Multi-Omics Strategies for Investigating the Microbiome in Toxicology Research - PubMed
- ️Sat Jan 01 2022
Review
Multi-Omics Strategies for Investigating the Microbiome in Toxicology Research
Ethan W Morgan et al. Toxicol Sci. 2022.
Abstract
Microbial communities on and within the host contact environmental pollutants, toxic compounds, and other xenobiotic compounds. These communities of bacteria, fungi, viruses, and archaea possess diverse metabolic potential to catabolize compounds and produce new metabolites. Microbes alter chemical disposition thus making the microbiome a natural subject of interest for toxicology. Sequencing and metabolomics technologies permit the study of microbiomes altered by acute or long-term exposure to xenobiotics. These investigations have already contributed to and are helping to re-interpret traditional understandings of toxicology. The purpose of this review is to provide a survey of the current methods used to characterize microbes within the context of toxicology. This will include discussion of commonly used techniques for conducting omic-based experiments, their respective strengths and deficiencies, and how forward-looking techniques may address present shortcomings. Finally, a perspective will be provided regarding common assumptions that currently impede microbiome studies from producing causal explanations of toxicologic mechanisms.
Keywords: gut microbiome; metabolism; metabolomics; microbiome.
© The Author(s) 2022. Published by Oxford University Press on behalf of the Society of Toxicology. All rights reserved. For permissions, please e-mail: journals.permissions@oup.com.
Figures
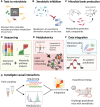
A, Exposure to xenobiotics may have a toxic effect on members of the microbiota, altering the community composition and affecting the host. B, Microbial metabolism of xenobiotics may mitigate the host’s response to the compound. This may provide protection to the host against toxicants or reduce the effectiveness of pharmaceutical treatments. C, Members of the microbiota may obscure the cause of host toxicity by generating toxins from xenobiotic compounds. D, 16S rRNA gene sequencing, shotgun metagenomics, and metatranscriptomics provide detailed profiles of the taxonomic composition and genetics of the gut microbiota. E, Metabolite profiling with LC-MS/MS is used to identify the metabolic contributions of different organisms to the microbiome and identify relationships between detected compounds. F, Statistics methods simplify datasets with hundreds or thousands of features to identify similarities between samples and predict the causes of feature variability. G, Integrating sequencing and metabolomics techniques can provide a means to generate testable hypotheses that can be assessed using gnotobiotic mice and synthetic communities. Created with BioRender.com.
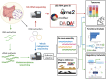
Sequencing-based analysis of the microbiome begins with the extraction of nucleic acids, library preparation, and high-throughput sequencing. Sequencing data is processed using bioinformatics software or pipelines based on the sequencing technique. Popular and user-friendly software for taxonomic identification using the 16S rRNA gene include QIIME2, DADA2, and mothur. Whole metagenome sequencing, also called shotgun metagenomics, and metatranscript sequencing data is often processed using complete software pipelines like HUMAnN2 or SqueezeMeta. All three sequencing techniques provide taxonomic profiling of the microbiome, but shotgun metagenomics and metatranscriptomics also provide profiles of functional potential or expressed genes. Created with BioRender.com.
Similar articles
-
Teffera M, Veith AC, Ronnekleiv-Kelly S, Bradfield CA, Nikodemova M, Tussing-Humphreys L, Malecki K. Teffera M, et al. Environ Int. 2024 Aug;190:108805. doi: 10.1016/j.envint.2024.108805. Epub 2024 Jun 10. Environ Int. 2024. PMID: 38901183 Review.
-
Chemical transformation of xenobiotics by the human gut microbiota.
Koppel N, Maini Rekdal V, Balskus EP. Koppel N, et al. Science. 2017 Jun 23;356(6344):eaag2770. doi: 10.1126/science.aag2770. Science. 2017. PMID: 28642381 Free PMC article. Review.
-
Metaproteomic and Metabolomic Approaches for Characterizing the Gut Microbiome.
Peters DL, Wang W, Zhang X, Ning Z, Mayne J, Figeys D. Peters DL, et al. Proteomics. 2019 Aug;19(16):e1800363. doi: 10.1002/pmic.201800363. Epub 2019 Jul 31. Proteomics. 2019. PMID: 31321880 Review.
-
Challenges and Perspective in Integrated Multi-Omics in Gut Microbiota Studies.
Daliri EB, Ofosu FK, Chelliah R, Lee BH, Oh DH. Daliri EB, et al. Biomolecules. 2021 Feb 17;11(2):300. doi: 10.3390/biom11020300. Biomolecules. 2021. PMID: 33671370 Free PMC article. Review.
-
Ruiz-Perez D, Gimon I, Sazal M, Mathee K, Narasimhan G. Ruiz-Perez D, et al. mSystems. 2024 Oct 22;9(10):e0130323. doi: 10.1128/msystems.01303-23. Epub 2024 Sep 6. mSystems. 2024. PMID: 39240096 Free PMC article.
Cited by
-
Bouyahya A, El Omari N, Bakha M, Aanniz T, El Menyiy N, El Hachlafi N, El Baaboua A, El-Shazly M, Alshahrani MM, Al Awadh AA, Lee LH, Benali T, Mubarak MS. Bouyahya A, et al. Pharmaceuticals (Basel). 2022 Oct 8;15(10):1235. doi: 10.3390/ph15101235. Pharmaceuticals (Basel). 2022. PMID: 36297347 Free PMC article. Review.
-
Genitourinary syndrome of menopause and intestinal microbiota.
Pavlovska O, Savelyeva O, Pavlovska K. Pavlovska O, et al. Prz Menopauzalny. 2023 Dec;22(4):213-219. doi: 10.5114/pm.2023.133828. Epub 2023 Dec 18. Prz Menopauzalny. 2023. PMID: 38239403 Free PMC article.
-
Perdew GH, Esser C, Snyder M, Sherr DH, van den Bogaard EH, McGovern K, Fernández-Salguero PM, Coumoul X, Patterson AD. Perdew GH, et al. Int J Mol Sci. 2023 Mar 14;24(6):5550. doi: 10.3390/ijms24065550. Int J Mol Sci. 2023. PMID: 36982624 Free PMC article.
-
Potential roles of gut microbes in biotransformation of natural products: An overview.
Zhao Y, Zhong X, Yan J, Sun C, Zhao X, Wang X. Zhao Y, et al. Front Microbiol. 2022 Sep 29;13:956378. doi: 10.3389/fmicb.2022.956378. eCollection 2022. Front Microbiol. 2022. PMID: 36246222 Free PMC article. Review.
-
Metabolome patterns identify active dechlorination in bioaugmentation consortium SDC-9™.
May AL, Xie Y, Kara Murdoch F, Michalsen MM, Löffler FE, Campagna SR. May AL, et al. Front Microbiol. 2022 Oct 25;13:981994. doi: 10.3389/fmicb.2022.981994. eCollection 2022. Front Microbiol. 2022. PMID: 36386687 Free PMC article.
References
-
- Aitchison J. (1982). The statistical analysis of compositional data. J. R. Stat. Soc. Series B Stat. Methodol. 44, 139–160.
-
- Aitchison J., Barceló-Vidal C., Martín-Fernández J. A., Pawlowsky-Glahn V. (2000). Logratio analysis and compositional distance. Math. Geol. 32, 271–275.
Publication types
MeSH terms
Substances
LinkOut - more resources
Full Text Sources