Prognostic Value and Correlation With Tumor Immune Infiltration of a Novel Metabolism-Related Gene Signature in Pancreatic Cancer - PubMed
- ️Sat Jan 01 2022
Prognostic Value and Correlation With Tumor Immune Infiltration of a Novel Metabolism-Related Gene Signature in Pancreatic Cancer
Hui Chen et al. Front Oncol. 2022.
Abstract
Background: Energy metabolism has been considered as one of the novel features of neoplasms. This study aimed to establish the prognostic signature for pancreatic cancer (PC) based on metabolism-related genes (MRGs).
Methods: We obtained MRGs from the Molecular Signatures Database (MSigDB) and gene sequence data in the Cancer Genome Atlas (TCGA) databases. Then, differentially expressed MRGs (DE-MRGs) were identified utilizing the R software. We built the prognostic model via multivariate Cox regression. Moreover, external validation of the prognostic signature was also performed. Nomogram was created to predict the overall survival (OS). Next, this study analyzed the prognostic value, clinical relationship, and metabolism-related signaling pathways of the prognostic signature. The role in tumor infiltration was further evaluated. Eventually, the expression level of the three MRGs along with the function of NT5E was validated.
Results: Twenty-two MRGs were chosen, eight of which were identified to be most significantly correlated with the prognosis of PC. Meanwhile, a 3-MRG prognostic signature was established, and we verified this prognostic model in two separate external cohorts. What is more, the nomogram was used to predict 1-/2-/3-year OS of PC patients. In addition, the immune cell infiltration and expression of immune checkpoint were significantly influenced by the risk score. Finally, three MRGs were highly expressed in PC cell lines, and NT5E was associated with the proliferation and migration ability of PC.
Conclusion: To sum up, the study established and validated a 3-MRG prognostic signature for PC, and the signature could be utilized to predict the prognosis and assist the individualized clinical management of patients with PC.
Keywords: immunotherapy; metabolism-related genes; pancreatic cancer; prognostic signature; tumor immune microenvironment.
Copyright © 2022 Chen, Zu, Zeng, Chen, Wei, Liu, Li, Zhou, Wang, Tan and Tan.
Conflict of interest statement
The authors declare that the research was conducted in the absence of any commercial or financial relationships that could be construed as a potential conflict of interest.
Figures
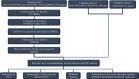
The flowchart for analyzing MRGs-prognostic signature.

DE-MRGs in PC. (A, B) MRGs in the TCGA database are shown in the Venn diagram and volcano plot. (C) Common MRGs in the GSE62452 dataset and TCGA database are shown in the Venn diagram. (D) Common MRGs are shown in the heatmap.
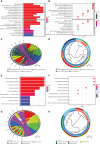
Enrichment analysis. (A, B) The bar and bubble charts of GO terms are enriched in BP and MF. (C, D) The chord with cluster plots shows the top 5 GO terms and the corresponding enriched genes. (E, F) The bar and bubble plots of KEGG enriched terms. (G, H) The chord with cluster maps shows the top 3 KEGG terms and the corresponding enriched genes. The false discovery rate (FDR) <0.05 of all terms was significant.
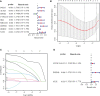
Establishing MRGs prognostic signature. (A) Recognition of the outcome-related MRGs in PC via univariate Cox regression analysis. p < 0.01 were statistically meaningful. (B) The tuning parameter (lambda) is determined at the vertical line. (C) LASSO coefficient profiles of the 5-MRGs according to the tuning parameter. (D) The 3-MRG prognostic signature was built via multivariate Cox analysis.
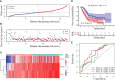
Risk score analysis of the 3-MRG prognostic model in the training cohort. (A–D) The risk curve, survival status of PC patients, heatmap of the 3-MRG expression, and survival curves between lower and higher-risk groups are shown. (E) Time-independent ROC curves of the risk score for predicting the OS in the training cohort.
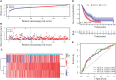
Risk score analysis of 3-MRG prognostic signature in the validation cohort 1. (A–D) The risk score, survival status of PC patients, heatmap of the 3-MRG expression, and survival curves between low and high-risk groups are shown. (E) Time-independent ROC analysis of risk score for predicting the OS in the ICGC cohort (PACA-AU and PACA-CA).
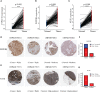
Comparing the 3-MRG mRNA levels in PC and normal pancreas tissues. (A–C) CYP2C18, INPP4B, and NT5E. Verifying the 3-MRG expression in PC with normal tissues on the HPA database. Information about CYP2C18 was not available. (D–G) INPP4B and NT5E.
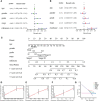
Independent prognostic value of risk score. (A) Univariate analysis. (B) Multivariate analysis. (C) Nomogram based on the signature for PC patients at 1/2/3-year. (D–F) Calibration plots of the nomogram at 1, 2, and 3 years. (G) DCA of this prognostic signature.
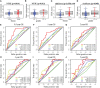
Association between risk score and MRGs expression with clinical features of PC. (A, B) NT5E. (C, D) Risk score. (E–J) The ROC curve analyses of the prognostic variables in the TCGA cohort of 0.5, 1, 2, 3, 4, and 5 years, respectively.
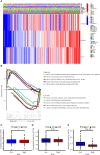
(A) The heatmap showed the 3-MRG expression in the prognostic signature based on different risk, clinicopathological characteristics, and survival status in the TCGA cohort. (B) GSEA revealed the top 5 up- and downregulated metabolism-related signaling pathways enriched in low- and high-risk groups. (C–E) The IC50 showed the chemosensitivity of cisplatin, gemcitabine, and paclitaxel in the low- and high-risk groups.
