Resolving tricky nodes in the tree of life through amino acid recoding - PubMed
- ️Sat Jan 01 2022
Resolving tricky nodes in the tree of life through amino acid recoding
Mattia Giacomelli et al. iScience. 2022.
Abstract
Genomic data allowed a detailed resolution of the Tree of Life, but "tricky nodes" such as the root of the animals remain unresolved. Genome-scale datasets are heterogeneous as genes and species are exposed to different pressures, and this can negatively impacts phylogenetic accuracy. We use simulated genomic-scale datasets and show that recoding amino acid data improves accuracy when the model does not account for the compositional heterogeneity of the amino acid alignment. We apply our findings to three datasets addressing the root of the animal tree, where the debate centers on whether sponges (Porifera) or comb jellies (Ctenophora) represent the sister of all other animals. We show that results from empirical data follow predictions from simulations and suggest that, at the least in phylogenies inferred from amino acid sequences, a placement of the ctenophores as sister to all the other animals is best explained as a tree reconstruction artifact.
Keywords: Biological sciences; Evolutionary biology; Phylogenetics.
© 2022 The Authors.
Conflict of interest statement
The authors declare no competing interests.
Figures
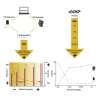
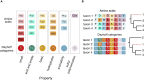
Recoding data The six-bin Dayhoff-6 recoding scheme, see STAR methods, is the most widely used recoding strategy, and is the one primarily tested in this study. Dayhoff-6 recoding partitions amino acids into six differently sized bins (see STAR methods), based on how frequently they are expected to exchange with each other. (A) The bins of the Dayhoff-6 scheme and the biochemical properties of the amino acids in each bin. (B) An exemplar amino acid dataset and its Dayhoff-6 recoded representation. Dayhoff-6 recoding is achieved by replacing, in multiple sequence alignments, one letter amino acid codes with one letter codes representing the bin where the considered amino acid is clustered.
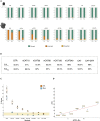
Recoding the data improves accuracy when the model fails to fit the amino acid alignment (A) Accuracy of amino acids and recoded data as models that can account for more across-sites compositional heterogeneity are used. (B) Table summarizing the Total Accuracy (TA) for amino acids and recoded data under each model. TA is calculated (from the values in A) as the percentage of accurate trees (see STAR methods) under both Porifera- and Ctenophora-sister. (C) Change in the fit (expressed as Z-scores) of the model to the data (estimated using PPA-Div) as models that can account for more across-sites compositional heterogeneity are used. In Orange amino acid datasets; in Blue recoded datasets. (D) Correlation between the difference in Z-scores achieved by each considered model on the amino acid and recoded datasets (δPPA-Div), against the difference in TA achieved before and after recoding (δTA). See Figures S4–S7 for sensitivity tests showing that our conclusions would not have changed if we used Maximum Likelihood instead of Bayesian analyses, if we run our Bayesian analyses 8,000 more generations (convergence was achieved before 1000 cycles), if we used a more stringent threshold to define success (PP = 0.95 instead of PP = 0.5), and if we used alternative recoding schemes (SR6 and KGB6 see STAR methods for details) instead of Dayhoff-6.
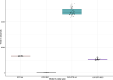
Recoding as a tool in green phylogenomics Time taken to complete 50 GTR and CAT-GTR analyses of amino acid and recoded datasets. Recoded analyses are invariably completed in a shorter time, with the difference becoming significantly more marked when using the complex CAT-GTR model. Given the high accuracy of recoded analyses this result suggests that recoding could play a significant role in the development of “green phylogenomics”.
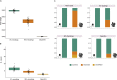
Dayhoff-6 outperforms random recoding schemes (A) Boxplot representing the distribution of tree lengths for 0%-recoded, 90%-recoded, and Dayhoff-6 recoded dataset. (B) Comparison of PPA-Div scores for 0%-recoded, 90%-recoded, and Dayhoff-6 recoded datasets. The figure indicates that PPA-Div scores of Dayhoff-6 recoded data are significantly better than PPA-Div scores from 0%-recoded data – the distributions do not overlap. (C) A comparison of the TA values achieved by amino acids data, 0%-recoded, 90-%recoded and Dayhoff-6 recoded data, indicating that Dayhoff-6 outperforms both amino acids and randomly recoded data.
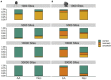
Accuracy of recoded data increases with alignment size (A) Accuracy of amino acid and recoded datasets of 1,000, 5,000, 10,000 and 30,000 sites analyzed under nCAT10, when the generating tree assumes Ctenophora-sister to be true. (B) Success rate of amino acid and Dayhoff-6 datasets of 1,000, 5,000, 10,000 and 30,000 sites analyzed under nCAT10, when the generating tree assumes Porifera-sister to be true. Analyses performed in Phylobayes. In Green: Correct trees; Dark Orange: Incorrect Trees; Light Orange: Uncertain trees.
