Normative theory of visual receptive fields - PubMed
- ️Fri Jan 01 2021
Normative theory of visual receptive fields
Tony Lindeberg. Heliyon. 2021.
Abstract
This article gives an overview of a normative theory of visual receptive fields. We describe how idealized functional models of early spatial, spatio-chromatic and spatio-temporal receptive fields can be derived in a principled way, based on a set of axioms that reflect structural properties of the environment in combination with assumptions about the internal structure of a vision system to guarantee consistent handling of image representations over multiple spatial and temporal scales. Interestingly, this theory leads to predictions about visual receptive field shapes with qualitatively very good similarities to biological receptive fields measured in the retina, the LGN and the primary visual cortex (V1) of mammals.
Keywords: Affine covariance; Double-opponent cell; Functional model; Galilean covariance; Gaussian derivative; Illumination invariance; LGN; Primary visual cortex; Receptive field; Retina; Scale covariance; Simple cell; Temporal causality; Vision.
© 2021 The Author(s).
Conflict of interest statement
The authors declare no conflict of interest.
Figures
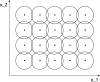
A traditional definition of the notion of a receptive field is as a region in the visual field for which a visual sensor/neuron/operator responds to visual stimuli. In this figure, we have illustrated a set of receptive fields over the spatial domain that partially overlap, and where all the receptive fields have the same size. More generally, we could consider distributions of receptive fields over space or space-time that have varying sizes, shapes and orientations in image space as well as having different directions in joint space-time. Adjacent receptive fields could also have substantially larger relative overlap than displayed here. In this work, we focus on a functional description of such linear receptive fields, concerning how a neuron responds to visual stimuli over image space regarding spatial receptive fields or over joint space-time regarding spatio-temporal receptive fields.
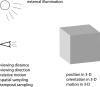
Basic factors that influence the formation of images for an eye with a two-dimensional retina that observes objects in the three-dimensional world. In addition to the position, the orientation and the motion of the object in 3-D, the perspective projection onto the retina is affected by the viewing distance, the viewing direction and the relative motion of the eye in relation to the object, the spatial and the temporal sampling characteristics of the neurons in the retina as well the usually unknown external illumination field in relation to the geometry of the scene and the observer.
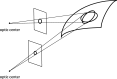
Illustration of the importance of covariance of the receptive field responses under natural image transformations. Consider a vision system that computes image features from image data based on image operations that are formulated over rotationally symmetric support regions in the spatial image domain. If such image measurements are performed for two different viewing directions relative to the same three-dimensional surface patch, then the backprojections of the image operations onto the tangent plane surface of the object will, in general, correspond to different regions in physical space over which corresponding information will be weighted differently. If such image features are in turn to be used for deriving three-dimensional shape cues of the object from binocular cues, such as surface orientation, then there will be a systematic error caused by the mismatch between the backprojections of the receptive fields from the image domain onto the world. By requiring the family of receptive fields to be covariant under local affine image deformations, it is possible to reduce this amount of mismatch, such that the backprojected receptive fields can be made equal, when projected onto the tangent plane of the surface by local linearizations of the perspective mapping. In this way, the source to error caused by mismatch between the two different receptive fields is eliminated. Corresponding effects occur when analyzing spatio-temporal image data based on receptive fields that are restricted to being space-time separable only. If an object is observed over time from two observations having different relative motions between the viewing direction and the observer, then the corresponding receptive fields cannot be matched unless the family of receptive fields possesses sufficient covariance properties under local Galilean transformations.
