Automated model-predictive design of synthetic promoters to control transcriptional profiles in bacteria - PubMed
- ️Sat Jan 01 2022
Automated model-predictive design of synthetic promoters to control transcriptional profiles in bacteria
Travis L LaFleur et al. Nat Commun. 2022.
Abstract
Transcription rates are regulated by the interactions between RNA polymerase, sigma factor, and promoter DNA sequences in bacteria. However, it remains unclear how non-canonical sequence motifs collectively control transcription rates. Here, we combine massively parallel assays, biophysics, and machine learning to develop a 346-parameter model that predicts site-specific transcription initiation rates for any σ70 promoter sequence, validated across 22132 bacterial promoters with diverse sequences. We apply the model to predict genetic context effects, design σ70 promoters with desired transcription rates, and identify undesired promoters inside engineered genetic systems. The model provides a biophysical basis for understanding gene regulation in natural genetic systems and precise transcriptional control for engineering synthetic genetic systems.
© 2022. The Author(s).
Conflict of interest statement
H.M.S. is a founder of De Novo DNA. T.L.L. and A.H. declare no competing interests.
Figures
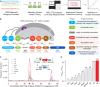
A Model development combined promoter design, barcoded oligopool synthesis, library cloning & culturing, and next-generation sequencing to measure the transcription start site distribution and transcription rate of each promoter variant. B The interaction strengths between RNAP/σ70 and promoter DNA control transcription initiation rates. 14206 promoter variants were designed to quantify how sequence modifications affect each interaction. Sequence design criteria are shown. C The frequencies of observed transcription start sites are shown for each set of promoter variants. The star indicates the predominant start site. The inset schematic shows the system architecture, the locations of the promoter and barcode variants, and the cDNA architecture after library preparation. D The transcription rates’ dynamic ranges are shown for each set of promoter variants, considering only the predominant start site. Con: UP element promoter variants with consensus hexamers. Anti: UP element promoter variants with anti-consensus hexamers. Data are provided in Supplementary Data 1.
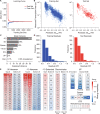
A A learning curve shows the training and testing of a ridge regression model to identify the unknown interaction energies. B Model-predicted free energies are compared to measured transcription rates for both (left) the training set and (right) the unseen test set. Pearson correlation coefficient (R2) is equal to 0.80 for both the training and test sets. C The explained variances for each promoter interaction are shown. D Histograms of model error are shown for the training and test sets, using energy units. Mean absolute error (MAE) is equal to 0.27 RT for the training set (left) and 0.28 RT for the test set (right). E The learned interaction energies are shown for the strongest and weakest ones. A poster-sized schematic showing all interaction energies is available (Supplementary Information). Data are provided in Supplementary Data 1.
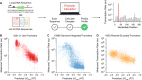
A Arbitrary DNA sequences are inputted into the model to predict its transcriptional profile (transcription rates vs. nucleotide positions) without start site information. B Model comparisons on 5391 designed promoters (LaFleur et al., this study) are compared to in vitro transcription rate measurements (R2 = 0.79, Spearman’s ρ = 0.80, MAE = 0.33 RT, MSE = 0.18 RT). C Model predictions on 10898 genome-integrated modular promoters characterized by Urtecho et al. are compared to in vivo transcription rate measurements (R2 = 0.60, Spearman’s ρ = 0.67, MAE = 0.93 RT, MSE = 1.28 RT). D Model predictions on 4350 non-repetitive plasmid-encoded promoters characterized by Hossain et al. are compared to in vivo transcription rate measurements (R2 = 0.45, Spearman’s ρ = 0.69, MAE = 1.08 RT, MSE = 1.88 RT). MAE and MSE were determined by fitting a proportionality constant (best-fit slope) accounting for experimental variation. Data are provided in Supplementary Data 1.
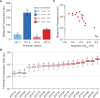
A Measured in vivo transcription rates for the J23101 promoter genetic part with varied upstream (UP) and downstream (ITR) sequences, showing transcriptional context effects. Gray dots denote independent measurements (n = 4 biological replicates). Bars denote each variants measured mean ± standard deviation. B Transcriptional context effects are quantified for common promoter genetic parts, showing predicted transcription rates when varying 30 bp of the upstream and downstream sequences. Distributions were created using 10,000 simulations (n = 10,000). Box bounds were defined by the first and third quartiles of the distribution, center lines by the median, whiskers by the minima and maxima, and black dots by outliers. C Model predictions are compared to in vivo fluorescence measurements for these promoters when specifying their upstream and downstream sequences (R2 = 0.79, Spearman’s ρ = 0.85). Data are provided in Supplementary Data 1.
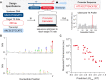
A Promoter sequences were forward engineered with desired transcription rates and start sites. B Sequence analysis of the 75 bp forward engineered promoters showed low sequence similarity. C Model predictions are compared to in vivo transcription rate measurements for the 35 designed promoters (R2 = 0.80, Spearman’s ρ = 0.89). Red dots denote the mean of duplicate measurements (n = 2 biological replicates). Data are provided in Supplementary Data 1.
