The functional role of spatial anisotropies in ensemble perception - PubMed
- ️Mon Jan 01 2024
The functional role of spatial anisotropies in ensemble perception
Natalia A Tiurina et al. BMC Biol. 2024.
Abstract
Background: The human brain can rapidly represent sets of similar stimuli by their ensemble summary statistics, like the average orientation or size. Classic models assume that ensemble statistics are computed by integrating all elements with equal weight. Challenging this view, here, we show that ensemble statistics are estimated by combining parafoveal and foveal statistics in proportion to their reliability. In a series of experiments, observers reproduced the average orientation of an ensemble of stimuli under varying levels of visual uncertainty.
Results: Ensemble statistics were affected by multiple spatial biases, in particular, a strong and persistent bias towards the center of the visual field. This bias, evident in the majority of subjects and in all experiments, scaled with uncertainty: the higher the uncertainty in the ensemble statistics, the larger the bias towards the element shown at the fovea.
Conclusion: Our findings indicate that ensemble perception cannot be explained by simple uniform pooling. The visual system weights information anisotropically from both the parafovea and the fovea, taking the intrinsic spatial anisotropies of vision into account to compensate for visual uncertainty.
Keywords: Ensemble coding; Ensemble perception; Spatial biases; Statistical perception; Summary statistics; Visual uncertainty.
© 2024. The Author(s).
Conflict of interest statement
The authors declare that they have no competing interests.
Figures
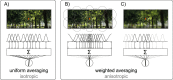
Ensemble coding in natural scenes. Example of isotropic (uniform) and weighted (anisotropic) averaging in a simple pooling scheme. A Uniform: local features (e.g., the orientations of the trees) are pooled together over the entire visual field, independently of their location and distance from the fovea (the orange fixation spot). In uniform pooling, the activity of many neurons encoding local features (depicted as a set of Gaussian tuning functions) is equally weighted and integrated over large areas of space. Integration (Σ) results in a single estimate: the average orientation of all the trees. B Weighted averaging: integration occurs in anisotropic space, due to changes in the density and size of receptive fields (illustrated by gray circles). In this case, ensemble coding is biased towards features at the center of the scene, because of the higher number and narrower tuning of neurons at the fovea. Hence, much more information is received from the central visual field. C Anisotropies due to spatial biases (here, a bias towards the center and the left-hand side is illustrated by the difference in blurring between the central and left-hand region and the rest of the image). Features inside the region of preferential processing (black tuning functions and lines) are weighted more than features outside (gray tuning functions and lines). Note that the three scenarios can lead to different average estimates (the oriented line resulting from integration)
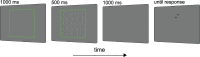
Schematic of the sequence of events on each trial. The green frame indicated the beginning of the trial and the region of the display where both the ensemble and the response tool were presented. An ensemble of oriented Gabor patches was then shown for 500 ms, followed by a blank interval before the response. The response tool appeared at random locations
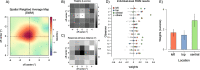
Results of experiment 1. A Spatial weighted maps (SWM) represented as interpolated heat maps (see the “Methods” section) to depict the increase in weights (in the colormap from blue to red) at central, leftward, and upward regions. B Raw weights from the spatial weighted average model (t-scores) in grayscale (white means larger weights). Asterisks indicate locations with weights significantly larger than those expected in uniform averaging (permutation statistics, see the “Methods” section). C The response-stimulus distance metric with values in grayscale (black means lower distances between the reported orientation and the orientation of the stimulus at each location). D Raw weights for the central (green dots), leftward (red dots), and upward locations (blue dots) compared to weights at all other locations (gray dots) at the individual subject level. E Bar plot of the estimated weights at the three significant locations. Error bars are standard errors of the mean (SEM)
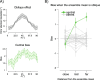