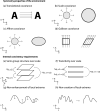
Schematic illustration of main assumptions underlying the proposed normative theory for visual receptive fields, regarding (i) transformation properties of the environment and (ii) internal consistency requirements to guarantee internally consistent image representations over multiple spatial and temporal scales. (a) Translational covariance means that visual representations of objects should be processed in a similar manner over the entire visual field. (b) Scale covariance means that scaling transformations, as occur in the visual domain because of objects of different size and objects at different distances to the observer, should be processed in a similar manner such that the receptive field responses can be matched. (c) Affine covariance is a generalization of scale covariance to non-uniform scaling transformations, as occur when surface structures are foreshortened for surfaces with a non-frontal slant angle relative to the tangent plane of the surface. (d) Galilean covariance means that if we observe objects or events that move relative to a fixed viewing direction, then these visual patterns should be processed in a conceptually similar way as if we observe the same patterns with the gaze direction following the same objects or events, and in such a way that the two types of spatio-temporal image representations can be matched. (e) The assumption of a semi-group structure over spatial scales implies that with a spatial smoothing operation in terms of convolution operations, which follows from a combination of the assumptions of translational covariance and linearity, the composition of two spatial smoothing operations with scale parameters s1 and s2 should be a spatial smoothing operation of a similar form and with added values of scale parameters s1 + s2. (f) The assumption of a transitivity structure over temporal scales implies that the composition of two temporal smoothing operations from temporal scales τ1 to τ2 and from temporal scales τ2 to τ3 should be a similar type of temporal smoothing operation from temporal scales τ1 to τ3 (while without imposing an additive structure of the temporal scale parameters). (g) The assumption of non-enhancement of local extrema means that the spatial smoothing operation that determines the shape of the spatial receptive fields should obey the property that the smoothed intensity value L at a spatial maximum must not increase with increasing scale and that the intensity value at a spatial minimum must not decrease with increasing scale. (h) The assumption of non-creation of local extrema implies that the temporal smoothing operation that determines the temporal shape of the spatio-temporal receptive fields must not increase the number of local extrema in a purely temporal signal.
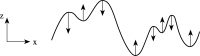
Illustration of the notion of non-enhancement of local extrema, which is a way to restrict the class of possible image operations by preventing new structures from being created from finer to coarser levels of scales. Non-enhancement of local extrema means that the value at a local maximum must not increase and that the value at a local minimum must not decrease with increasing scale s.
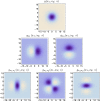
Illustrations of spatial receptive fields formed by the 2-D rotationally symmetric Gaussian kernel (for s = 16) and its partial derivatives up to order two. The resulting receptive fields are closed under translations, rotations and scaling transformations. This means that if an image is transformed in these ways, then it will always be possible to find some possibly other receptive field such that the receptive field responses of the original image and the transformed image can be perfectly matched.
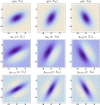
Illustrations of spatial receptive fields formed by affine Gaussian kernels and directional derivatives of these up to order two of, here visualized for three different covariance matrices Σ1, Σ2 and Σ3 that correspond to the major eigendirections θ1 = π/6, θ2 = π/3 and θ3 = 2π/3 of the covariance matrix and with directional derivatives computed in the corresponding orthogonal directions φ1, φ2 and φ3. The resulting family of receptive fields is closed under general affine transformations of the spatial domain, including translations, rotations, scaling transformations and perspective foreshortening. In this figure, however, only variabilities in the orientation of the filter are illustrated, thereby disregarding variabilities in both the size and the degree of elongation. This closedness property implies that receptive field responses computed from different views of a smooth local surface patch can be perfectly matched, if the transformation between the two views can be modelled as a local affine transformation. (Scale parameters s1 = 16 and s2 = 4 in the orthogonal eigendirections of the spatial covariance matrices Σi.)
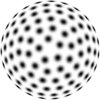
Illustration of the variability of zero-order affine Gaussian receptive fields for a uniform distribution on a hemisphere. In the most idealized version of the theory, one can think of all affine receptive fields with their directional derivatives in preferred directions aligned to the eigendirections of the covariance matrix Σ as being present at any position in the image domain. This variability makes it possible to perfectly match the first-order variability of receptive field responses under variations of the slant and tilt directions of a smooth surface patch.
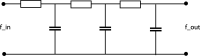
Illustration of the time-causal receptive field model in terms of an electric wiring diagram composed of a set of resistors and capacitors that emulate a series of first-order integrators coupled in cascade. In this model, the time-varying voltage fin represents the time varying input signal, whereas the time-varying voltage fout represents the time-varying output signal at a coarser temporal scale. From the theory for temporal scale-space kernels for one-dimensional signals (Lindeberg , ; Lindeberg and Fagerström [57]), it holds that the corresponding equivalent truncated exponential kernels are the only primitive temporal smoothing kernels that guarantee both temporal causality and non-creation of local extrema (or zero-crossings) with increasing temporal scale.
