Integrated Brain Connectivity Analysis with fMRI, DTI, and sMRI Powered by Interpretable Graph Neural Networks - PubMed
- ️Mon Jan 01 2024
Integrated Brain Connectivity Analysis with fMRI, DTI, and sMRI Powered by Interpretable Graph Neural Networks
Gang Qu et al. ArXiv. 2024.
Abstract
Multimodal neuroimaging modeling has become a widely used approach but confronts considerable challenges due to heterogeneity, which encompasses variability in data types, scales, and formats across modalities. This variability necessitates the deployment of advanced computational methods to integrate and interpret these diverse datasets within a cohesive analytical framework. In our research, we amalgamate functional magnetic resonance imaging (fMRI), diffusion tensor imaging (DTI), and structural MRI (sMRI) into a cohesive framework. This integration capitalizes on the unique strengths of each modality and their inherent interconnections, aiming for a comprehensive understanding of the brain's connectivity and anatomical characteristics. Utilizing the Glasser atlas for parcellation, we integrate imaging-derived features from various modalities-functional connectivity from fMRI, structural connectivity from DTI, and anatomical features from sMRI-within consistent regions. Our approach incorporates a masking strategy to differentially weight neural connections, thereby facilitating a holistic amalgamation of multimodal imaging data. This technique enhances interpretability at connectivity level, transcending traditional analyses centered on singular regional attributes. The model is applied to the Human Connectome Project's Development study to elucidate the associations between multimodal imaging and cognitive functions throughout youth. The analysis demonstrates improved predictive accuracy and uncovers crucial anatomical features and essential neural connections, deepening our understanding of brain structure and function. This study not only advances multi-modal neuroimaging analytics by offering a novel method for the integrated analysis of diverse imaging modalities but also improves the understanding of intricate relationship between the brain's structural and functional networks and cognitive development.
Keywords: Brain Connectivity; Cognitive Development; Cognitive Neuroscience; Diffusion Tensor Imaging (DTI); Functional MRI (fMRI); Graph deep learning; Human Connectome Project; Multimodal Neuroimaging Integration; Structural MRI (sMRI).
Conflict of interest statement
Financial Dissclosures Section All authors declare that they have no conflicts of interest.
Figures
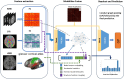
Depiction of the Proposed Framework: Functional connectivity (FC) and structural connectivity (SC) obtained from fMRI and DTI, respectively, are amalgamated at the nodal level and subsequently fed into the MaskGNN for predictive analysis. In the latent space, embeddings of nodal features are integrated with anatomical statistics (AS) from sMRI, alongside a computation of structure-functional coupling using the FC and SC matrices. The aggregated features are then subjected to MaskGNN embedding, graph pooling, and readout processes. After post-training, visualization of the uniform mask across MaskGNN layers is achieved, and a posthoc approach elucidates the contribution of AS.
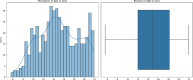
The age distribution of selected subjects.
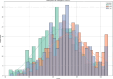
The distribution of intelligence metrics.
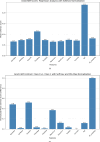
The use of Grad-CAM and Grad-RAM scores for model explainability: (a) Grad-RAM scores for simultaneous prediction of CCC and FCC; (b) Discrimination of groups using Grad-CAM scores across distinct TCC levels
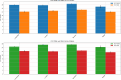
A comparative analysis of predictive performance showing the individual and combined effects of the manifold regularization term (Lmanifold) and mask penalty (Lmask) on the proposed model, with a baseline scenario for reference. All comparisons are supported by pair-wise t-tests, with p-values less than 1e − 3 relative to the fully proposed model, highlighting significant differences.
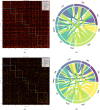
The model interpretability through learned masks with 0.52 as threshold: (a) mask derived from the simultaneous prediction task for CCC and FCC; (b) Chord diagram from the simultaneous CCC and FCC prediction task, showing inter-network connections among brain functional networks, excluding intra-network links;(c) mask generated for the classification task across distinct TCC levels;(d) Chord diagram from the classification task across distinct TCC levels, showing inter-network connections among brain functional networks, excluding intra-network links. Vis-Visual, SM-Somatomotor, CO-Cingulo-Opercular, DA-Dorsal-Attention, Lang-Language, FP-Frontoparietal , Aud-Auditory, Def-Default, Oth-Others.
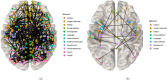
The visualization of Identified Brain Connectivities: Enhanced clarity is achieved by setting the visualization threshold to 0.53. (a) Connectivity patterns identified via the mask generated from the prediction task for CCC and FCC scores. (b) Connectivity patterns identified via the mask generated from the classification task for groups with high and low TCC scores.
Similar articles
-
Lee TW, Xue SW. Lee TW, et al. Neurosci Lett. 2017 Jun 9;651:123-127. doi: 10.1016/j.neulet.2017.05.005. Epub 2017 May 4. Neurosci Lett. 2017. PMID: 28479103
-
Sui J, Huster R, Yu Q, Segall JM, Calhoun VD. Sui J, et al. Neuroimage. 2014 Nov 15;102 Pt 1:11-23. doi: 10.1016/j.neuroimage.2013.09.044. Epub 2013 Sep 29. Neuroimage. 2014. PMID: 24084066 Free PMC article. Review.
-
Abrol A, Fu Z, Du Y, Calhoun VD. Abrol A, et al. Annu Int Conf IEEE Eng Med Biol Soc. 2019 Jul;2019:4409-4413. doi: 10.1109/EMBC.2019.8856500. Annu Int Conf IEEE Eng Med Biol Soc. 2019. PMID: 31946844
-
Zou R, Li L, Zhang L, Huang G, Liang Z, Xiao L, Zhang Z. Zou R, et al. Front Mol Neurosci. 2022 Mar 15;15:844146. doi: 10.3389/fnmol.2022.844146. eCollection 2022. Front Mol Neurosci. 2022. PMID: 35370547 Free PMC article.
-
Fusing DTI and fMRI data: a survey of methods and applications.
Zhu D, Zhang T, Jiang X, Hu X, Chen H, Yang N, Lv J, Han J, Guo L, Liu T. Zhu D, et al. Neuroimage. 2014 Nov 15;102 Pt 1:184-91. doi: 10.1016/j.neuroimage.2013.09.071. Epub 2013 Oct 5. Neuroimage. 2014. PMID: 24103849 Free PMC article. Review.
References
-
- Chattopadhay A., et al., 2018. Grad-cam++: Generalized gradient-based visual explanations for deep convolutional networks, in: 2018 IEEE winter conference on applications of computer vision (WACV), IEEE. pp. 839–847.
-
- Chok N.S., 2010. Pearson’s versus Spearman’s and Kendall’s correlation coefficients for continuous data. Ph.D. thesis. University of Pittsburgh.
-
- Dahnke R., et al., 2013. Cortical thickness and central surface estimation. Neuroimage 65, 336–348. - PubMed