Fitting quantum machine learning potentials to experimental free energy data: predicting tautomer ratios in solution - PubMed
- ️Fri Jan 01 2021
. 2021 Jul 19;12(34):11364-11381.
doi: 10.1039/d1sc01185e. eCollection 2021 Sep 1.
Affiliations
- PMID: 34567495
- PMCID: PMC8409483
- DOI: 10.1039/d1sc01185e
Fitting quantum machine learning potentials to experimental free energy data: predicting tautomer ratios in solution
Marcus Wieder et al. Chem Sci. 2021.
Abstract
The computation of tautomer ratios of druglike molecules is enormously important in computer-aided drug discovery, as over a quarter of all approved drugs can populate multiple tautomeric species in solution. Unfortunately, accurate calculations of aqueous tautomer ratios-the degree to which these species must be penalized in order to correctly account for tautomers in modeling binding for computer-aided drug discovery-is surprisingly difficult. While quantum chemical approaches to computing aqueous tautomer ratios using continuum solvent models and rigid-rotor harmonic-oscillator thermochemistry are currently state of the art, these methods are still surprisingly inaccurate despite their enormous computational expense. Here, we show that a major source of this inaccuracy lies in the breakdown of the standard approach to accounting for quantum chemical thermochemistry using rigid rotor harmonic oscillator (RRHO) approximations, which are frustrated by the complex conformational landscape introduced by the migration of double bonds, creation of stereocenters, and introduction of multiple conformations separated by low energetic barriers induced by migration of a single proton. Using quantum machine learning (QML) methods that allow us to compute potential energies with quantum chemical accuracy at a fraction of the cost, we show how rigorous relative alchemical free energy calculations can be used to compute tautomer ratios in vacuum free from the limitations introduced by RRHO approximations. Furthermore, since the parameters of QML methods are tunable, we show how we can train these models to correct limitations in the underlying learned quantum chemical potential energy surface using free energies, enabling these methods to learn to generalize tautomer free energies across a broader range of predictions.
This journal is © The Royal Society of Chemistry.
Conflict of interest statement
There are no conflicts to declare.
Figures
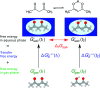
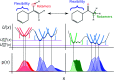
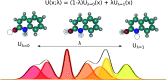
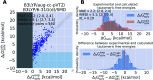
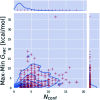
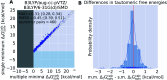
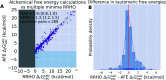
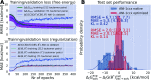
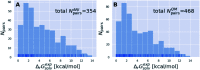
Similar articles
-
Using Experience Sampling Methodology to Capture Disclosure Opportunities for Autistic Adults.
Love AMA, Edwards C, Cai RY, Gibbs V. Love AMA, et al. Autism Adulthood. 2023 Dec 1;5(4):389-400. doi: 10.1089/aut.2022.0090. Epub 2023 Dec 12. Autism Adulthood. 2023. PMID: 38116059 Free PMC article.
-
Depressing time: Waiting, melancholia, and the psychoanalytic practice of care.
Salisbury L, Baraitser L. Salisbury L, et al. In: Kirtsoglou E, Simpson B, editors. The Time of Anthropology: Studies of Contemporary Chronopolitics. Abingdon: Routledge; 2020. Chapter 5. In: Kirtsoglou E, Simpson B, editors. The Time of Anthropology: Studies of Contemporary Chronopolitics. Abingdon: Routledge; 2020. Chapter 5. PMID: 36137063 Free Books & Documents. Review.
-
Ryan R, Hill S. Ryan R, et al. Cochrane Database Syst Rev. 2019 Oct 23;10(10):ED000141. doi: 10.1002/14651858.ED000141. Cochrane Database Syst Rev. 2019. PMID: 31643081 Free PMC article.
-
Enabling Systemic Identification and Functionality Profiling for Cdc42 Homeostatic Modulators.
Malasala S, Azimian F, Chen YH, Twiss JL, Boykin C, Akhtar SN, Lu Q. Malasala S, et al. bioRxiv [Preprint]. 2024 Jan 8:2024.01.05.574351. doi: 10.1101/2024.01.05.574351. bioRxiv. 2024. PMID: 38260445 Free PMC article. Updated. Preprint.
-
Triana L, Palacios Huatuco RM, Campilgio G, Liscano E. Triana L, et al. Aesthetic Plast Surg. 2024 Oct;48(20):4217-4227. doi: 10.1007/s00266-024-04260-2. Epub 2024 Aug 5. Aesthetic Plast Surg. 2024. PMID: 39103642 Review.
Cited by
-
QDπ: A Quantum Deep Potential Interaction Model for Drug Discovery.
Zeng J, Tao Y, Giese TJ, York DM. Zeng J, et al. J Chem Theory Comput. 2023 Feb 28;19(4):1261-1275. doi: 10.1021/acs.jctc.2c01172. Epub 2023 Jan 25. J Chem Theory Comput. 2023. PMID: 36696673 Free PMC article.
-
Machine-learned molecular mechanics force fields from large-scale quantum chemical data.
Takaba K, Friedman AJ, Cavender CE, Behara PK, Pulido I, Henry MM, MacDermott-Opeskin H, Iacovella CR, Nagle AM, Payne AM, Shirts MR, Mobley DL, Chodera JD, Wang Y. Takaba K, et al. Chem Sci. 2024 Jun 26;15(32):12861-12878. doi: 10.1039/d4sc00690a. eCollection 2024 Aug 14. Chem Sci. 2024. PMID: 39148808 Free PMC article.
-
Software Infrastructure for Next-Generation QM/MM-ΔMLP Force Fields.
Giese TJ, Zeng J, Lerew L, McCarthy E, Tao Y, Ekesan Ş, York DM. Giese TJ, et al. J Phys Chem B. 2024 Jul 4;128(26):6257-6271. doi: 10.1021/acs.jpcb.4c01466. Epub 2024 Jun 21. J Phys Chem B. 2024. PMID: 38905451 Free PMC article.
-
Célerse F, Wodrich MD, Vela S, Gallarati S, Fabregat R, Juraskova V, Corminboeuf C. Célerse F, et al. J Chem Inf Model. 2024 Feb 26;64(4):1201-1212. doi: 10.1021/acs.jcim.3c01953. Epub 2024 Feb 6. J Chem Inf Model. 2024. PMID: 38319296 Free PMC article.
References
-
- Kapetanović I., Drug Discovery and Development: Present and Future, IntechOpen, 2011
LinkOut - more resources
Full Text Sources