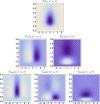
Illustrations of space-time separable receptive fieldsTxmtn(x,t;s,τ)=∂xmtn(g(x;s)h(t;τ)) up to order two, formed from by the composition of Gaussian kernels over the spatial domain x for spatial scale parameter s = 1 and a set of truncated exponential kernels coupled in cascade over the temporal domain t according to Equation (27), with a logarithmic distribution of the intermediate temporal scale levels that approximates the time-causal limit kernel in Equation (30) with the following parameters: τ = 1, K = 7, c = 2, v = 0. The corresponding family of spatio-temporal receptive fields is closed under spatial scaling transformations as well as under temporal scaling transformations for temporal scaling factors that are integer powers of the distribution parameter c of the temporal smoothing kernel. (Horizontal axis: space x. Vertical axis: time t.)
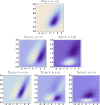
Illustrations of velocity-adapted spatio-temporal receptive fieldsTxmtn(x,t;s,τ,v)=∂xmtn(g(x−vt;s)h(t;τ)) up to order two, formed from the composition of Gaussian kernels over the spatial domain x for spatial scale parameter s = 1 and a set of truncated exponential kernels coupled in cascade over the temporal domain t according to Equation (27), with a logarithmic distribution of the intermediate temporal scale levels that approximates the time-causal limit kernel in Equation (30) with the following parameters: τ = 1, K = 7, c = 2, v = 1. In addition to spatial and temporal scaling transformations, the corresponding family of receptive fields is also closed under Galilean transformations. (Horizontal axis: space x. Vertical axis: time t.)
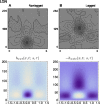
Spatio-temporal modelling of LGN neurons. Regarding space-time separable receptive fields in the lateral geniculate nucleus (LGN), there are two main types: For a “non-lagged cell”, the first temporal lobe is strongest, whereas for a “lagged cell”, the second temporal lobe is the strongest one. The top row shows examples of such neurons reported by DeAngelis et al. . In the bottom row, we have modelled these receptive fields by idealized spatio-temporal receptive fields of the form T(x,t;s,τ)=∂xm∂tn(g(;s)h(t;τ)) according to Equation (25), for m = 2 corresponding to a Laplacian of Gaussian over the spatial domain, and with the temporal smoothing function h(t; τ) expressed as a cascade of first-order integrators or equivalently truncated exponential kernels of the form (27) and using a logarithmic distribution of the intermediate temporal scale levels. Specifically, in the (left) we model a “non-lagged cell” by first-order temporal derivatives, whereas we model (right) a “lagged cell” using second-order temporal derivatives. Parameter values with σx=s and σt=τ: (a) hxxt: σx = 0.5 degrees, σt = 60 ms, c = 2. (b) hxxtt: σx = 0.6 degrees, σt = 140 ms, c = 2. (Horizontal dimension: space x. Vertical dimension: time t.) (The figures in the top row are reprinted with permission.)
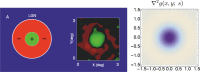
Spatial modelling of LGN neurons. (left) DeAngelis et al. report that LGN neurons have approximately circular center-surround responses over the spatial domain. (right) In terms of our idealized receptive field models, such a spatial dependency can be modelled by the Laplacian of the Gaussian ∇2g(x,y;s)=(x2+y2−2s)/(2πs3)exp(−(x2+y2)/2s), here with σs=s=0.6 in units of degrees of visual angle. (Left and middle figures reprinted with permission.)
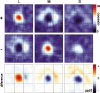
Receptive field responses of a spatio-chromatic double-opponent neuron according to Conway and Livingstone [22, Fig. 2, Page 10831]. Here, the colour channels L, M and S basically correspond to red, green and blue colour channels, respectively, from which corresponding red/green and yellow/blue colour-opponent channels can be computed from the difference between L to M and the difference between L+M to S, respectively.
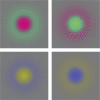
Modelling of double-opponent neurons using idealized spatio-chromatic receptive fields over the spatial domain. Here, we have applied the spatial Laplacian operator to positive and negative red/green and yellow/blue colour opponent channels, respectively. These receptive fields can be seen as idealized models of the spatial component of double-opponent spatio-chromatic receptive fields in the LGN.
