Dynamic convergence of autism disorder risk genes across neurodevelopment - PubMed
- ️Mon Jan 01 2024
[Preprint]. 2024 Aug 24:2024.08.23.609190.
doi: 10.1101/2024.08.23.609190.
Kayla Retallick-Townsley 1 2 , April Pruitt 3 , Elizabeth Davidson 4 , Yi Dai 4 , Sarah E Fitzpatrick 3 , Annabel Sen 1 , Sophie Cohen 1 , Olivia Livoti 1 , Suha Khan 4 , Grace Dossou 4 , Jen Cheung 1 , P J Michael Deans 1 , Zuoheng Wang 4 , Laura Huckins 1 3 2 , Ellen Hoffman 3 4 , Kristen Brennand 1 3 2
Affiliations
- PMID: 39229156
- PMCID: PMC11370590
- DOI: 10.1101/2024.08.23.609190
Dynamic convergence of autism disorder risk genes across neurodevelopment
Meilin Fernandez Garcia et al. bioRxiv. 2024.
Abstract
Over a hundred risk genes underlie risk for autism spectrum disorder (ASD) but the extent to which they converge on shared downstream targets to increase ASD risk is unknown. To test the hypothesis that cellular context impacts the nature of convergence, here we apply a pooled CRISPR approach to target 29 ASD loss-of-function genes in human induced pluripotent stem cell (hiPSC)-derived neural progenitor cells, glutamatergic neurons, and GABAergic neurons. Two distinct approaches (gene-level and network-level analyses) demonstrate that convergence is greatest in mature glutamatergic neurons. Convergent effects are dynamic, varying in strength, composition, and biological role between cell types, increasing with functional similarity of the ASD genes examined, and driven by cell-type-specific gene co-expression patterns. Stratification of ASD genes yield targeted drug predictions capable of reversing gene-specific convergent signatures in human cells and ASD-related behaviors in zebrafish. Altogether, convergent networks downstream of ASD risk genes represent novel points of individualized therapeutic intervention.
Keywords: CRISPR screen; GABAergic neurons; Human induced pluripotent stem cells; autism spectrum disorder; convergence; glutamatergic neurons; neural progenitor cells; precision medicine; psychiatric genomics.
Conflict of interest statement
CONFLICT OF INTEREST STATEMENT The authors declare no conflict of interest.
Figures
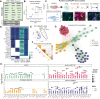
(A) List of targeted risk genes associated with autism spectrum disorder (ASD) and neurodevelopmental disorders (NDD). The boldest of the name indicates the strength of ASD correlation and genes are annotated if they are also rare variant targets of SCZ, BIP, and epilepsy (EPI) (B) MAGMA enrichments of targeted ASD genes across psychiatric GWAS. (C) Illustration of hiPSC derived cell-type specific scCRISPR-KO screen. Representative immunofluorescence for cell type markers for NPCs (DAPI/Nestin), mature iGLUTs (DAPI/MAP2/vGLUT), and mature iGABAs (DAPI/MAP2/GABA). (D) Transcriptomic impact of ASD/NDD gene KO across cell-type specific screens represented as the number of nominally significant (p<0.01) differentially expressed genes (DEGs). The degree of KO as indicated by gene expression (logFC) is not correlated with the proportion of significant DEGs (SI Fig. 8F,i–ii). (E) PCA of transcriptomic similarity between scramble controls across cell-types shows clustering based on cellular maturity, with mature iGLUTs and iGABAs and immature iGLUTs and NPCs clustering together respectively. (F) Pearson’s correlation matrix of log2FC across all KOs and cell-types demonstrated greater transcriptomic similarity within cell-types across distinct KOs compared to between cell-types and the same KO. (G) Cross cell-type correlation network diagram across KO perturbations demonstrates greater clustering between NPCs, immature iGLUTs, and surprisingly mature iGABAs, with KO signatures in mature GLUTs the most distinct and most strongly correlated. (H) GWAS disorder enrichments of KO DEGs across cell-types reveal unique KO-by-cell type common variant associations. (#nominal p-value<0.05, *FDR<0.05, **FDR<0.01, *FDR<0.001).