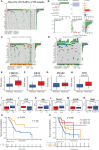
(A, B) Waterfall plot for the TCGA patients. (C, D) Waterfall plot for the patients in the high- and low-risk group. (E–H) Relationship between genes (CDKN2A, KRAS, SMAD4, and TP53) status and risk score. (I–N) The association of clinical characteristics and risk scores with TMB. (O, P) Kaplan–Meier survival analysis of patients with different TMB with/or different risks.
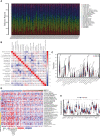
Assessment of tumor-infiltrating cells and immunotherapy value. (A) The bar chart reveals the percentage of 22 infiltrating immune cells from the TCGA cohort. (B) The correlation matrix shows correlations between infiltrating immune cells in tumor samples. (C, D) Violin plot and heatmap present the differentially infiltrated immune cells in lower- and higher-risk groups. (E) The differential expression of immune checkpoints between low- and high-risk groups.
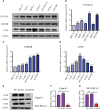
Validation of the 3-MRGs levels via WB and qRT-PCR. (A) The protein levels of the three MRGs in six PC cell lines and pancreatic epithelial cells. (B–D) The mRNA expression of the three MRGs in six PC cell lines and pancreatic epithelial cells. (E–G) Confirmation of NT5E knockdown efficiency by WB. *p < 0.05, **p < 0.01, ***p < 0.001, ns, not significant.
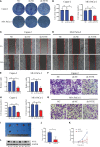
Validation of the function of NT5E via colony formation assay, wound-healing assay, Transwell assay, and animal experiments. (A, B) Colony formation assays. (C–E) Wound-healing assays. (F–H) Transwell assays. (I–K) Animal experiments. (L) Confirmation of NT5E knockdown efficiency in vivo by WB. N, negatively control; K, NT5E knockdown. *p < 0.05, **p < 0.01, ***p < 0.001, ns, not significant.
Similar articles
-
Wang Z, Embaye KS, Yang Q, Qin L, Zhang C, Liu L, Zhan X, Zhang F, Wang X, Qin S. Wang Z, et al. Cancer Cell Int. 2021 Apr 15;21(1):219. doi: 10.1186/s12935-021-01915-x. Cancer Cell Int. 2021. PMID: 33858449 Free PMC article.
-
Shen Y, Huang Q, Zhang Y, Hsueh CY, Zhou L. Shen Y, et al. Cancer Cell Int. 2022 Jul 8;22(1):226. doi: 10.1186/s12935-022-02647-2. Cancer Cell Int. 2022. PMID: 35804447 Free PMC article.
-
Jiang P, Sun W, Shen N, Huang X, Fu S. Jiang P, et al. BMC Cancer. 2020 Sep 7;20(1):864. doi: 10.1186/s12885-020-07345-8. BMC Cancer. 2020. PMID: 32894095 Free PMC article.
-
Zhao H, Chen Y, Shen P, Gong L. Zhao H, et al. Math Biosci Eng. 2021 Sep 15;18(6):8045-8063. doi: 10.3934/mbe.2021399. Math Biosci Eng. 2021. PMID: 34814288
-
Xu X, Wang J. Xu X, et al. Front Endocrinol (Lausanne). 2023 Mar 24;14:1153562. doi: 10.3389/fendo.2023.1153562. eCollection 2023. Front Endocrinol (Lausanne). 2023. PMID: 37033261 Free PMC article.
Cited by
-
Fang K, Tang DS, Yan CS, Ma J, Cheng L, Li Y, Wang G. Fang K, et al. Front Pharmacol. 2022 May 18;13:862502. doi: 10.3389/fphar.2022.862502. eCollection 2022. Front Pharmacol. 2022. PMID: 35662734 Free PMC article.
-
Chen G, Liu Y, Su D, Qiu J, Long J, Zhao F, Tao J, Yang G, Huang H, Xiao J, Zhang T, Zhao Y. Chen G, et al. Cell Oncol (Dordr). 2023 Dec;46(6):1691-1708. doi: 10.1007/s13402-023-00836-3. Epub 2023 Jul 11. Cell Oncol (Dordr). 2023. PMID: 37434012
-
Liu W, Qu C, Wang X. Liu W, et al. Oncol Res. 2023 Jun 27;31(4):543-567. doi: 10.32604/or.2023.029563. eCollection 2023. Oncol Res. 2023. PMID: 37415739 Free PMC article.
-
A glycosylation-related signature predicts survival in pancreatic cancer.
Hu H, He B, He M, Tao H, Li B. Hu H, et al. Aging (Albany NY). 2023 Nov 30;15(23):13710-13737. doi: 10.18632/aging.205258. Epub 2023 Nov 30. Aging (Albany NY). 2023. PMID: 38048216 Free PMC article.
-
INPP4B promotes PDAC aggressiveness via PIKfyve and TRPML-1-mediated lysosomal exocytosis.
Saffi GT, To L, Kleine N, Melo CMP, Chen K, Genc G, Lee KCD, Chow JT, Jang GH, Gallinger S, Botelho RJ, Salmena L. Saffi GT, et al. J Cell Biol. 2024 Nov 4;223(11):e202401012. doi: 10.1083/jcb.202401012. Epub 2024 Aug 9. J Cell Biol. 2024. PMID: 39120584 Free PMC article.
References
LinkOut - more resources
Full Text Sources
Research Materials
Miscellaneous