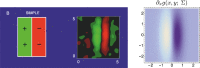
Computational modelling of a receptive field profile over the spatial domain in the primary visual cortex (V1) as reported by DeAngelis et al. , using affine Gaussian derivatives: (middle) Receptive field profile of a simple cell over image intensities as reconstructed from cell recordings, with positive weights represented as green and negative weights by red. (left) Stylized simplification of the receptive field shape. (right) Idealized model of the receptive field from a first-order directional derivative of an affine Gaussian kernel ∂xg(x,y; Σ)=∂xg(x,y; λx,λy) according to (21), here with σx=λx=0.5 and σy=λy=1.5 in units of degrees of visual angle, and with positive weights with respect to image intensities represented by white and negative values by violet. (Left and middle figures reprinted with permission.)
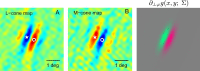
Modelling of double-opponent simple cells in the primary visual cortex (V1) in terms of affine Gaussian derivatives over colour-opponent channels, based on neurophysiological cell recordings by Johnson et al. : (left) Responses to L-cones corresponding to long wavelength red cones, with positive weights represented by red and negative weights by blue. (middle) Responses to M-cones corresponding to medium wavelength green cones, with positive weights represented by red and negative weights by blue. (right) Idealized model of the receptive field from a first-order directional derivative of an affine Gaussian kernel ∂⊥φg(x,y; Σ) according to (21) over a red-green colour-opponent channel for σ1=λ1=0.6 and σ2=λ2=0.2 in units of degrees of visual angle, α = 67 degrees and with positive weights for the red-green colour-opponent channel represented by red and negative values by green. (Left and middle figures: Copyright 2008 of Society for Neuroscience with permission.)
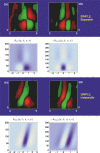
Modelling of space-time separable and inseparable simple cells in the primary visual cortex (V1) based on neural cell recordings reported by DeAngelis et al. . The idealized spatio-temporal receptive fields are of the form T(x,t;s,τ,v)=∂xm∂tn(g(x−vt;s)h(t;τ)) according to Equation (25), where v = 0 corresponds to space-time separable receptive fields and v ≠ 0 to inseparable receptive fields. The temporal smoothing function h(t; τ) is modelled as a set of first-order integrators/truncated exponential kernels of the form (27) coupled in cascade and using a logarithmic distribution of the intermediate temporal scale levels. (upper left) Separable receptive fields corresponding to first-order derivatives with respect to space and time. (upper right) Separable receptive fields corresponding to second-order derivatives with respect to space and first-order derivatives with respect to time. (lower left) Inseparable velocity-adapted receptive fields corresponding to second-order derivatives over space. (lower right) Inseparable velocity-adapted receptive fields corresponding to third-order derivatives over space. Parameter values with σx=s and σt=τ: (a) hxt: σx = 0.6 degrees, σt = 80 ms, c = 2. (b) hxxt: σx = 0.6 degrees, σt = 120 ms, c = 2. (c) hxx: σx = 0.7 degrees, σt = 70 ms, v = 0.007 degrees/ms, c = 2. (d) hxxx: σx = 0.5 degrees, σt = 100 ms, v = 0.004 degrees/ms, c = 2. (Horizontal axis: Space x in degrees of visual angle. Vertical axis: Time t in ms.) (The figures in the top and third rows reprinted with permission.)
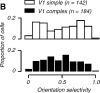
Measurements of the orientation selectivity of simple cells and complex cells in the primary visual cortex of the Macaque monkey as reported by Goris et al. . Interpreted with regard to the affine Gaussian derivative model for the receptive fields of simple cells (23), this large variability in orientation selectivity implies that we should consider covariance matrices Σ for a large range of eccentricities, as can be quantified by ratio between their eigenvalues λ1 and λ2. (The orientation selectivity of an affine Gaussian derivative kernel increases with the eccentricity.)
