A Transcription Factor-Based Risk Model for Predicting the Prognosis of Prostate Cancer and Potential Therapeutic Drugs - PubMed
- ️Fri Jan 01 2021
A Transcription Factor-Based Risk Model for Predicting the Prognosis of Prostate Cancer and Potential Therapeutic Drugs
Ruixiang Luo et al. Evid Based Complement Alternat Med. 2021.
Abstract
Background: Prostate cancer (PC) is one of the most critical cancers affecting men's health worldwide. The development of many cancers involves dysregulation or mutations in key transcription factors. This study established a transcription factor-based risk model to predict the prognosis of PC and potential therapeutic drugs.
Materials and methods: In this study, RNA-sequencing data were downloaded and analyzed using The Cancer Genome Atlas dataset. A total of 145 genes related to the overall survival rate of PC patients were screened using the univariate Cox analysis. The Kdmist clustering method was used to classify prostate adenocarcinoma (PRAD), thereby determining the cluster related to the transcription factors. The support vector machine-recursive feature elimination method was used to identify genes related to the types of transcription factors and the key genes specifically upregulated or downregulated were screened. These genes were further analyzed using Lasso to establish a model. Gene Ontology (GO) and Kyoto Encyclopedia of Genes and Genomes (KEGG) were used for the functional analysis. The TIMER algorithm was used to quantify the abundance of immune cells in PRAD samples. The chemotherapy response of each GBM patient was predicted based on the public pharmacogenomic database, Genomics of Drug Sensitivity in Cancer (GDSC, http://www.cancerrxgene.org). The R package "pRRophetic" was applied to drug sensitivity (IC50) value prediction.
Results: We screened 10 genes related to prognosis, including eight low-risk genes and two high-risk genes. The receiver operating characteristic (ROC) curve was 0.946. Patients in the high-risk score group had a poorer prognosis than those in the low-risk score group. The average area under the curve value of the model at different times was higher than 0.8. The risk score was an independent prognostic factor. Compared with the low-risk score group, early growth response-1 (EGR1), CACNA2D1, AC005831.1, SLC52A3, TMEM79, IL20RA, CRACR2A, and FAM189A2 expressions in the high-risk score group were decreased, while AC012181.1 and TRAPPC8 expressions were increased. GO and KEGG analyses showed that prognosis was related to various cancer signaling pathways. The proportion of B_cell, T_cell_CD4, and macrophages in the high-risk score group was significantly higher than that in the low-risk score group. A total of 25 classic immune checkpoint genes were screened out to express abnormally high-risk scores, and there were significant differences. Thirty mutant genes were identified; in the high- and low-risk score groups, SPOP, TP53, and TTN had the highest mutation frequency, and their mutations were mainly missense mutations. A total of 36 potential drug candidates for the treatment of PC were screened and identified.
Conclusions: Ten genes of both high-and low-risk scores were associated with the prognosis of PC. PC prognosis may be related to immune disorders. SPOP, TP53, and TTN may be potential targets for the prognosis of PC.
Copyright © 2021 Ruixiang Luo et al.
Conflict of interest statement
The authors declare that there are no conflicts of interest regarding the publication of this paper.
Figures
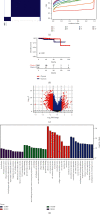
PRAD clustering and analysis. (a) Kdmist clustering. (b) Survival analysis. p < 0.05 indicated that there was a significant difference in the survival rate between the two groups. (c) Volcano map. (d) Functional analysis. PRAD, prostate adenocarcinoma.
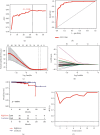
Screening of genes closely related to the prognosis of PC. (a) A different analysis of the two types of transcription factors. (b) ROC curve. (c) and (d) Lasso analysis. (e) Kaplan-Meier analysis. p < 0.05 indicated that there was a significant difference in survival rate between the two groups. (f) AUC value. PC, prostate cancer; ROC, receiver operating characteristic; AUC, area under the curve.
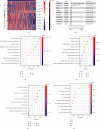
Correlation analysis of genes closely related to the prognosis of PC with risk score model. (a) Heat map. (b) Univariate factor analysis. (c) Analysis of related gene enrichment function.