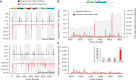
A The predicted σ70 transcriptional profile of an 11-promoter genetic circuit is compared to in vivo transcription rate and start site measurements on the (top) sense and (bottom) anti-sense strand. Transcription flux ratios are the measured differences in adjacent mRNA levels from RNA-Seq. White and gray shadows correspond to each transcribed cistron. The horizontal dotted black lines show the minimum transcription rates that define a start site. Experimental TSS cutoffs are previously described, and prediction cutoffs are defined in the Methods. Annotated start sites are depicted in the circuit diagram with arrows. B The predicted σ70 transcriptional profile for the PBAD-amtR portion of the circuit in the OFF state. Red lines are model predictions, and gray overlays show measured RNAP flux. C The predicted σ70 transcriptional profile for a redesigned amtR protein coding sequence that minimized the transcription initiation rate inside the coding sequence. (inset) Measured fluorescence levels for designed no-promoter regions predicted to have minimal transcription rates (NP1: 120-bp, NP2: 60-bp) as compared to (WC) white cells and the J23100 promoter. Black dots denote independent measurements. Bars denote the mean across duplicate measurements (n = 2 biological replicates). Data are provided in Supplementary Data 1.
Similar articles
-
Local and global regulation of transcription initiation in bacteria.
Browning DF, Busby SJ. Browning DF, et al. Nat Rev Microbiol. 2016 Oct;14(10):638-50. doi: 10.1038/nrmicro.2016.103. Epub 2016 Aug 8. Nat Rev Microbiol. 2016. PMID: 27498839 Review.
-
Sigma and RNA polymerase: an on-again, off-again relationship?
Mooney RA, Darst SA, Landick R. Mooney RA, et al. Mol Cell. 2005 Nov 11;20(3):335-45. doi: 10.1016/j.molcel.2005.10.015. Mol Cell. 2005. PMID: 16285916 Review.
-
Vuthoori S, Bowers CW, McCracken A, Dombroski AJ, Hinton DM. Vuthoori S, et al. J Mol Biol. 2001 Jun 8;309(3):561-72. doi: 10.1006/jmbi.2001.4690. J Mol Biol. 2001. PMID: 11397080
-
Ramaniuk O, Převorovský M, Pospíšil J, Vítovská D, Kofroňová O, Benada O, Schwarz M, Šanderová H, Hnilicová J, Krásný L. Ramaniuk O, et al. J Bacteriol. 2018 Aug 10;200(17):e00251-18. doi: 10.1128/JB.00251-18. Print 2018 Sep 1. J Bacteriol. 2018. PMID: 29914988 Free PMC article.
-
Baldus JM, Buckner CM, Moran CP Jr. Baldus JM, et al. Mol Microbiol. 1995 Jul;17(2):281-90. doi: 10.1111/j.1365-2958.1995.mmi_17020281.x. Mol Microbiol. 1995. PMID: 7494477
Cited by
-
Engineering activatable promoters for scalable and multi-input CRISPRa/i circuits.
Alba Burbano D, Cardiff RAL, Tickman BI, Kiattisewee C, Maranas CJ, Zalatan JG, Carothers JM. Alba Burbano D, et al. Proc Natl Acad Sci U S A. 2023 Jul 25;120(30):e2220358120. doi: 10.1073/pnas.2220358120. Epub 2023 Jul 18. Proc Natl Acad Sci U S A. 2023. PMID: 37463216 Free PMC article.
-
Genetic Circuit Design in Rhizobacteria.
Dundas CM, Dinneny JR. Dundas CM, et al. Biodes Res. 2022 Sep 1;2022:9858049. doi: 10.34133/2022/9858049. eCollection 2022. Biodes Res. 2022. PMID: 37850138 Free PMC article. Review.
-
Radde N, Mortensen GA, Bhat D, Shah S, Clements JJ, Leonard SP, McGuffie MJ, Mishler DM, Barrick JE. Radde N, et al. Nat Commun. 2024 Jul 24;15(1):6242. doi: 10.1038/s41467-024-50639-9. Nat Commun. 2024. PMID: 39048554 Free PMC article.
-
Peng P, Yang J, DiSpirito AA, Semrau JD. Peng P, et al. Appl Environ Microbiol. 2023 Dec 21;89(12):e0160123. doi: 10.1128/aem.01601-23. Epub 2023 Nov 28. Appl Environ Microbiol. 2023. PMID: 38014956 Free PMC article.
-
Diebold PJ, Rhee MW, Shi Q, Trung NV, Umrani F, Ahmed S, Kulkarni V, Deshpande P, Alexander M, Thi Hoa N, Christakis NA, Iqbal NT, Ali SA, Mathad JS, Brito IL. Diebold PJ, et al. Nat Commun. 2023 Nov 14;14(1):7366. doi: 10.1038/s41467-023-42998-6. Nat Commun. 2023. PMID: 37963868 Free PMC article.
References
Publication types
MeSH terms
Substances
LinkOut - more resources
Full Text Sources