Differentially Expressed Genes, miRNAs and Network Models: A Strategy to Shed Light on Molecular Interactions Driving HNSCC Tumorigenesis - PubMed
- ️Sun Jan 01 2023
Differentially Expressed Genes, miRNAs and Network Models: A Strategy to Shed Light on Molecular Interactions Driving HNSCC Tumorigenesis
Saniya Arfin et al. Cancers (Basel). 2023.
Abstract
Head and neck squamous cell carcinoma (HNSCC) is among the most common cancer worldwide, accounting for hundreds thousands deaths annually. Unfortunately, most patients are diagnosed in an advanced stage and only a percentage respond favorably to therapies. To help fill this gap, we hereby propose a retrospective in silico study to shed light on gene-miRNA interactions driving the development of HNSCC. Moreover, to identify topological biomarkers as a source for designing new drugs. To achieve this, gene and miRNA profiles from patients and controls are holistically reevaluated using protein-protein interaction (PPI) and bipartite miRNA-target networks. Cytoskeletal remodeling, extracellular matrix (ECM), immune system, proteolysis, and energy metabolism have emerged as major functional modules involved in the pathogenesis of HNSCC. Of note, the landscape of our findings depicts a concerted molecular action in activating genes promoting cell cycle and proliferation, and inactivating those suppressive. In this scenario, genes, including VEGFA, EMP1, PPL, KRAS, MET, TP53, MMPs and HOXs, and miRNAs, including mir-6728 and mir-99a, emerge as key players in the molecular interactions driving HNSCC tumorigenesis. Despite the heterogeneity characterizing these HNSCC subtypes, and the limitations of a study pointing to relationships that could be context dependent, the overlap with previously published studies is encouraging. Hence, it supports further investigation for key molecules, both those already and not correlated to HNSCC.
Keywords: DEGs; PPI network; bipartite network; head and neck cancer; hubs; miRNAs; network topology.
Conflict of interest statement
The authors declare no conflict of interest. The funders had no role in the design of the study; in the collection, analyses, or interpretation of data; in the writing of the manuscript; or in the decision to publish the results.
Figures
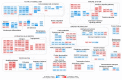
Protein–protein interaction functional modules differentially expressed by comparing HNSCC and control samples. In red (FC ≥ 2, adjP ≤ 0.01), genes upregulated in HNSCC (and downregulated in control), while in blue (FC ≤ −2, adjP ≤ 0.01), genes upregulated in control (and downregulated in HNSCC).
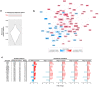
Protein–protein interaction network topology. (a) Violin plot of betweenness values from random networks (n = 1000) calculated from nodes (DEGs) in Reference Network. Compared to the reference network (Av. betweenness: 1384), random ones show significantly different average values and support the selection of hubs. (b) Hubs selected by betweenness, centroid and bridging centralities; all hubs had to show values higher than the average of the entire network. Red nodes indicate hubs upregulated in HNSCC (and downregulated in controls), while blue nodes indicate hubs upregulated in control (and downregulated in HNSCC). (c) Trend of expression, per disease stage, of the 20 best ranked hubs based on betweenness. Full red bars (FC ≥ 1.5, adjP ≤ 0.01) show the fold change of genes upregulated in HNSCC, while full blue bars (FC ≤ −1.5, adjP ≤ 0.01) show the fold change of genes upregulated in controls. For each gene, it is shown also the corresponding fold change at different stages (empty bars, adjP ≤ 0.01). Most hubs were upregulated in HNSCC and in all stages (red bars).
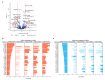
Differentially expressed miRNAs. (a) Volcano plot showing differentially expressed miRNAs by comparing HNSCC and control profiles (FC ≥ 2 AND p values ≤ 0.00001). (b) Stage-level expression of miRNAs upregulated in HNSCC samples (p values ≤ 0.01). (c) Stage-level expression of miRNAs downregulated in HNSCC samples (p values ≤ 0.01). Full bars indicate the most significant differences (FC ≥ 2 AND p values ≤ 0.00001).
