RiboNT: A Noise-Tolerant Predictor of Open Reading Frames from Ribosome-Protected Footprints - PubMed
- ️Fri Jan 01 2021
RiboNT: A Noise-Tolerant Predictor of Open Reading Frames from Ribosome-Protected Footprints
Bo Song et al. Life (Basel). 2021.
Abstract
Ribo-seq, also known as ribosome profiling, refers to the sequencing of ribosome-protected mRNA fragments (RPFs). This technique has greatly advanced our understanding of translation and facilitated the identification of novel open reading frames (ORFs) within untranslated regions or non-coding sequences as well as the identification of non-canonical start codons. However, the widespread application of Ribo-seq has been hindered because obtaining periodic RPFs requires a highly optimized protocol, which may be difficult to achieve, particularly in non-model organisms. Furthermore, the periodic RPFs are too short (28 nt) for accurate mapping to polyploid genomes, but longer RPFs are usually produced with a compromise in periodicity. Here we present RiboNT, a noise-tolerant ORF predictor that can utilize RPFs with poor periodicity. It evaluates RPF periodicity and automatically weighs the support from RPFs and codon usage before combining their contributions to identify translated ORFs. The results demonstrate the utility of RiboNT for identifying both long and small ORFs using RPFs with either good or poor periodicity. We implemented the pipeline on a dataset of RPFs with poor periodicity derived from membrane-bound polysomes of Arabidopsis thaliana seedlings and identified several small ORFs (sORFs) evolutionarily conserved in diverse plant species. RiboNT should greatly broaden the application of Ribo-seq by minimizing the requirement of RPF quality and allowing the use of longer RPFs, which is critical for organisms with complex genomes because these RPFs can be more accurately mapped to the position from which they were derived.
Keywords: ORFs; RPFs; Ribo-seq; periodicity; ribosome profiling; small ORFs.
Conflict of interest statement
The authors declare that they have no competing interests.
Figures
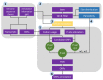
The RiboNT workflow consists six steps. Step 1: assemble transcripts according to the genome annotation information and extract candidate ORFs; step 2: examine the quality of RPFs and filter out low-quality RPFs; step 3: calculate the offsets to the start codon for RPFs in each size; step 4: balance the weight between RPFs and codon usages according to the periodicity of RPFs; step 5: identify translated ORFs from candidate ORFs by combing four student’s t-tests (RPF depth, frame 0 vs. 1, 2; codon usage, frame 0 vs. 1, 2); step 6: classify the predicted ORFs into different classes.
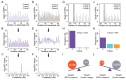
The strategy of RPFs filtering and weighing. (A) The distribution of high-quality RPFs on CDSs. (B) The depth distribution of RPFs shown in (A) is transformed into a wave function by connecting the vertices, with the coordinates on the CDSs converted into a timeline in second. (C) The resulting wave was subjected to a F-test implemented in “multitaper”, an R package. RPFs showing significance (p <= 0.01) at frequency of 0.33 Hz (the period recurs every 3 s) were retained. RPFs without periodicity (D–F) do not satisfy these criteria. The horizontal (gray) and vertical (red) dotted lines in (C) and (F) indicate the position of p = 0.01 and Frequency = 0.33 Hz, respectively. (G) The first RPFs shown in CDSs represent the mRNA fragments protected by ribosomes translating the start codon. Their offsets to the start codon were determined by the distance from its 5′ terminus to the translated P-sites. (H) RPFs predominantly located on one of these frames would result in lower overall entropy and will be weighted more (I) in the identification of ORFs. On the other hand, (J) RPFs with weak periodicities have higher overall entropy (K) and will be weighted less (L).
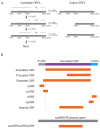
Searching and classification of ORFs. (A) Stepwise search for the longest ORF candidates with a priority to those start with AUG. (B) Classification of identified ORFs according to their positions relative to the annotated ORFs.
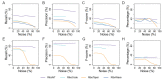
Performance of RiboNT, RiboCode and RiboTaper in detecting annotated ORFs. The recall (A), precision (B), F-score (C) and percent support from MS datasets (D) of annotated ORFs identified by RiboNT (purple), RiboCode (cyan) and RiboTaper (orange) in human (A–D) and yeast (E–H) datasets using RPFs with varying amount of noise (0–90%). The horizontal dotted lines in (D) and (H) indicate the percentage of MS-validated ORFs in the reference genome.
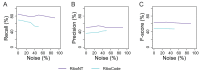
Performance of RiboNT and RiboCode in TIS identification. The recall (A), precision (B) and F-score (C) of TISs identified by RiboNT (purple) and RiboCode (cyan) in human using RPFs with different amounts of noise (0–90%).
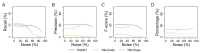
Performance of RiboNT, RiboCode and RiboTaper in sORF identification. The recall (A), precision (B), F-score (C) and support percentage of MS datasets (D) of sORFs identified by RiboNT (purple), RiboCode (cyan) and RiboTaper (orange) in the yeast genome using RPFs with varied amount of noise (0–90%). The horizontal dotted line in (D) indicates the percentage of MS-validated sORFs in the reference genome.
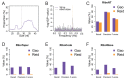
Performance of RiboNT, RiboCode, RiboTaper and RiboWave for the identification of annotated ORFs from a noisy dataset of human RPFs. (A) Distribution of RPFs sizes from the Reid et al. dataset with the vertical dotted line indicating the canonical 28 nt RPF size. (B) “Multitaper” test for periodicity of the Reid et al. RPFs (34 nt) indicates poor periodicity. The prediction of annotated ORFs from the Gao et al. (periodic) and Reid et al. (poor periodicity) datasets by (C) RiboNT, (D) RiboTaper, (E) RiboCode and (F) RiboWave.
