 102 genes, 13 demonstrate nominally significant heterogeneity between samples ascertained for ASD versus NDD (Table S4). (B) ASD cases with disruptive de novo variants in ASD genes show delayed walking compared with ASD cases without such de novo variants, and the effect is greater for those with disruptive de novo variants in ASDNDD genes. (C) Similarly, cases with disruptive de novo variants in ASDNDD genes and, to a lesser extent, ASDP genes have a lower full-scale IQ (FSIQ) than other ASD cases. (D) Despite the association between de novo variants in ASD genes and cognitive impairment shown in (C), an excess of disruptive de novo variants is observed in cases without intellectual disability (FSIQ ≥ 70) or with an IQ above the cohort mean (FSIQ ≥ 82). (E) Along with the phenotypic division (A), genes can also be classified functionally into four groups (gene expression regulation [GER], neuronal communication [NC], cytoskeleton, and other) based on Gene Ontology and research literature. The 102 ASD risk genes are shown in a mosaic plot divided by gene function and, from (A), the ASD versus NDD variant frequency, with the area of each box proportional to the number of genes. Statistical tests: (B) and (C), t test; (D), chi-square test with 1° of freedom.
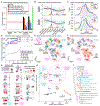
(A) GTEx bulk RNA-seq data from 53 tissues were processed to identify genes enriched in specific tissues. Gene set enrichment was performed for the 102 ASD genes and four subsets (ASDP, ASDNDD, GER, and NC) for each tissue. Five representative tissues are shown here, including cortex, which has the greatest degree of enrichment (OR = 3.7; p = 2.6 × 10−6). (B) BrainSpan bulk RNA-seq data across 10 developmental stages was used to plot the normalized expression of the 101 cortically expressed ASD genes (excluding PAX5, which is not expressed in the cortex) across development, split by the four subsets. (C) A t-statistic was calculated, comparing prenatal with postnatal expression in the BrainSpan data. The t-statistic distribution of 101 ASD-associated genes shows a prenatal bias (p = 8 × 10−8) for GER genes (p = 9 × 10−15), whereas NC genes are postnatally biased (p = 0.03). (D) The cumulative number of ASD-associated genes expressed in RNA-seq data for 4,261 cells collected from human forebrain across prenatal development (Nowakowski et al., 2017). (E) t-SNE analysis identifies 19 clusters with unambiguous cell type in these single-cell expression data. (F) The enrichment of the 102 ASD-associated genes within cells of each type is represented by color. The most consistent enrichment is observed in maturing and mature excitatory (bottom center) and inhibitory (top right) neurons. (G) The developmental relationships of the 19 clusters are indicated by black arrows, with the inhibitory lineage shown on the left (cyan), excitatory lineage in the middle (magenta), and non-neuronal cell types on the right (gray). The proportion of the 102 ASD-associated genes observed in at least 25% of cells within the cluster is shown by the pie chart, whereas the log-transformed Bonferroni-corrected p value of gene set enrichment is shown by the size of the red circle. (H) The relationship between the number of cells in the cluster (x axis) and the p value for ASD gene enrichment (y axis) is shown for the 19 cell type clusters. Linear regression indicates that clusters with few expressed genes (e.g., C23 newborn inhibitory neurons) have higher p valuesthan clusters with many genes (e.g., C25 radial glia). (I) The relationship between the 19 cell type clusters using hierarchical clustering based on the 10% of genes with the greatest variability among cell types. Statistical tests: (A), t test; (C), Wilcoxon test; (E), (F), (H), and (I), FET.
Comment in
-
High-Risk, High-Reward Genetics in ASD.
Castellani CA, Arking DE. Castellani CA, et al. Neuron. 2020 Feb 5;105(3):407-410. doi: 10.1016/j.neuron.2020.01.007. Neuron. 2020. PMID: 32027831 Free PMC article.
-
Autism Genetics: Over 100 Risk Genes and Counting.
Forrest MP, Penzes P. Forrest MP, et al. Pediatr Neurol Briefs. 2020 Dec 4;34:13. doi: 10.15844/pedneurbriefs-34-13. Pediatr Neurol Briefs. 2020. PMID: 33304087 Free PMC article.
Similar articles
-
Kim N, Kim KH, Lim WJ, Kim J, Kim SA, Yoo HJ. Kim N, et al. Genes (Basel). 2020 Dec 22;12(1):1. doi: 10.3390/genes12010001. Genes (Basel). 2020. PMID: 33374967 Free PMC article.
-
Wu R, Li X, Meng Z, Li P, He Z, Liang L. Wu R, et al. Orphanet J Rare Dis. 2024 May 19;19(1):205. doi: 10.1186/s13023-024-03214-w. Orphanet J Rare Dis. 2024. PMID: 38764027 Free PMC article.
-
Yuan B, Wang M, Wu X, Cheng P, Zhang R, Zhang R, Yu S, Zhang J, Du Y, Wang X, Qiu Z. Yuan B, et al. Neurosci Bull. 2023 Oct;39(10):1469-1480. doi: 10.1007/s12264-023-01037-6. Epub 2023 Mar 7. Neurosci Bull. 2023. PMID: 36881370 Free PMC article.
-
A de novo convergence of autism genetics and molecular neuroscience.
Krumm N, O'Roak BJ, Shendure J, Eichler EE. Krumm N, et al. Trends Neurosci. 2014 Feb;37(2):95-105. doi: 10.1016/j.tins.2013.11.005. Epub 2013 Dec 30. Trends Neurosci. 2014. PMID: 24387789 Free PMC article. Review.
-
Genetic architecture of autism spectrum disorder: Lessons from large-scale genomic studies.