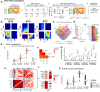
(A) Schematic explaining cross-KO cell-type specific convergence at the individual gene level using differential gene expression meta-analysis. (B) Convergence across 9 ASD gene KO perturbations is largely unique to a given neuronal cell type. Rank-rank hypergeometric (RRHO) test exploring correlation of convergent genes shared across 9 KO perturbations (RRHO score = −log10*direction of effect) between cell-types. The top right quadrant represents up-regulated genes (meta-analysis z-score >0) for the y-axis and x-axis cell-type. The bottom left quadrant represents down-regulated convergent genes (meta-analysis z-score <0) for the y-axis and x-axis cell-type. Significance is represented by color with red regions representing significantly convergent gene expression. (C) Venn diagram representing the absolute overlap (regardless of direction of dysregulation) of cell-type specific convergent genes shared across 9 KOs. (D) (i) Cell-type specific convergence across these 9 KOs is uniquely enriched for neuropsychiatric GWAS-risk associated genes by, ASD common variants only enriched in immature iGLUT convergence (direction of triangle represents beta direction and significance annotation FDRs from two-sided enrichment testing with MAGMA). (ii) ORA of cross-KO cell-type specific convergence and rare variant target genes related to SCZ, ASD, and ID. (#unadjusted p-value=<0.05, *FDR<=0.05, **FDR<0.01, ***FDR<0.001) (E) (i) Across all combinations of 2–5 genes across 9 KOs, the average magnitude of convergence was highest in iGLUTs. (ii) The magnitude of convergence between the same KOs tested in different cells was highly correlated – with the strongest relationship between NPCs, immature, and mature iGLUTs. (iii) Despite strong significant correlation between convergence degree, the top-most convergent KO combinations were unique to each cell-type. (iv) Gene-level convergence based on significant DEGs often corresponds with the cell-type specific strength of correlation between KO effects on the transcriptomic – with the strongest correlations in mature iGLUTs. (F) Expanding the convergence analysis across random subsets of 2–5 genes across 16,14, 21, and 21 KOs for NPCs, immature iGLUTs, mature iGLUTs, and mature iGABAs respectively, the average magnitude of convergence was still highest in iGLUTs.
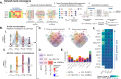
(A) Schematic explaining cross-KO cell-type specific convergence at the network level using Bayesian bi-clustering and undirected network reconstruction. (B) Testing the strength of network convergence across all possible combinations of 9 ASD/NDD KO perturbations, (i) the mean strength of network convergence is significantly different by cell-type, with the highest convergence present in immature iGLUTs. However, the same KO combinations test in one cell type may not resolve convergence in another cell type – indicating cell-type specificity. Each point represents the calculated convergence strength of one resolved network. Dots that represent the same combinations of KO perturbations, but tested in each cell type, are connected by a line. (ii) Testing the strength of network convergence across a subset of random combinations of 2–10 genes from the total 23 ASD/NDD KO perturbations, The mean strength of network convergence is significantly different by cell-type, with the highest convergence present in immature and mature iGLUTs, confirming the pattern seen with gene-level convergence (B) Venn diagrams of (i) the total number of networks resolved of the 502 combinations tested across 9 KOs further demonstrate that some KO combinations are only convergent in one cell-type – for example 6 are unique to NPCs. (ii) While overlap of the total number of unique node genes within those networks by cell-type demonstrate that even if the same combination of KOs resolve convergence, the node genes within their networks will be cell-type specific.(E) Enrichment of cell-type specific convergent node genes for rare variant targets revealed significant associations of immature iGLUTs with SCZ non-synonymous (NS) targets and nominal enrichments of mature iGLUTs and ASD LoF targets. (F) Although not significantly enriched, many node genes overlapped with GWAS common variant targets; heatmap representing the percent overlap of unique node genes for each cell-type that are targets of. Number in the center of each tile represent the absolute number of overlapping genes.