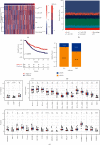
The correlation between risk score model and immune cells. (a) Heat map. (b) The proportion of cells in each sample. (c) Survival analysis. p < 0.05 indicated that there was a significant difference in the survival rate between the two groups. (d) The relative percentage of CR/PR and SD/PD in the high- and low-risk score. (e) Analysis of immune checkpoints at different levels. ∗p < 0.05. ∗∗p < 0.01. ∗∗∗p < 0.001. ∗∗∗∗p < 0.0001. CR, complete response; PR, partial response; PD; SD.
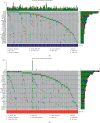
The waterfall chart showed the top 30 mutant genes. (a) Low-risk score group. (b) High-risk score group.
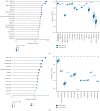
Drug candidates for the treatment of PC. (a) CTRP. (b) PRISM. ∗p < 0.05. ∗∗p < 0.01. ∗∗∗p < 0.001. ∗∗∗∗p < 0.0001. PC, prostate cancer.
Similar articles
-
A Novel Prognostic Signature of Transcription Factors for the Prediction in Patients With GBM.
Cheng Q, Huang C, Cao H, Lin J, Gong X, Li J, Chen Y, Tian Z, Fang Z, Huang J. Cheng Q, et al. Front Genet. 2019 Oct 1;10:906. doi: 10.3389/fgene.2019.00906. eCollection 2019. Front Genet. 2019. PMID: 31632439 Free PMC article.
-
Construction and Validation of a Prognostic Model Based on Mitochondrial Genes in Prostate Cancer.
Wang D, Pan H, Cheng S, Huang Z, Shi Z, Deng H, Yang J, Jin C, Dai J. Wang D, et al. Horm Metab Res. 2024 Nov;56(11):807-817. doi: 10.1055/a-2330-3696. Epub 2024 Jun 13. Horm Metab Res. 2024. PMID: 38870985
-
Construction of a Prognostic Immune-Related LncRNA Risk Model for Lung Adenocarcinoma.
Li Y, Shen R, Wang A, Zhao J, Zhou J, Zhang W, Zhang R, Zhu J, Liu Z, Huang JA. Li Y, et al. Front Cell Dev Biol. 2021 Mar 18;9:648806. doi: 10.3389/fcell.2021.648806. eCollection 2021. Front Cell Dev Biol. 2021. PMID: 33869203 Free PMC article.
-
Huang W, Li G, Wang Z, Zhou L, Yin X, Yang T, Wang P, Teng X, Feng Y, Yu H. Huang W, et al. Front Oncol. 2021 Feb 17;10:567931. doi: 10.3389/fonc.2020.567931. eCollection 2020. Front Oncol. 2021. PMID: 33680913 Free PMC article.
-
Classification of Lung Adenocarcinoma Based on Immune Checkpoint and Screening of Related Genes.
Zhou T, Yang P, Tang S, Zhu Z, Li X, Yang Z, Wu R, Tian X, Li L. Zhou T, et al. J Oncol. 2021 Jul 27;2021:5512325. doi: 10.1155/2021/5512325. eCollection 2021. J Oncol. 2021. PMID: 34367284 Free PMC article.
Cited by
-
Wang Y, Jin Q, Zhang S, Wang Y. Wang Y, et al. Eur J Med Res. 2023 Nov 7;28(1):490. doi: 10.1186/s40001-023-01388-w. Eur J Med Res. 2023. PMID: 37936239 Free PMC article.
-
Endocrine Disrupting Chemicals Influence Hub Genes Associated with Aggressive Prostate Cancer.
Alwadi D, Felty Q, Yoo C, Roy D, Deoraj A. Alwadi D, et al. Int J Mol Sci. 2023 Feb 6;24(4):3191. doi: 10.3390/ijms24043191. Int J Mol Sci. 2023. PMID: 36834602 Free PMC article.
-
PRLR and CACNA2D1 Impact the Prognosis of Breast Cancer by Regulating Tumor Immunity.
Liang J, Deng Y, Zhang Y, Wu B, Zhou J. Liang J, et al. J Pers Med. 2022 Dec 19;12(12):2086. doi: 10.3390/jpm12122086. J Pers Med. 2022. PMID: 36556307 Free PMC article.
References
LinkOut - more resources
Full Text Sources
Research Materials
Miscellaneous