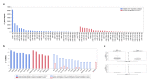
Differentially expressed miRNAs and corresponding target genes. (a) Up- and downregulated miRNAs ranked based on the number of genes they target. (b) Full bars indicate genes specifically targeted by up- and downregulated miRNAs and selected based on a Degree > 2∗Average Degree. Empty bars indicate differentially expressed genes (DEGs) most targeted by up- or downregulated miRNAs. (c) Differentially expressed genes (DEGs) in HNSCC, and number of miRNAs up- and down-regulated targeting them; Kolmogorov–Smirnov test (p ≤ 0.05).
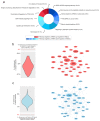
Pathways and PPI hubs from gene targets of up- and downregulated miRNAs. (a) Pathways enriched from gene targets of up- (red) and downregulated (blue) miRNAs in HNSCC. (b) On the left, violin plot of betweenness values from PPI random networks reconstructed from gene targets of miRNAs upregulated in HNSCC. Compared to the reference network (Av. betweenness: 515), random ones show significantly different average values and support the selection of hubs (on the right). (c) On the left, violin plot of average betweenness values from PPI random networks reconstructed from gene targets of miRNAs downregulated in HNSCC. Compared to the reference network (Av. betweenness: 457), random ones show significantly different average values and support the selection of hubs (on the right). All hubs were selected by betweenness, bridging and centroid centralities, which had to show values higher than the average of the entire network.
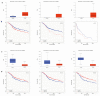
Differential miRNAs expression and survival analysis. (a) Expression level of miRNAs upregulated in tumor (miR-301a, miR-3144, miR-6728) and (b) survival analysis in HNSCC patients (Control, n = 44; Tumor, n = 482). (c) Expression level of miRNAs downregulated in tumor (miR-29c, miR-378a, miR-99a) and (d) survival analysis in HNSCC patients (Control, n = 44; Tumor, n = 482).
Similar articles
-
Okada R, Koshizuka K, Yamada Y, Moriya S, Kikkawa N, Kinoshita T, Hanazawa T, Seki N. Okada R, et al. Cells. 2019 Nov 28;8(12):1535. doi: 10.3390/cells8121535. Cells. 2019. PMID: 31795200 Free PMC article.
-
Gong SQ, Xu M, Xiang ML, Shan YM, Zhang H. Gong SQ, et al. Front Oncol. 2019 Jul 31;9:678. doi: 10.3389/fonc.2019.00678. eCollection 2019. Front Oncol. 2019. PMID: 31417866 Free PMC article.
-
Jin Y, Qin X. Jin Y, et al. Ann Transl Med. 2020 Mar;8(6):282. doi: 10.21037/atm.2020.03.30. Ann Transl Med. 2020. PMID: 32355726 Free PMC article.
-
Starska-Kowarska K. Starska-Kowarska K. Nutrients. 2023 May 31;15(11):2592. doi: 10.3390/nu15112592. Nutrients. 2023. PMID: 37299554 Free PMC article. Review.
Cited by
-
Construction and validation of a prognostic model of angiogenesis-related genes in multiple myeloma.
Hu R, Chen F, Yu X, Li Z, Li Y, Feng S, Liu J, Li H, Shen C, Gu X, Lu Z. Hu R, et al. BMC Cancer. 2024 Oct 11;24(1):1269. doi: 10.1186/s12885-024-13024-9. BMC Cancer. 2024. PMID: 39394121 Free PMC article.
-
Biyu H, Mengshan L, Yuxin H, Ming Z, Nan W, Lixin G. Biyu H, et al. BMC Cancer. 2024 Jun 5;24(1):683. doi: 10.1186/s12885-024-12420-5. BMC Cancer. 2024. PMID: 38840078 Free PMC article.
References
LinkOut - more resources
Full Text Sources
Research Materials
Miscellaneous