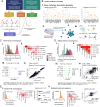
(A) Training random forest models for gene and network-level convergence - the proportion of cell-types was confirmed as balanced across the training sets and the testing sets and is representative the full data (SI Fig. 15). (B) Predictors included in the model include (i) semantic similarity scores based on gene ontology cellular component (C.C), biological process (B.P.) and molecular function (M.F) geneset membership, (ii) the scaled brain expression correlation of ASD genes in the adult dorsolateral prefrontal cortex (DLPFC), (iii) the cell-type, and (iv) the number of KOs represented in a convergent set, (C) (i) Distribution of gene-level convergence scores show cell-type specific distributions as described in Fig. 2 (n=20,240) – (ii) convergence is significantly correlated with functional similarity and brain co-expressions scores (FDR<0.001). (iii) Functional similarity, brain co-expression, cell-type, and the number of KOs assayed strongly predicted gene-level convergence (nTrees=500, 98% variance explained; mean of squared residuals<0.01) with cell-type as the most important predictor base on percent increase in mean error (%IncMSE) and the increase in node purity (IncNodePurity). (iv) Evaluation of the model in the testing set (n=6072) showed that predicted gene-level convergence by the model strongly correlated with the actual convergence (Pearson’s rho=0.99; Bonferroni p<0.001; root mean squared error (RMSE) =0.12); distribution of the number of nodes and error per tree in the random forest models reported in SI Fig. 15. (D) (i) Distribution of network-level convergence scores show cell-type specific distributions as described in Fig. 3 (n=3,204) – (ii) convergence is significantly correlated with CC and MF functional similarity (Holm’s adjP<0.001) nominally correlated with brain co-expressions scores (Holm’s adjP <0.08) (iii) Functional similarity, brain co-expression, cell-type, and the number of KOs assayed moderately predicted gene-level convergence (nTrees=500, 54% variance explained; mean of squared residuals=0.752) with the number of KOs/set and the C.C scores as the most important predictors. (iv) Evaluation of the model in the testing set (n=962) showed that predicted gene-level convergence by the model strongly correlated with the actual convergence (Pearson’s rho=0.714; Bonferroni p<0.001; RMSE=0.857). (E) Validation of predictor models in an independent scCRISPRa screen of 10 multifunctional SCZ common variant genes (eGenes) perturbed in iGLUTs demonstrated that network-level convergence model had greater predictive power. (i,ii) gene-level and network-level convergence distribution across 1,013 and 826 sets of 2–10 SCZ eGenes – convergence was significantly correlated with B.P (Holm’s adjP<0.05). M.F (Holm’s adjP<0.08), and BEC scores (Holm’s adjP <0.01) at the gene level and the number of KOs/set, B.EC and BP scores (Holm’s adjP<0.001) at the network level. While the gene-level convergence model has more predictive power within our data, it performed more poorly in external validation (RMSE=1.312, rho=0.07, Bonferroni P<0.02,) compared to the network-level model (RMSE=0.87, rho=0.364, Bonferroni P<0.001). (B.P score = semantic similarity of gene ontology: Biological Process membership between KO genes; C.C. score = semantic similarity of gene ontology: Cellular Component membership between KO genes; M.F score = semantic similarity of gene ontology: Molecular functions membership between KO genes; B.E.C = dorsolateral prefrontal cortex expression correlations between KO genes; nKOs = number of KO genes tested for convergence) (#nominal p-value<0.05, *adjP <0.05, **adjP <0.01, ***adjP <0.001).
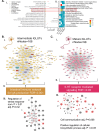
(A) Functional gene annotation (FUMA) identified sets of KOs with more specific shared function, beyond chromatin organization, and shared regulation by transcription factor and miRNAs. Network convergence across KOs based on functional annotation showed cell-type specificity and resolved networks with variable convergence and gene membership (i-ii). Convergent networks between targets of FOXO1 (PHF21A, SIN3A, and CHD2) were resolved in iGLUTs, but not NPCs and iGABAs. FOXO1 target convergent networks were unique in immature (B,i) and mature (C,i) iGLUTs. Central nodes in immature and mature iGLUTs included non-overlapping gene targets of psychiatric common and rare variants. The convergent network in immature iGLUTs was significantly enriched for immune networks related to IgA production (FDR<0.001) (B,ii) – network expansion using FunMap identified protein-protein connection between node genes that were nominally enriched for the regulation of stress response in immature iGLUTs (B,iii) (nom. P<0.01, adj. P=0.32). The convergent network in mature iGLUTs was significantly enriched 5-HT receptor mediated signaling (FDR<0.05) (C,ii) – network expansion identified a PPI network nominally enriched for cell communication (adj. P=0.066) and significantly enriched for positive regulation of cellular biosynthetic processes in mature iGLUTs (C,iii) (adj. P <=0.05).
