Micapipe: A pipeline for multimodal neuroimaging and connectome analysis - PubMed
doi: 10.1016/j.neuroimage.2022.119612. Epub 2022 Sep 5.
Jessica Royer 2 , Peer Herholz 3 , Sara Larivière 4 , Reinder Vos de Wael 4 , Casey Paquola 5 , Oualid Benkarim 4 , Bo-Yong Park 6 , Janie Degré-Pelletier 7 , Mark C Nelson 8 , Jordan DeKraker 4 , Ilana R Leppert 8 , Christine Tardif 8 , Jean-Baptiste Poline 8 , Luis Concha 9 , Boris C Bernhardt 10
Affiliations
- PMID: 36070839
- PMCID: PMC10697132
- DOI: 10.1016/j.neuroimage.2022.119612
Micapipe: A pipeline for multimodal neuroimaging and connectome analysis
Raúl R Cruces et al. Neuroimage. 2022 Nov.
Abstract
Multimodal magnetic resonance imaging (MRI) has accelerated human neuroscience by fostering the analysis of brain microstructure, geometry, function, and connectivity across multiple scales and in living brains. The richness and complexity of multimodal neuroimaging, however, demands processing methods to integrate information across modalities and to consolidate findings across different spatial scales. Here, we present micapipe, an open processing pipeline for multimodal MRI datasets. Based on BIDS-conform input data, micapipe can generate i) structural connectomes derived from diffusion tractography, ii) functional connectomes derived from resting-state signal correlations, iii) geodesic distance matrices that quantify cortico-cortical proximity, and iv) microstructural profile covariance matrices that assess inter-regional similarity in cortical myelin proxies. The above matrices can be automatically generated across established 18 cortical parcellations (100-1000 parcels), in addition to subcortical and cerebellar parcellations, allowing researchers to replicate findings easily across different spatial scales. Results are represented on three different surface spaces (native, conte69, fsaverage5), and outputs are BIDS-conform. Processed outputs can be quality controlled at the individual and group level. micapipe was tested on several datasets and is available at https://github.com/MICA-MNI/micapipe, documented at https://micapipe.readthedocs.io/, and containerized as a BIDS App http://bids-apps.neuroimaging.io/apps/. We hope that micapipe will foster robust and integrative studies of human brain microstructure, morphology, function, cand connectivity.
Keywords: BIDS; Connectome; MRI; Multimoda; Multiscale; Neuroimaging.
Copyright © 2022. Published by Elsevier Inc.
Figures
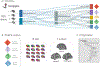
(A) Pipeline workflow. B) Outputs can be generated across 18 different cortical parcellations (100–1000 parcels), in addition to subcortical and cerebellar parcellations. Most results are mapped to three different surface spaces: native, conte69 and fsaverage5. C) Outputs are hierarchically ordered with BIDS-conform naming.
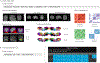
(A) Individual level quality control (QC), which can be run at any point during the processing. The QC procedure will generate a html report file for each subject containing visualizations of intermediate files for volume visualization, cross-modal co-registrations, and surface parcellations. Moreover, it allows inspection of inter-regional matrices such as the structural connectome (from diffusion MRI tractography), the functional connectome (from resting-state fMRI signal correlation), the microstructural profile covariance matrix (from correlations of intracortical microstructural profiles), and geodesic distance matrices. (B) QC can also be run at a group/dataset-level. The report consists of a color-coded table with rows as subjects and columns as the pipeline modules (blue: completed, orange: incomplete/error, dark gray: not processed).
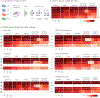
Within dataset mean consistency, indicating the correlation between subject- and group-level measurements (Spearman’s rho), for Schaefer-100, Schaefer-400, and Schaefer-1000 parcellations. (A) For each modality, five measurements were evaluated: principal gradient, edges, node strength, path length, and clustering coefficient. Empty rows indicate modalities that were not analyzed due to missing acquisitions. (B,C) Across datasets, correlations were highest for GD and SC, followed by FC and MPC. Gradient 1 was the most consistent measure across parcellations and modalities, followed by edges and node strength. Overall, characteristic path length was similar at lower granularity (100 parcels) but increasingly dissimilar at higher granularity (1000 parcels). Clustering coefficient had variable patterns depending on the modality and granularity. MPC: microstructural profile covariance, FC: functional connectivity, SC structural connectivity, GD geodesic distance.
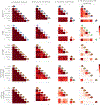
We assessed consistency of matrix parameters across datasets using Spearman’s rho correlation coefficient for the same features as in Fig. 3A. Each column represents the different modality matrices: (A) geodesic distance, (B) structural connectome, (C) functional connectome, and (D) microstructural profile covariance. The diagonal shows the mean value plotted on the surface of each dataset by measurement. As for the within-dataset analysis, we found the highest similarities between datasets for GD and SC, followed by FC and MPC. GD, SC, and FC showed high similarity between datasets for the edges, first eigenvector/gradient, and node strength. FC had decreased consistency between datasets for characteristic path length and clustering coefficient. MPC had the lowest between dataset consistency for all measurements. Empty matrix entries (coloured in white) indicate missing data for a given dataset.
