The mitochondrial energy metabolism pathway-related signature predicts prognosis and indicates immune microenvironment infiltration in osteosarcoma - PubMed
- ️Sun Jan 01 2023
The mitochondrial energy metabolism pathway-related signature predicts prognosis and indicates immune microenvironment infiltration in osteosarcoma
Sen Yang et al. Medicine (Baltimore). 2023.
Abstract
Background: Abnormalities in the mitochondrial energy metabolism pathways are closely related to the occurrence and development of many cancers. Furthermore, abnormal genes in mitochondrial energy metabolism pathways may be novel targets and biomarkers for the diagnosis and treatment of osteosarcoma. In this study, we aimed to establish a mitochondrial energy metabolism-related gene signature for osteosarcoma prognosis.
Methods: We first obtained differentially expressed genes based on the metastatic status of 84 patients with osteosarcoma from the TARGET database. After Venn analysis of differentially expressed genes and mitochondrial energy metabolism pathway-related genes (MMRGs), 2 key genes were obtained using univariate Cox regression and least absolute shrinkage and selection operator (LASSO) regression analysis. Next, we used these 2 genes to establish a prognostic signature. Subsequent analyses elucidated the correlation between these 2 key genes with clinical features and 28 types of immune cells. Pathway changes in osteosarcoma pathogenesis under different metastatic states were clarified using gene set enrichment analysis (GSEA) of differentially expressed genes.
Results: A gene signature composed of 2 key prognosis-related genes (KCNJ5 and PFKFB2) was identified. A risk score was calculated based on the gene signature, which divided osteosarcoma patients into low- or high-risk groups that showed good and poor prognosis, respectively. High expression of these 2 key genes is associated with low-risk group in patients with osteosarcoma. We constructed an accurate nomogram to help clinicians assess the survival time of patients with osteosarcoma. The results of immune cell infiltration level showed that the high-risk group had lower levels of immune cell infiltration. GSEA revealed changes in immune regulation and hypoxia stress pathways in osteosarcoma under different metastatic states.
Conclusion: Our study identified an excellent gene signature that could be helpful in improving the prognosis of patients with osteosarcoma.
Copyright © 2023 the Author(s). Published by Wolters Kluwer Health, Inc.
Conflict of interest statement
The authors have no conflicts of interest to disclose.
Figures
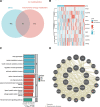
Identification and functional enrichment analysis of MMRGs between Osteosarcoma patients with metastasis and non-metastasis group. (A) Venn analysis of the intersection between MMRGs and DEGs identified. (B) Heatmap visualizing the expression of 10 DEGs related to the mitochondrial energy metabolism pathway in the 2 subgroups. (C) Terms of Gene Ontology (GO) enrichment analysis and KEGG pathways related to the 10 MMRGs. (D) PPI network construction of differentially expressed MMRGs. DEGs = differentially expressed genes, KEGG = Kyoto encyclopedia of genes and genomes, MMRGs = mitochondrial energy metabolism pathway-related genes, PPI = protein–protein interaction.
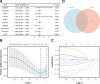
Construction of a risk prognostic model based on 10 MMRGs. (A) Univariate Cox regression analysis was performed for all prognosis-related genes. The value of P < .05 was considerate statistically significant. (B) The LASSO regression model of the 10 MMRGs performed by Lasso-ten-fold cross-validation. (C) Cross-validation for tuning the parameter selection in the LASSO regression. (D) Venn diagram of the intersection between the LASSO regression model and Univariate Cox regression analysis. LASSO = least absolute shrinkage and selection operator, MMRGs = mitochondrial energy metabolism pathway-related genes.
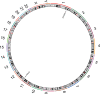
Chromosomal mapping of 2 prognosis-related genes.
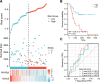
Construction and validation of risk score model in 84 Osteosarcoma patients. (A) The risk score, survival time distributions and gene expression heat map of MMRGs in the Osteosarcoma patients. (B) The ROC curves of the risk scoring model predicting overall survival of 1-yr, 3-yr, and 5-yr. (C) Kaplan–Meier curves showing the overall survival of patients in the high-risk and low-risk groups. MMRGs = mitochondrial energy metabolism pathway-related genes, ROC = receiver operating characteristic.
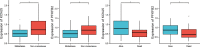
Expression of KCNJ5 and PFKFB2 in different survival and metastases states.
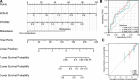
Prognostic nomogram for the 1-yr, 3-yr, and 5-yr OS of osteosarcoma patients. (A) The independent risk factors that affect the OS of osteosarcoma patients were incorporated into the nomogram model. (B) The ROC curves for predicting the nomogram of 1-yr, 3-yr, and 5-yr OS. (C) The nomogram calibration curves for predicting the 1-yr, 3-yr, and 5-yr OS. OS = overall survival, ROC = receiver operating characteristic.
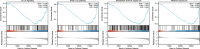
Enrichment plots from GSEA. Several pathways and biological processes were differentially enriched in the osteosarcoma patients. (A) LU IL4 Signaling. (B) PID_IL12_2PATHWAY. (C) WIERENGA STAT5A Targets Dn. (D) WINTER Hypoxia Dn. GSEA = gene set enrichment analysis.
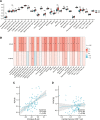
Assessing the potential of the gene signature in immune cell infiltration level. (A) Difference in 28 kinds of immune cell infiltration between high- and low-risk group (*P < .05; **P < .01; ***P < .001). (B) The correlation between 28 kinds of immune cells and 2 key genes. (C) Correlation analysis between KCNJ5 expression and immature B cells. (D) Correlation analysis between PFKFB2 expression and central memory CD8 T cells.
Similar articles
-
Lv Y, Wu L, Jian H, Zhang C, Lou Y, Kang Y, Hou M, Li Z, Li X, Sun B, Zhou H. Lv Y, et al. Front Immunol. 2022 Oct 5;13:997765. doi: 10.3389/fimmu.2022.997765. eCollection 2022. Front Immunol. 2022. PMID: 36275664 Free PMC article.
-
Hong X, Fu R. Hong X, et al. PLoS One. 2023 Dec 1;18(12):e0295364. doi: 10.1371/journal.pone.0295364. eCollection 2023. PLoS One. 2023. PMID: 38039294 Free PMC article.
-
Li Q, Huang X, Zhao Y. Li Q, et al. Cancer Biother Radiopharm. 2024 Sep;39(7):502-516. doi: 10.1089/cbr.2023.0103. Epub 2023 Oct 27. Cancer Biother Radiopharm. 2024. PMID: 37889617
-
Su Z, Wang C, Pan R, Li H, Chen J, Tan J, Tian X, Lin T, Shen J. Su Z, et al. Front Immunol. 2022 Oct 6;13:1028263. doi: 10.3389/fimmu.2022.1028263. eCollection 2022. Front Immunol. 2022. PMID: 36275679 Free PMC article.
-
Zhong Y, Zhang Y, Wei S, Chen J, Zhong C, Cai W, Jin W, Peng H. Zhong Y, et al. Front Endocrinol (Lausanne). 2022 Oct 14;13:1030655. doi: 10.3389/fendo.2022.1030655. eCollection 2022. Front Endocrinol (Lausanne). 2022. PMID: 36313783 Free PMC article.
References
MeSH terms
Substances
LinkOut - more resources
Full Text Sources
Medical