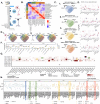
(A) Gene expression in human mature iGLUTs and iGABAs correlate with expression in the zebrafish brain. Cellular deconvolution of wild-type (wt) zebrafish brain expression based on an adult single-cell brain reference identified neurons as the largest proportion of cells in the fish brain. Gene expression in wt zebrafish brain significantly positively correlate with gene expression of human-induced mature iGLUTs (rho=0.39, Holm’s adj.P<0.001) and iGABAs (rho=0.39, Holm’s adj.P <0.001) (SI Fig. 9). (B) ASD/NDD risk genes uniquely cluster based on wake/startle behavioral responses in zebrafish KOs. Based on correlations between 24 phenotypic measurements related to sleep, wake, and startle response in zebrafish KOs, ASD/NDD risk genes clustered into four behavioral sets. Set 1: MBD5, NRXN1, KDM5B; Set 2: CHD2, SMARCC2, PHF12, SKI; Set 3: KMT5B, KMT2C, KDM6B; Set 4: ARID1B, ASH1L, CHD8, PHF21A, WAC. (C) Gene-level convergence within these sets is largely non-overlapping between mature iGLUTs and iGABAs and (D) show unique enrichments for common psychiatric risk gene targets. (E) Within each cell-type, behavioral sets have both shared and unique convergent genes (i-ii). (F) In both iGABAs and iGLUTs, all four behavioral sets were enriched for FMRP targets. Gene targets of neurodevelopmental rare variants were only significantly enriched for convergent signature in mature iGLUTs – with behavioral set 4 uniquely significantly enriched for secondary targets of ASD loss-of-function variant and set 3 uniquely enriched for primary targets of SCZ non-synonymous variants (iii). (F). Prediction of drugs “reversers” from the LiNCs database targeting these convergent signals in mature neurons identify shared and unique drugs enriched across the sets (i.e., antipsychotic perphenazine in iGLUTs) and some specific to unique convergence (i.e., valsartan only in in Set 3 iGABAs, and sirolimus in Set 3 iGLUTs). (G) Top predicted drugs show opposing effects on zebrafish behavior compared to individual ASD KOs – these signatures do not always correspond with their predicted direction of effect on transcriptomic convergence. (#nominal p-value<0.05, *FDR<0.05, **FDR<0.01, *FDR<0.001)
Similar articles
-
Breen MS, Browne A, Hoffman GE, Stathopoulos S, Brennand K, Buxbaum JD, Drapeau E. Breen MS, et al. Mol Autism. 2020 Jun 19;11(1):53. doi: 10.1186/s13229-020-00355-0. Mol Autism. 2020. PMID: 32560742 Free PMC article.
-
Lewis EMA, Meganathan K, Baldridge D, Gontarz P, Zhang B, Bonni A, Constantino JN, Kroll KL. Lewis EMA, et al. Mol Autism. 2019 Dec 30;10:51. doi: 10.1186/s13229-019-0306-0. eCollection 2019. Mol Autism. 2019. PMID: 31893020 Free PMC article.
-
Prem S, Dev B, Peng C, Mehta M, Alibutud R, Connacher RJ, St Thomas M, Zhou X, Matteson P, Xing J, Millonig JH, DiCicco-Bloom E. Prem S, et al. Elife. 2024 Mar 25;13:e82809. doi: 10.7554/eLife.82809. Elife. 2024. PMID: 38525876 Free PMC article.
-
Human Inducible Pluripotent Stem Cells and Autism Spectrum Disorder: Emerging Technologies.
Nestor MW, Phillips AW, Artimovich E, Nestor JE, Hussman JP, Blatt GJ. Nestor MW, et al. Autism Res. 2016 May;9(5):513-35. doi: 10.1002/aur.1570. Epub 2015 Oct 1. Autism Res. 2016. PMID: 26426199 Review.
-
Convergence of spectrums: neuronal gene network states in autism spectrum disorder.
Sullivan JM, De Rubeis S, Schaefer A. Sullivan JM, et al. Curr Opin Neurobiol. 2019 Dec;59:102-111. doi: 10.1016/j.conb.2019.04.011. Epub 2019 Jun 18. Curr Opin Neurobiol. 2019. PMID: 31220745 Free PMC article. Review.
References
-
- Bicks L. K. et al. Functional neurogenomics in autism spectrum disorders: A decade of progress. Curr Opin Neurobiol 86, 102858, (2024). - PubMed
Publication types
Grants and funding
LinkOut - more resources
Full Text Sources