A Oblique effect (top plot, gray curve) and central bias (bottom plot, green curve) as a function of the average orientation of the ensemble. The 0–90° range of orientations in the x-axis summarizes the effects by collapsing orientations within the actual 0–90° and the 90–180° ranges. Shaded areas are SEM. B Bias towards the central vs. other locations in trials where the average ensemble orientation was at and near the obliques. Separate biases are computed as a function of the absolute distance between the orientation of the stimulus at the location of interest and the average orientation of the ensemble (e.g., for “close” distances, the stimulus had an orientation near the oblique; for “far” distances, the stimulus had an orientation away from the oblique)
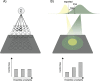
Candidate models of the central bias in ensemble statistics. A A non-uniform pooling scheme—i.e., weighted average, in which central elements are given greater integration weights compared to the rest. This model predicts a fixed bias with no changes due to the overall ensemble uncertainty (exemplified in the bottom plot). B Observers combine the representation of the stimulus at the fovea with the statistics of the elements in the parafovea. As the uncertainty in the ensemble statistics increases, the estimates in the parafovea become noisier while the representation of the central elements is unchanged: the central bias increases in strength (exemplified in the bottom plot)
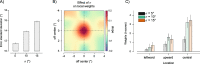
Results of experiment 2. A Standard deviation of the errors in adjustment responses as a function of the ensemble variability σ. B Effect of σ on local weight changes, showing the increase in the central bias with the increasing variability in the ensemble’s orientations. C Weights (t-scores) estimated at each of the three locations of interest (leftward, upward, central), as a function of the ensemble σ
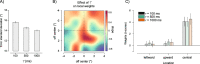
Results of experiment 3. A Standard deviation of the errors as a function of the ensemble duration τ. B Effect of τ on local weight changes. C Weights (t-scores) estimated at each of the three locations of interest (leftward, upward, central), as a function of the ensemble τ
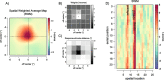
Results of experiment 4. A Spatial weighted maps (SWMs) represented as interpolated heat maps (see the “Methods” section). B, C Raw weights (t-scores) and the response-stimulus distance metric. The patterns in A–C are a clear replication of the results of experiment 1. D Matrix of weights (t-scores) at each location (columns) as a function of the serial position of each element in the sequence (rows). For example, the value in the first row, first column corresponds to the weight of the Gabor at the first location (top-left location, coded as 1) in trials where the stimulus at the top-left location appeared in the first frame of the sequence. The three locations of interest (leftward, coded as 8; upward, coded as 12; and central, coded as 13) are highlighted by their respective names
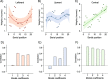
A–C Weights (t-scores) for the three locations of interest as a function of the serial position of the stimulus at the corresponding location. Dots are the weights estimated for each serial position. Prediction lines and shaded confidence intervals are obtained from the best-fitting model of each bias. Train-test correlation metric to evaluate the fit of polynomial models of increasing order for the time course of the leftward (D), upward (E), and central (F) bias
Similar articles
-
Spatial ensemble statistics are efficient codes that can be represented with reduced attention.
Alvarez GA, Oliva A. Alvarez GA, et al. Proc Natl Acad Sci U S A. 2009 May 5;106(18):7345-50. doi: 10.1073/pnas.0808981106. Epub 2009 Apr 20. Proc Natl Acad Sci U S A. 2009. PMID: 19380739 Free PMC article.
-
Ensemble perception includes information from multiple spatial scales.
Sweeny TD, Bates A, Elias E. Sweeny TD, et al. Atten Percept Psychophys. 2021 Apr;83(3):982-997. doi: 10.3758/s13414-020-02109-9. Atten Percept Psychophys. 2021. PMID: 33083989
-
The anisotropic field of ensemble coding.
Pascucci D, Ruethemann N, Plomp G. Pascucci D, et al. Sci Rep. 2021 Apr 15;11(1):8212. doi: 10.1038/s41598-021-87620-1. Sci Rep. 2021. PMID: 33859281 Free PMC article.
-
Whitney D, Yamanashi Leib A. Whitney D, et al. Annu Rev Psychol. 2018 Jan 4;69:105-129. doi: 10.1146/annurev-psych-010416-044232. Epub 2017 Sep 11. Annu Rev Psychol. 2018. PMID: 28892638 Review.
-
Cui L, Liu Z. Cui L, et al. Atten Percept Psychophys. 2021 Apr;83(3):1290-1311. doi: 10.3758/s13414-020-02212-x. Epub 2021 Jan 3. Atten Percept Psychophys. 2021. PMID: 33389673 Review.
Cited by
-
Serial dependence: A matter of memory load.
Markov YA, Tiurina NA, Pascucci D. Markov YA, et al. Heliyon. 2024 Jul 2;10(13):e33977. doi: 10.1016/j.heliyon.2024.e33977. eCollection 2024 Jul 15. Heliyon. 2024. PMID: 39071578 Free PMC article.
-
Decoding time-resolved neural representations of orientation ensemble perception.
Yashiro R, Sawayama M, Amano K. Yashiro R, et al. Front Neurosci. 2024 Aug 1;18:1387393. doi: 10.3389/fnins.2024.1387393. eCollection 2024. Front Neurosci. 2024. PMID: 39148524 Free PMC article.