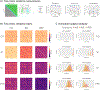
We assessed the capability of micapipe to generate reproducible outcomes in a test-retest scenario, adoping a prior framework (Seguin et al., 2022). For all modalities and three parcellations, we evaluated the similarity between matrices of two different acquisitions (53 subjects from HCP, run-1 and run-2 scans). (A) From each similarity matrix of subject-test by subject-retest, we computed three measures of similarity: reliability (intra-subject), uniformity (inter-subject), and identifiability (effect size between intra- and inter-). Reliability quantifies the mean processing consistency for an individual; uniformity quantifies the mean conformity of matrices belonging to different individuals, and identifiability quantifies how an individual can be recognized from a group based on the matrix features. The scatter plots with lines show the mean values of each similarity measure for each modality over three granularities (Schaefer-100, 400 and 1000). (B) Similarity matrices for each modality and granularity. (C) Density plots of the reliability and uniformity by modality and granularity of all subject pairs. For all feature matrices, we found higher reliability than uniformity, with excellent performance for GD and SC, and good results for FC and MPC. Overall, less granular parcellation data had higher similarity than more granular parcellation data across all modalities. GD=geodesic distance, SC=structural connectome, FC=functional connectome, and MPC=microstructural profile covariance.
Similar articles
-
Schirner M, Rothmeier S, Jirsa VK, McIntosh AR, Ritter P. Schirner M, et al. Neuroimage. 2015 Aug 15;117:343-57. doi: 10.1016/j.neuroimage.2015.03.055. Epub 2015 Mar 31. Neuroimage. 2015. PMID: 25837600
-
An Open MRI Dataset For Multiscale Neuroscience.
Royer J, Rodríguez-Cruces R, Tavakol S, Larivière S, Herholz P, Li Q, Vos de Wael R, Paquola C, Benkarim O, Park BY, Lowe AJ, Margulies D, Smallwood J, Bernasconi A, Bernasconi N, Frauscher B, Bernhardt BC. Royer J, et al. Sci Data. 2022 Sep 15;9(1):569. doi: 10.1038/s41597-022-01682-y. Sci Data. 2022. PMID: 36109562 Free PMC article.
-
BrainSuite BIDS App: Containerized Workflows for MRI Analysis.
Kim Y, Joshi AA, Choi S, Joshi SH, Bhushan C, Varadarajan D, Haldar JP, Leahy RM, Shattuck DW. Kim Y, et al. bioRxiv [Preprint]. 2024 Sep 9:2023.03.14.532686. doi: 10.1101/2023.03.14.532686. bioRxiv. 2024. PMID: 36993283 Free PMC article. Preprint.
-
The nonhuman primate neuroimaging and neuroanatomy project.
Hayashi T, Hou Y, Glasser MF, Autio JA, Knoblauch K, Inoue-Murayama M, Coalson T, Yacoub E, Smith S, Kennedy H, Van Essen DC. Hayashi T, et al. Neuroimage. 2021 Apr 1;229:117726. doi: 10.1016/j.neuroimage.2021.117726. Epub 2021 Jan 20. Neuroimage. 2021. PMID: 33484849 Free PMC article. Review.
-
The Human Connectome Project's neuroimaging approach.
Glasser MF, Smith SM, Marcus DS, Andersson JL, Auerbach EJ, Behrens TE, Coalson TS, Harms MP, Jenkinson M, Moeller S, Robinson EC, Sotiropoulos SN, Xu J, Yacoub E, Ugurbil K, Van Essen DC. Glasser MF, et al. Nat Neurosci. 2016 Aug 26;19(9):1175-87. doi: 10.1038/nn.4361. Nat Neurosci. 2016. PMID: 27571196 Free PMC article. Review.
Cited by
-
Serio B, Hettwer MD, Wiersch L, Bignardi G, Sacher J, Weis S, Eickhoff SB, Valk SL. Serio B, et al. bioRxiv [Preprint]. 2023 Nov 23:2023.11.23.568437. doi: 10.1101/2023.11.23.568437. bioRxiv. 2023. PMID: 38045320 Free PMC article. Updated. Preprint.
-
Newman BT, Jacokes Z, Venkadesh S, Webb SJ, Kleinhans NM, McPartland JC, Druzgal TJ, Pelphrey KA, Van Horn JD; GENDAAR Research Consortium. Newman BT, et al. PLoS One. 2024 Apr 17;19(4):e0301964. doi: 10.1371/journal.pone.0301964. eCollection 2024. PLoS One. 2024. PMID: 38630783 Free PMC article.
-
Raj A, Sipes BS, Verma P, Mathalon DH, Biswal B, Nagarajan S. Raj A, et al. bioRxiv [Preprint]. 2024 Mar 27:2024.03.22.586305. doi: 10.1101/2024.03.22.586305. bioRxiv. 2024. PMID: 38586057 Free PMC article. Preprint.
-
Microstructural asymmetry in the human cortex.
Wan B, Saberi A, Paquola C, Schaare HL, Hettwer MD, Royer J, John A, Dorfschmidt L, Bayrak Ş, Bethlehem RAI, Eickhoff SB, Bernhardt BC, Valk SL. Wan B, et al. Nat Commun. 2024 Nov 22;15(1):10124. doi: 10.1038/s41467-024-54243-9. Nat Commun. 2024. PMID: 39578424 Free PMC article.
-
Serio B, Hettwer MD, Wiersch L, Bignardi G, Sacher J, Weis S, Eickhoff SB, Valk SL. Serio B, et al. Nat Commun. 2024 Sep 4;15(1):7714. doi: 10.1038/s41467-024-51942-1. Nat Commun. 2024. PMID: 39231965 Free PMC article.
References
-
- Angeles-Valdez D, Rasgado-Toledo J, Issa-Garcia V, Balducci T, Villicaña V, Valencia A, Gonzalez-Olvera JJ, Reyes-Zamorano E, Garza-Villarreal EA, 2022. The Mexican magnetic resonance imaging dataset of patients with cocaine use disorder: SUDMEX CONN. Scientific data 9 (1), 1–10. doi: 10.1038/s41597-022-01251-3. - DOI - PMC - PubMed
Publication types
MeSH terms
LinkOut - more resources
Full Text Sources