Multi-state MRAM cells for hardware neuromorphic computing - PubMed
- ️Sat Jan 01 2022
Multi-state MRAM cells for hardware neuromorphic computing
Piotr Rzeszut et al. Sci Rep. 2022.
Abstract
Magnetic tunnel junctions (MTJ) have been successfully applied in various sensing application and digital information storage technologies. Currently, a number of new potential applications of MTJs are being actively studied, including high-frequency electronics, energy harvesting or random number generators. Recently, MTJs have been also proposed in designs of new platforms for unconventional or bio-inspired computing. In the current work, we present a complete hardware implementation design of a neural computing device that incorporates serially connected MTJs forming a multi-state memory cell can be used in a hardware implementation of a neural computing device. The main purpose of the multi-cell is the formation of quantized weights in the network, which can be programmed using the proposed electronic circuit. Multi-cells are connected to a CMOS-based summing amplifier and a sigmoid function generator, forming an artificial neuron. The operation of the designed network is tested using a recognition of hand-written digits in 20 [Formula: see text] 20 pixels matrix and shows detection ratio comparable to the software algorithm, using weights stored in a multi-cell consisting of four MTJs or more. Moreover, the presented solution has better energy efficiency in terms of energy consumed per single image processing, as compared to a similar design.
© 2022. The Author(s).
Conflict of interest statement
The authors declare no competing interests.
Figures
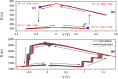
(a) Experimental R(V) dependence (solid points) and the model consisting of four lines and two critical points (stars) presenting a single MTJ behaviour. (b) A representative simulation result of three serially-connected MTJs (solid line) together with a series of example measurements (gray-scale lines) of a two-bit multicell. Parameters of a single MTJ were used to model the multi-cell characteristics. Arrows indicate voltage sweep direction.
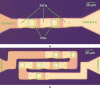
Microimage of the experimental setup: (a) three and (b) seven serially connected MTJs (dots) with vias (squares) marked.
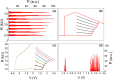
Simulation and experimental results for seven serially connected MTJs with a given parameter spread. (a) Spread of readout resistances for the simulation. (b) Representative write-read-erase curves. Red line represents full write-read-erase cycle, while black ones represent write-read cycles while programming subsequent values. (c) Experimental data for seven serially connected MTJs. MTJs in this case come from another batch, characterized by higher RA product of around 20Ømegaμm2, which results in approximately three times higher resistance of the multicell and slightly higher switching voltages. (d) Spread of all write voltages for the simulation.
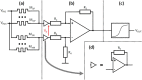
The proposed neuron design with multi-cells. The circuit consists of (a) a set of memristors serving as a quantized weight, (b) a differential amplifier with voltage followers (d) at input and (c) a sigmoid function block.
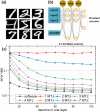
Simulated neural network based on multi-bit MRAM cells. Handwritten digits from MNIST database (a) are recognized by a standard neural network with architecture shown in (b), where black lines represent network weights and yellow circles represent individual neurons. After training, weights calculated by software are replaced by discretized values corresponding to 1–7 serial MTJs MRAM cells, which affects the network performance (c).
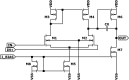
Operational amplifier circuit used in the design.
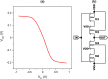
(a) A transfer function of a sigmoid-generating inverter implemented by (b) the proposed inverter circuit.
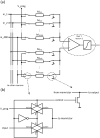
(a) Schematic of connection of programming switches and (b) the design of the programming switches.
Similar articles
-
Zhang X, Lu J, Wang Z, Wang R, Wei J, Shi T, Dou C, Wu Z, Zhu J, Shang D, Xing G, Chan M, Liu Q, Liu M. Zhang X, et al. Sci Bull (Beijing). 2021 Aug 30;66(16):1624-1633. doi: 10.1016/j.scib.2021.04.014. Epub 2021 Apr 17. Sci Bull (Beijing). 2021. PMID: 36654296
-
Design Space Exploration of Hardware Spiking Neurons for Embedded Artificial Intelligence.
Abderrahmane N, Lemaire E, Miramond B. Abderrahmane N, et al. Neural Netw. 2020 Jan;121:366-386. doi: 10.1016/j.neunet.2019.09.024. Epub 2019 Sep 26. Neural Netw. 2020. PMID: 31593842
-
A Probabilistic Synapse With Strained MTJs for Spiking Neural Networks.
Pagliarini SN, Bhuin S, Isgenc MM, Biswas AK, Pileggi L. Pagliarini SN, et al. IEEE Trans Neural Netw Learn Syst. 2020 Apr;31(4):1113-1123. doi: 10.1109/TNNLS.2019.2917819. Epub 2019 Jun 18. IEEE Trans Neural Netw Learn Syst. 2020. PMID: 31226090
-
Advancements in Algorithms and Neuromorphic Hardware for Spiking Neural Networks.
Javanshir A, Nguyen TT, Mahmud MAP, Kouzani AZ. Javanshir A, et al. Neural Comput. 2022 May 19;34(6):1289-1328. doi: 10.1162/neco_a_01499. Neural Comput. 2022. PMID: 35534005 Review.
-
Towards spike-based machine intelligence with neuromorphic computing.
Roy K, Jaiswal A, Panda P. Roy K, et al. Nature. 2019 Nov;575(7784):607-617. doi: 10.1038/s41586-019-1677-2. Epub 2019 Nov 27. Nature. 2019. PMID: 31776490 Review.
Cited by
-
Li B, Xia F, Du B, Zhang S, Xu L, Su Q, Zhang D, Yang J. Li B, et al. Adv Sci (Weinh). 2024 Jun;11(23):e2310263. doi: 10.1002/advs.202310263. Epub 2024 Apr 22. Adv Sci (Weinh). 2024. PMID: 38647431 Free PMC article. Review.
-
Kim K, Song MS, Hwang H, Hwang S, Kim H. Kim K, et al. Front Neurosci. 2024 Apr 10;18:1279708. doi: 10.3389/fnins.2024.1279708. eCollection 2024. Front Neurosci. 2024. PMID: 38660225 Free PMC article. Review.
References
-
- Fu, J., Zheng, H. & Mei, T. Look closer to see better: Recurrent attention convolutional neural network for fine-grained image recognition. In Proceedings of the IEEE conference on computer vision and pattern recognition, 4438–4446 (2017).
-
- Venayagamoorthy, G. K., Moonasar, V. & Sandrasegaran, K. Voice recognition using neural networks. In Proceedings of the 1998 South African Symposium on Communications and Signal Processing-COMSIG’98 (Cat. No. 98EX214), 29–32 (IEEE, 1998).
-
- Zhang Y, Li S, Guo H. A type of biased consensus-based distributed neural network for path planning. Nonlinear Dyn. 2017;89:1803–1815. doi: 10.1007/s11071-017-3553-7. - DOI
-
- Muralitharan K, Sakthivel R, Vishnuvarthan R. Neural network based optimization approach for energy demand prediction in smart grid. Neurocomputing. 2018;273:199–208. doi: 10.1016/j.neucom.2017.08.017. - DOI
-
- Abhishek K, Singh M, Ghosh S, Anand A. Weather forecasting model using artificial neural network. Proc. Technol. 2012;4:311–318. doi: 10.1016/j.protcy.2012.05.047. - DOI
Publication types
MeSH terms
LinkOut - more resources
Full Text Sources