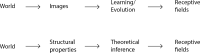
Two structurally different ways of deriving receptive field shapes for a vision system intended to infer properties of the world by either biological or artificial visual perception. (top row) A traditional model for learning receptive fields shapes consists of collecting real-world image data from the environment, and then applying learning algorithms possibly in combination with evolution over multiple generations of the organism that the vision system is a part of. (bottom row) With the normative theory for receptive fields presented in this paper, a short-cut is made in the sense that the derivation of receptive field shapes starts from structural properties of the world (corresponding to symmetry properties in theoretical physics) from which receptive field shapes are constrained by theoretical mathematical inference.
Similar articles
-
Lindeberg T. Lindeberg T. Front Comput Neurosci. 2023 Jun 15;17:1189949. doi: 10.3389/fncom.2023.1189949. eCollection 2023. Front Comput Neurosci. 2023. PMID: 37398936 Free PMC article.
-
A computational theory of visual receptive fields.
Lindeberg T. Lindeberg T. Biol Cybern. 2013 Dec;107(6):589-635. doi: 10.1007/s00422-013-0569-z. Epub 2013 Nov 7. Biol Cybern. 2013. PMID: 24197240 Free PMC article.
-
Invariance of visual operations at the level of receptive fields.
Lindeberg T. Lindeberg T. PLoS One. 2013 Jul 19;8(7):e66990. doi: 10.1371/journal.pone.0066990. Print 2013. PLoS One. 2013. PMID: 23894283 Free PMC article. Review.
-
Idealized computational models for auditory receptive fields.
Lindeberg T, Friberg A. Lindeberg T, et al. PLoS One. 2015 Mar 30;10(3):e0119032. doi: 10.1371/journal.pone.0119032. eCollection 2015. PLoS One. 2015. PMID: 25822973 Free PMC article.
-
Mapping the primate lateral geniculate nucleus: a review of experiments and methods.
Jeffries AM, Killian NJ, Pezaris JS. Jeffries AM, et al. J Physiol Paris. 2014 Feb;108(1):3-10. doi: 10.1016/j.jphysparis.2013.10.001. Epub 2013 Nov 21. J Physiol Paris. 2014. PMID: 24270042 Free PMC article. Review.
Cited by
-
Lindeberg T. Lindeberg T. Front Comput Neurosci. 2023 Jun 15;17:1189949. doi: 10.3389/fncom.2023.1189949. eCollection 2023. Front Comput Neurosci. 2023. PMID: 37398936 Free PMC article.
-
Wang J, Qian L, Wang S, Shi L, Wang Z. Wang J, et al. Animals (Basel). 2022 Apr 28;12(9):1143. doi: 10.3390/ani12091143. Animals (Basel). 2022. PMID: 35565569 Free PMC article.
-
Maximal Dependence Capturing as a Principle of Sensory Processing.
Raj R, Dahlen D, Duyck K, Yu CR. Raj R, et al. Front Comput Neurosci. 2022 Mar 25;16:857653. doi: 10.3389/fncom.2022.857653. eCollection 2022. Front Comput Neurosci. 2022. PMID: 35399919 Free PMC article.
-
Reconstructing Group Wavelet Transform From Feature Maps With a Reproducing Kernel Iteration.
Barbieri D. Barbieri D. Front Comput Neurosci. 2022 Mar 15;16:775241. doi: 10.3389/fncom.2022.775241. eCollection 2022. Front Comput Neurosci. 2022. PMID: 35370587 Free PMC article.
-
Bio-inspired contour extraction via EM-driven deformable and rotatable directivity-probing mask.
Wang JH, Huang RJ, Wang TY. Wang JH, et al. Sci Rep. 2022 Jul 19;12(1):12309. doi: 10.1038/s41598-022-16040-6. Sci Rep. 2022. PMID: 35853914 Free PMC article.
References
-
- Hubel D.H., Wiesel T.N. Oxford University Press; 2005. Brain and Visual Perception: The Story of a 25-Year Collaboration.
-
- DeAngelis G.C., Ohzawa I., Freeman R.D. Receptive field dynamics in the central visual pathways. Trends Neurosci. 1995;18(10):451–457. - PubMed
-
- DeAngelis G.C., Anzai A. A modern view of the classical receptive field: linear and non-linear spatio-temporal processing by V1 neurons. In: Chalupa L.M., Werner J.S., editors. The Visual Neurosciences. vol. 1. MIT Press; 2004. pp. 704–719.
LinkOut - more resources
Full Text Sources
Other Literature Sources