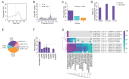
Implementation of RiboNT on a noisy RPF dataset derived from Arabidopsis membrane-bound polysomes. (A) RPF size distribution from Li et al. peaked at 32 nt with vertical dotted line indicating the canonical 28 nt RPF. (B) “Multitaper” test for periodicity from Li et al. (32 nt) indicates poor periodicity with high entropy (C). (D) RiboNT recovered the majority of the annotated ORFs in the A. thaliana genome with considerable precision, while RiboTaper did not. (E) RiboNT also identified several sORFs including 114 uORFs, 93 ouORFs, 245 dORFs, 232 odORFs and 13 ncsORFs. sORFs were also identified from transposable element and pseudogene transcripts. (F) The percentage of identified ORFs validated by the MS dataset for the varies sORF type; the error bars indicate standard deviation. The horizontal dotted line indicates the percentage of MS-validated ORFs in the reference genome. (G) The ncsORFs identified from this dataset can be categorized into three groups according to their sequence conservations across plant genomes. The ncsORFs in group 1 are specific to A. thaliana while those in group 2 are family conserved and those in group 3 are conserved from ferns to monocots and eudicots. The scale bar indicates sequence similarity.
Similar articles
-
Identification of short open reading frames in plant genomes.
Feng Y, Jiang M, Yu W, Zhou J. Feng Y, et al. Front Plant Sci. 2023 Feb 15;14:1094715. doi: 10.3389/fpls.2023.1094715. eCollection 2023. Front Plant Sci. 2023. PMID: 36875581 Free PMC article. Review.
-
Jiang M, Ning W, Wu S, Wang X, Zhu K, Li A, Li Y, Cheng S, Song B. Jiang M, et al. Brief Bioinform. 2022 Jul 18;23(4):bbac210. doi: 10.1093/bib/bbac210. Brief Bioinform. 2022. PMID: 35698834 Free PMC article.
-
Liu Q, Shvarts T, Sliz P, Gregory RI. Liu Q, et al. Nucleic Acids Res. 2020 Jul 2;48(W1):W218-W229. doi: 10.1093/nar/gkaa395. Nucleic Acids Res. 2020. PMID: 32427338 Free PMC article.
-
Super-resolution ribosome profiling reveals unannotated translation events in Arabidopsis.
Hsu PY, Calviello L, Wu HL, Li FW, Rothfels CJ, Ohler U, Benfey PN. Hsu PY, et al. Proc Natl Acad Sci U S A. 2016 Nov 8;113(45):E7126-E7135. doi: 10.1073/pnas.1614788113. Epub 2016 Oct 21. Proc Natl Acad Sci U S A. 2016. PMID: 27791167 Free PMC article.
-
Ribosomal profiling adds new coding sequences to the proteome.
Mumtaz MA, Couso JP. Mumtaz MA, et al. Biochem Soc Trans. 2015 Dec;43(6):1271-6. doi: 10.1042/BST20150170. Biochem Soc Trans. 2015. PMID: 26614672 Review.
Cited by
-
A review of Ribosome profiling and tools used in Ribo-seq data analysis.
Limbu MS, Xiong T, Wang S. Limbu MS, et al. Comput Struct Biotechnol J. 2024 Apr 22;23:1912-1918. doi: 10.1016/j.csbj.2024.04.051. eCollection 2024 Dec. Comput Struct Biotechnol J. 2024. PMID: 38721586 Free PMC article. Review.
-
Shining in the dark: the big world of small peptides in plants.
Feng YZ, Zhu QF, Xue J, Chen P, Yu Y. Feng YZ, et al. aBIOTECH. 2023 Apr 8;4(3):238-256. doi: 10.1007/s42994-023-00100-0. eCollection 2023 Sep. aBIOTECH. 2023. PMID: 37970469 Free PMC article. Review.
-
Identification of short open reading frames in plant genomes.
Feng Y, Jiang M, Yu W, Zhou J. Feng Y, et al. Front Plant Sci. 2023 Feb 15;14:1094715. doi: 10.3389/fpls.2023.1094715. eCollection 2023. Front Plant Sci. 2023. PMID: 36875581 Free PMC article. Review.
-
Small open reading frames in plant research: from prediction to functional characterization.
Ong SN, Tan BC, Al-Idrus A, Teo CH. Ong SN, et al. 3 Biotech. 2022 Mar;12(3):76. doi: 10.1007/s13205-022-03147-w. Epub 2022 Feb 24. 3 Biotech. 2022. PMID: 35251879 Free PMC article. Review.
-
Life: Computational Genomics Applications in Life Sciences.
Orlov YL, Anashkina AA. Orlov YL, et al. Life (Basel). 2021 Nov 9;11(11):1211. doi: 10.3390/life11111211. Life (Basel). 2021. PMID: 34833087 Free PMC article.
References
-
- Hayden C.A., Jorgensen R.A. Identification of novel conserved peptide uORF homology groups in Arabidopsis and rice reveals ancient eukaryotic origin of select groups and preferential association with transcription factor-encoding genes. BMC Biol. 2007;5:1–30. doi: 10.1186/1741-7007-5-32. - DOI - PMC - PubMed
Grants and funding
LinkOut - more resources
Full Text Sources
Research Materials