Choi L, An JY. Choi L, et al. Neurosci Biobehav Rev. 2021 Sep;128:244-257. doi: 10.1016/j.neubiorev.2021.06.028. Epub 2021 Jun 21. Neurosci Biobehav Rev. 2021. PMID: 34166716 Review.
Cited by
-
Monoallelic loss-of-function variants in GSK3B lead to autism and developmental delay.
Tan S, Zhang Q, Zhan R, Luo S, Han Y, Yu B, Muss C, Pingault V, Marlin S, Delahaye A, Peters S, Perne C, Kreiß M, Spataro N, Trujillo-Quintero JP, Racine C, Tran-Mau-Them F, Phornphutkul C, Besterman AD, Martinez J, Wang X, Tian X, Srivastava S, Urion DK, Madden JA, Saif HA, Morrow MM, Begtrup A, Li X, Jurgensmeyer S, Leahy P, Zhou S, Li F, Hu Z, Tan J, Xia K, Guo H. Tan S, et al. Mol Psychiatry. 2024 Oct 29. doi: 10.1038/s41380-024-02806-z. Online ahead of print. Mol Psychiatry. 2024. PMID: 39472663
-
Current Approaches and Future Directions for the Treatment of mTORopathies.
Karalis V, Bateup HS. Karalis V, et al. Dev Neurosci. 2021;43(3-4):143-158. doi: 10.1159/000515672. Epub 2021 Apr 28. Dev Neurosci. 2021. PMID: 33910214 Free PMC article. Review.
-
A predictive ensemble classifier for the gene expression diagnosis of ASD at ages 1 to 4 years.
Bao B, Zahiri J, Gazestani VH, Lopez L, Xiao Y, Kim R, Wen TH, Chiang AWT, Nalabolu S, Pierce K, Robasky K, Wang T, Hoekzema K, Eichler EE, Lewis NE, Courchesne E. Bao B, et al. Mol Psychiatry. 2023 Feb;28(2):822-833. doi: 10.1038/s41380-022-01826-x. Epub 2022 Oct 20. Mol Psychiatry. 2023. PMID: 36266569 Free PMC article.
-
Dynamic convergence of autism disorder risk genes across neurodevelopment.
Garcia MF, Retallick-Townsley K, Pruitt A, Davidson E, Dai Y, Fitzpatrick SE, Sen A, Cohen S, Livoti O, Khan S, Dossou G, Cheung J, Deans PJM, Wang Z, Huckins L, Hoffman E, Brennand K. Garcia MF, et al. bioRxiv [Preprint]. 2024 Aug 24:2024.08.23.609190. doi: 10.1101/2024.08.23.609190. bioRxiv. 2024. PMID: 39229156 Free PMC article. Preprint.
-
Feng S, Huang H, Wang N, Wei Y, Liu Y, Qin D. Feng S, et al. Front Behav Neurosci. 2021 May 20;15:673372. doi: 10.3389/fnbeh.2021.673372. eCollection 2021. Front Behav Neurosci. 2021. PMID: 34093147 Free PMC article. Review.
References
-
- Baio J, Wiggins L, Christensen DL, Maenner MJ, Daniels J, Warren Z, Kurzius-Spencer M, Zahorodny W, Robinson Rosenberg C, White T, et al. (2018). Prevalence of Autism Spectrum Disorder Among Children Aged 8 Years - Autism and Developmental Disabilities Monitoring Network, 11 Sites, United States, 2014. MMWR Surveill Summ. 67, 1–23. - PMC - PubMed
-
- Battle A, Brown CD, Engelhardt BE, and Montgomery SB; GTEx Consortium; Laboratory, Data Analysis &Coordinating Center (LDACC)—Analysis Working Group; Statistical Methods groups—Analysis Working Group; Enhancing GTEx (eGTEx) groups; NIH Common Fund; NIH/NCI; NIH/NHGRI; NIH/NIMH; NIH/NIDA; Biospecimen Collection Source Site—NDRI; Biospecimen Collection Source Site—RPCI; Biospecimen Core Resource—VARI; Brain Bank Repository—University of Miami Brain Endowment Bank; Leidos Biomedical—Project Management; ELSI Study; Genome Browser Data Integration &Visualization — EBI; Genome Browser Data Integration &Visualization — UCSC Genomics Institute, University of California Santa Cruz; Lead analysts; Laboratory, Data Analysis &Coordinating Center (LDACC); NIH program management; Biospecimen collection; Pathology; eQTL manuscript working group (2017). Genetic effects on gene expression across human tissues. Nature 550, 204–213. - PubMed
Publication types
MeSH terms
Grants and funding
- R01 MH110928/MH/NIMH NIH HHS/United States
- R01 MH106910/MH/NIMH NIH HHS/United States
- UM1 HG008895/HG/NHGRI NIH HHS/United States
- U01 MH111660/MH/NIMH NIH HHS/United States
- T32 HG002295/HG/NHGRI NIH HHS/United States
- R01 MH057881/MH/NIMH NIH HHS/United States
- U01 MH111658/MH/NIMH NIH HHS/United States
- U01 MH111661/MH/NIMH NIH HHS/United States
- U01 MH111662/MH/NIMH NIH HHS/United States
- R56 MH115957/MH/NIMH NIH HHS/United States
- R01 MH095797/MH/NIMH NIH HHS/United States
- R01 MH115957/MH/NIMH NIH HHS/United States
- R37 MH057881/MH/NIMH NIH HHS/United States
- R01 MH097849/MH/NIMH NIH HHS/United States
- R01 MH109900/MH/NIMH NIH HHS/United States
- R24 ES028533/ES/NIEHS NIH HHS/United States
- U01 MH100233/MH/NIMH NIH HHS/United States
- R01 MH113362/MH/NIMH NIH HHS/United States