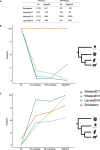
Results from empirical datasets follow predictions from simulations (A) PPA-Div and PPA-Mean scores for all three empirical datasets when the alignments are analyzed as amino acids and Dayhoff-6 recoded data. Average PPA scores for our simulated data are also reported (under nCAT10), indicating that PPA scores achieved for the simulated data under nCAT10 are comparable to those achieved under nCAT60 for the empirical datasets. (B) Support for Ctenophora-sister as different data types (amino acids, 0%-recodings, 90%-recodings, and Dayhoff-6) are used. (C) Support for Porifera-sister as different data types (amino acids, 0%-recodings, 90%-recodings, and Dayhoff-6) are used. In Orange: reference support values obtained for the two considered clades in our simulations. Light Green: Whelan2015; Dark Green Whelan2017; Blue Laumer2019. Note: Support values for 0%-recodings and 90%-recodings represent average values calculated over all random recoding generated.
Similar articles
-
Improved Modeling of Compositional Heterogeneity Supports Sponges as Sister to All Other Animals.
Feuda R, Dohrmann M, Pett W, Philippe H, Rota-Stabelli O, Lartillot N, Wörheide G, Pisani D. Feuda R, et al. Curr Biol. 2017 Dec 18;27(24):3864-3870.e4. doi: 10.1016/j.cub.2017.11.008. Epub 2017 Nov 30. Curr Biol. 2017. PMID: 29199080
-
Genomic data do not support comb jellies as the sister group to all other animals.
Pisani D, Pett W, Dohrmann M, Feuda R, Rota-Stabelli O, Philippe H, Lartillot N, Wörheide G. Pisani D, et al. Proc Natl Acad Sci U S A. 2015 Dec 15;112(50):15402-7. doi: 10.1073/pnas.1518127112. Epub 2015 Nov 30. Proc Natl Acad Sci U S A. 2015. PMID: 26621703 Free PMC article.
-
Redmond AK, McLysaght A. Redmond AK, et al. Nat Commun. 2021 Mar 19;12(1):1783. doi: 10.1038/s41467-021-22074-7. Nat Commun. 2021. PMID: 33741994 Free PMC article.
-
The ctenophore lineage is older than sponges? That cannot be right! Or can it?
Halanych KM. Halanych KM. J Exp Biol. 2015 Feb 15;218(Pt 4):592-7. doi: 10.1242/jeb.111872. J Exp Biol. 2015. PMID: 25696822 Review.
-
The hidden biology of sponges and ctenophores.
Dunn CW, Leys SP, Haddock SH. Dunn CW, et al. Trends Ecol Evol. 2015 May;30(5):282-91. doi: 10.1016/j.tree.2015.03.003. Epub 2015 Mar 31. Trends Ecol Evol. 2015. PMID: 25840473 Review.
Cited by
-
Redmond AK. Redmond AK. Proc Biol Sci. 2024 Sep;291(2031):20240329. doi: 10.1098/rspb.2024.0329. Epub 2024 Sep 18. Proc Biol Sci. 2024. PMID: 39288803 Free PMC article.
-
The monoaminergic system is a bilaterian innovation.
Goulty M, Botton-Amiot G, Rosato E, Sprecher SG, Feuda R. Goulty M, et al. Nat Commun. 2023 Jun 6;14(1):3284. doi: 10.1038/s41467-023-39030-2. Nat Commun. 2023. PMID: 37280201 Free PMC article.
-
Identifying and addressing methodological incongruence in phylogenomics: A review.
Fleming JF, Valero-Gracia A, Struck TH. Fleming JF, et al. Evol Appl. 2023 Jun 6;16(6):1087-1104. doi: 10.1111/eva.13565. eCollection 2023 Jun. Evol Appl. 2023. PMID: 37360032 Free PMC article. Review.
-
Reply to: Available data do not rule out Ctenophora as the sister group to all other Metazoa.
Redmond AK, McLysaght A. Redmond AK, et al. Nat Commun. 2023 Feb 10;14(1):710. doi: 10.1038/s41467-023-36152-5. Nat Commun. 2023. PMID: 36765060 Free PMC article. No abstract available.
-
Improving Orthologous Signal and Model Fit in Datasets Addressing the Root of the Animal Phylogeny.
McCarthy CGP, Mulhair PO, Siu-Ting K, Creevey CJ, O'Connell MJ. McCarthy CGP, et al. Mol Biol Evol. 2023 Jan 4;40(1):msac276. doi: 10.1093/molbev/msac276. Mol Biol Evol. 2023. PMID: 36649189 Free PMC article.
References
-
- Dunn C.W., Giribet G., Edgecombe G.D., Hejnol A. Animal phylogeny and its evolutionary implications. Annu. Rev. Ecol. Evol. Syst. 2014;45:371–395. doi: 10.1146/annurev-ecolsys-120213-091627. - DOI
LinkOut - more resources
Full Text Sources